Damage quantification method for aircraft structures based on multi-task CNN-LSTM and transfer learning
IEEE Sensors Journal(2024)
摘要
Damage quantification based on Lamb waves is one of the research hotspots in the field of aerospace structural health monitoring (SHM). Deep learning (DL) is an efficient method to identify damage-related features from Lamb waves complex responses. In this paper, a multi-task convolutional neural networks and long-term and short-term memory networks (CNN-LSTM) damage quantification method combining transfer learning is proposed, which directly uses the Lamb waves signal in the original discrete time domain to predict the size and location of damage. The one-dimensional convolutional neural network (1D-CNN) is used to achieve damage size prediction, which can not only learn the corresponding features but also avoid wasting training resources. For damage location prediction, a multi-task CNN-LSTM network architecture is established. Two parallel branches can output the coordinates of damage in x and y directions at the same time, to locate the damage at any location within the structure. To prove the reliability and generalization ability of the method, three data sets are collected through experiments. The three data sets are derived from two aluminum plates and one composite laminate. The model trained on the first aluminum plate is defined as the pre-training model, its structure and weight are extracted, and then the transfer learning method is used to realize the structural damage location identification of aluminum plate-aluminum plate and aluminum plate-composite laminate., which is of certain value for the research and application of transfer learning theory in damage quantification.
更多查看译文
关键词
Structural Health Monitoring,Damage Quantification,Lamb Waves,Multi-task CNN-LSTM,Transfer Learning
AI 理解论文
溯源树
样例
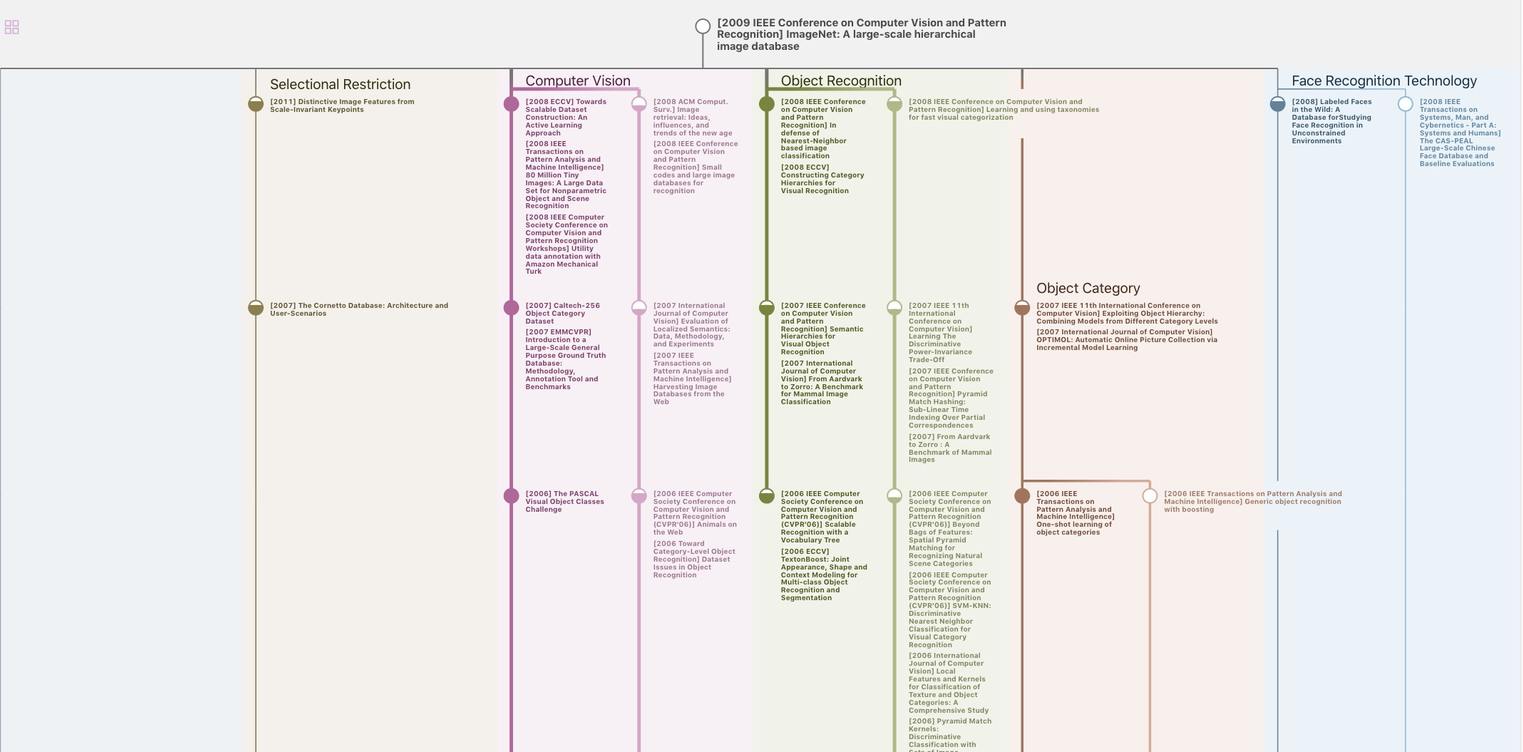
生成溯源树,研究论文发展脉络
Chat Paper
正在生成论文摘要