SEFRNet: A SAR Ship Target Detection Network with Effective Feature Representation
IEEE Sensors Journal(2024)
摘要
SAR ship detection plays a crucial role in various fields, such as maritime transportation supervision and crisis rescue. However, in most SAR ship images, the targets occupy a small number of pixels in the image, making it difficult to distinguish the targets from the background. This challenge makes it hard for lightweight deep learning-based object detection algorithms to obtain effective feature information. To address these issues, a SAR ship detection network with effective feature representation is proposed. Initially, an efficient feature extraction network (EFENet) using a hybrid architecture of convolutional neural network and Vision Transformer is proposed. The EFENet learns both local and global information within images, while embedding a bidirectional attention mechanism (BAM) module for precise target localization and enhanced capture of small target information. Subsequently, the multi-branch spatial pyramid pooling module (MBSPP) is introduced to expand the receptive field of feature maps, addressing the issue of the target position information loss in high-level features. Lastly, a cross-scale feature fusion network (CSFNet) is designed to aggregate feature maps of different scales, resulting in feature maps containing rich semantic and positional information. Experimental results demonstrate the effectiveness of this approach in achieving high-quality detection results on both the SSDD dataset and the HRSID dataset.
更多查看译文
关键词
Synthetic aperture radar (SAR),ship detection,feature representation,bidirectional attention mechanism,feature fusion
AI 理解论文
溯源树
样例
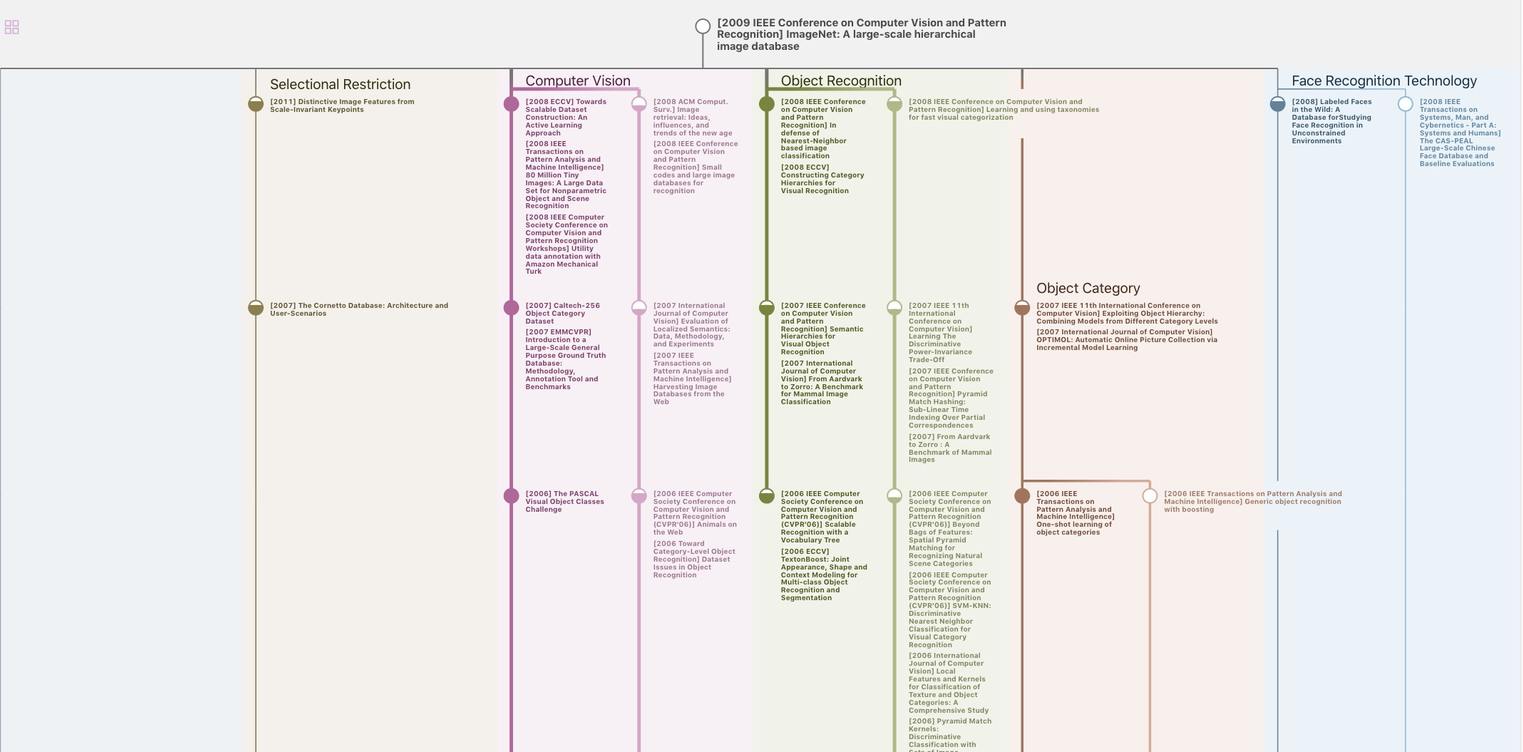
生成溯源树,研究论文发展脉络
Chat Paper
正在生成论文摘要