Recommendation-Enabled Edge Caching and D2D Offloading Via Incentive-Driven Deep Reinforcement Learning
IEEE Transactions on Services Computing(2024)
摘要
This paper proposes a novel architecture of Recommendation-Enabled Edge Caching and Device-to-Device (D2D) Offloading via Incentive-driven Deep Reinforcement Learning (DRL), which can not only solve the problem of inaccurate recommendation caused by sparse rating matrix, but also encourage users to participate in D2D offloading through an effective incentive mechanism. Specifically, we define Pseudo Markov Decision Process (PMDP) for the first time, which enables the conversion of the non-sequential process (
e.g. rating prediction
) into a sequential one, making it suitable for DRL. Then, combining Supervised Learning (SL) and DRL, a Supervised DRL for Collaborative Filtering (CF) algorithm, named SDRLCF, is proposed to predict missing ratings. After that, from the perspective of Content Service Center (CSC), the incentive-driven recommendation-enabled edge caching and D2D offloading can be formulated as a Non-Linear Integer Programming (NLIP) problem, which belongs to NP-hard, and is difficult to obtain the optimal solution in polynomial time. To address this issue, a DRL based Edge Caching and Recommendation algorithm, named DRLECR, is proposed to minimize the cost of CSC. Finally, combining with economic theory, a Reverse Auction based Payment Determination algorithm under Vickrey-Clarke-Groves (VCG) scheme, named RAPD, is proposed, which can stimulate users to participate in edge caching and D2D offloading while guaranteeing the individual rationality and truthfulness of participants. Extensive experiment results on both realistic and synthetic datasets demonstrate that the proposed algorithms outperform other baseline methods under different scenarios.
更多查看译文
关键词
Device-to-Device,edge caching,incentive mechanism,recommendation,reinforcement learning
AI 理解论文
溯源树
样例
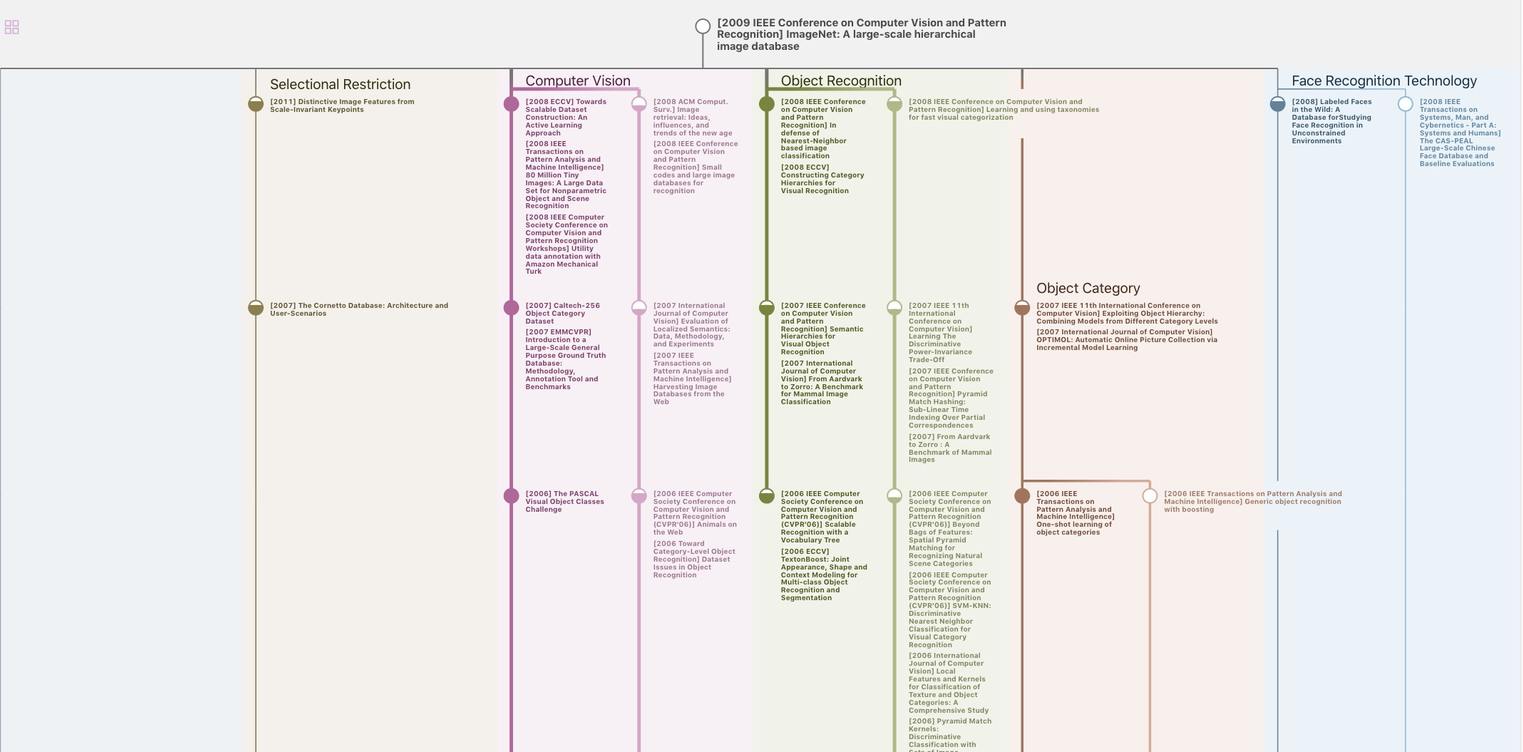
生成溯源树,研究论文发展脉络
Chat Paper
正在生成论文摘要