Self-Matching Chirplet Extraction Transform: A Novel Tool for Dense Multicomponent Signals Analysis and Machinery Fault Diagnosis
IEEE Sensors Journal(2024)
摘要
This study introduces a novel time-frequency (TF) analysis methodology, designated as the self-matching chirplet extraction transform (SMCET). This innovative technique is specifically crafted for the analysis of non-stationary signals characterized by dense multicomponents, aimed at achieving an accurate time-frequency representation (TFR). SMCET extends chirplet transform (CT) by employing parameterized phase kernels with higher-order expansions, which accurately fits the instantaneous frequencies of nonlinear variations, thereby achieving precise matching between chirprate and varying frequency. This technique effectively resolves the issue of TF resolution ambiguity, a challenge encountered by CT-improved algorithms, when processing dense components. The optimization strategy for self-matching parameter significantly enhances both precision of matching and computational efficiency by narrowing the traversal range of chirprate angle. By combining instantaneous frequency estimate with the matching extraction operator, a higher quality TFR can be obtained, even in the case of noise interference. The efficacy of SMCET is convincingly demonstrated via numerical and experimental analyses, focusing on vibration signals of bearing and planetary gearbox. The analytical outcomes reveal that SMCET exhibits superior capabilities in characterizing nonlinear multicomponent signals, including those with dense components. This underlines its significant potential for accurate fault diagnosis on rotary machinery.
更多查看译文
关键词
Chirplet transform,Dense multicomponents,Time-varying features,Instantaneous frequency,Fault diagnosis
AI 理解论文
溯源树
样例
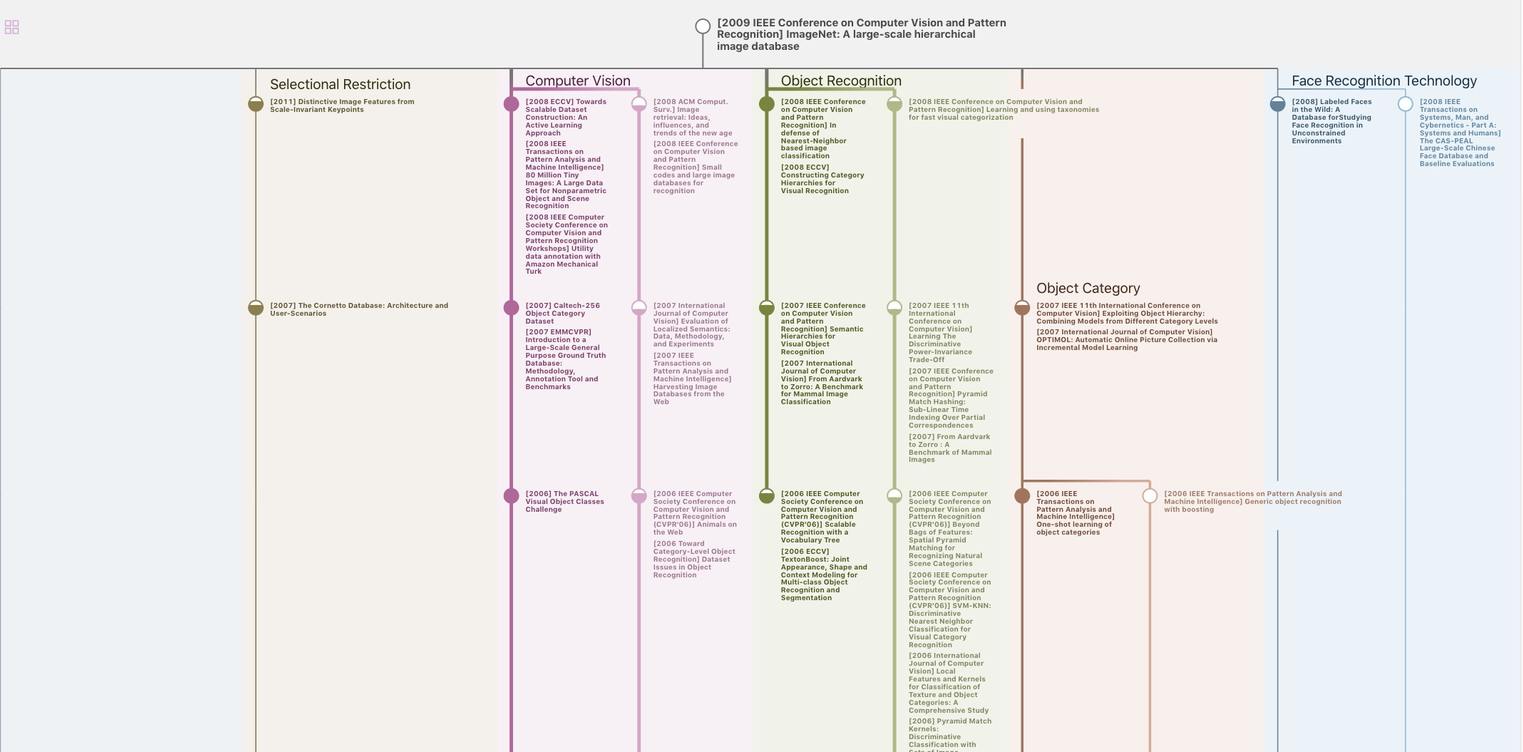
生成溯源树,研究论文发展脉络
Chat Paper
正在生成论文摘要