MIE-Net: Motion Information Enhancement Network for Fine-Grained Action Recognition Using RGB Sensors
IEEE Sensors Journal(2024)
摘要
In recent years, action recognition has received widespread attention, which classifies actions by extracting features from kinds of sensor data. However, with the growing difficulty of identifying fine-grained actions, certain methods cannot learn sufficient motion and temporal information. Therefore, an effective information enhancement method is required to reason motion clues in video sequences. This paper proposes an end-to-end video action recognition framework called the Motion Information Enhancement Network (MIE-Net), which consists of two innovative components. The first component, the Adaptive Fusion Module (AFM), selectively extracts the relationships between original and motion-enhanced features to enhance the interaction among different feature information. The second component, a Double Pooling Temporal Attention Module (DPTAM), implements temporal modeling to enhance subtle information during feature extraction. Finally, a Standing Long Jump Dataset (SLJD) containing over 1000 videos from 116 participants is collected by sensor camera, which differs from existing datasets in terms of strong background unbiasedness, to evaluate the effectiveness of our model robustly. Experimental results on SLJD, Something-Something v2 and Diving48 datasets demonstrate that the proposed MIE-Net outperforms most state-of-the-art methods. Our code is released at https://github.com/li-stu-998/MIE-Net.
更多查看译文
关键词
Video action recognition,feature fusion,temporal modeling,motion information enhancement
AI 理解论文
溯源树
样例
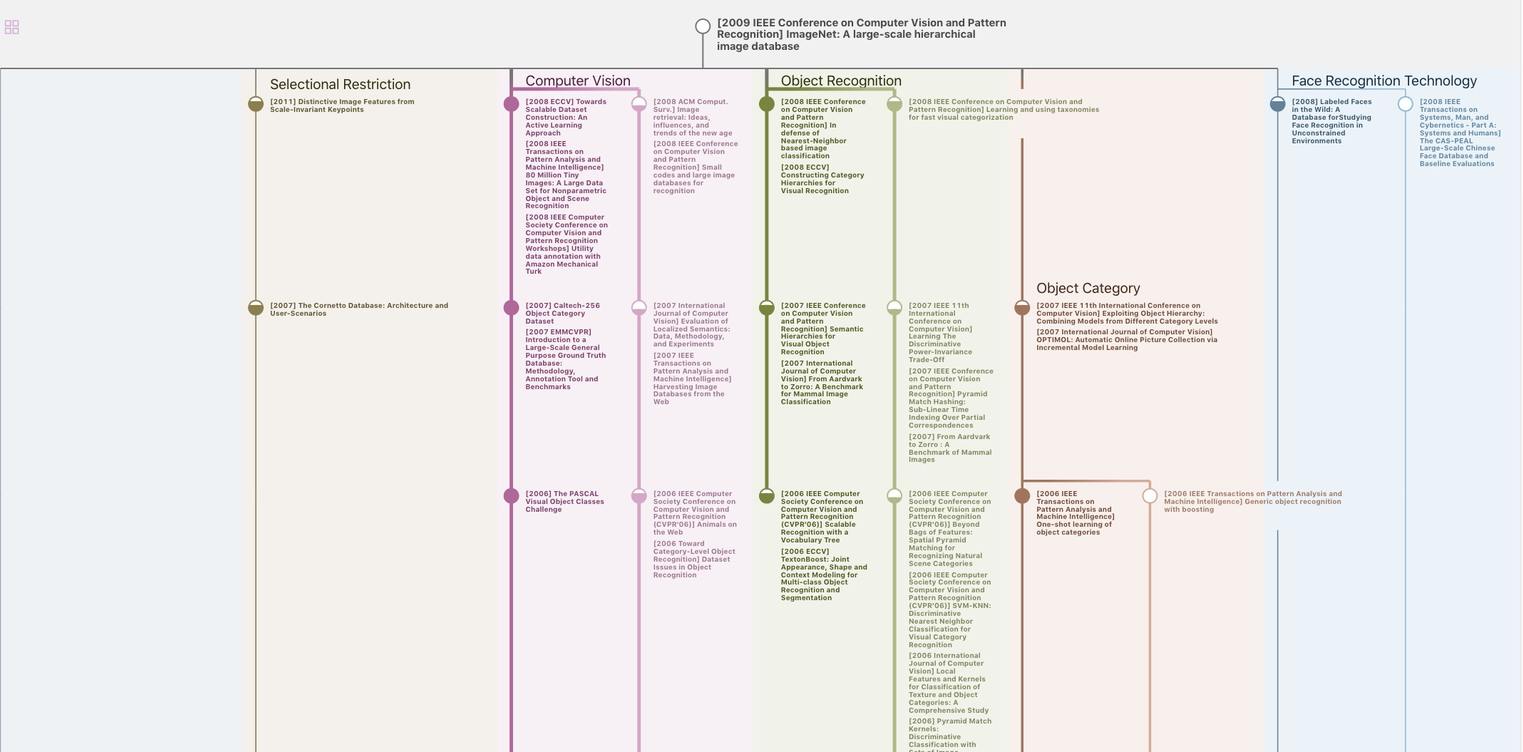
生成溯源树,研究论文发展脉络
Chat Paper
正在生成论文摘要