A Privacy-preserving Aggregation Scheme with Continuous Authentication for Federated Learning in VANETs
IEEE Transactions on Vehicular Technology(2024)
摘要
Federated Learning (FL) allows the collaborative training of a global model in Vehicular Ad-hoc Networks (VANETs): data is maintained on the owner's device and the local gradient updates to the model are aggregated through a secure protocol. However, despite its many advantages, FL is vulnerable to various attacks from malicious clients. Current defenses have several weaknesses. For example, the server may still select malicious clients for aggregation even after they have been identified in previous rounds. They haven't considered the continual monitoring of clients to resist their possible defection or collusion that occurs throughout the FL training process. In response to the weaknesses, we describe a new aggregation model with continuous authentication suits for VANETs. The authentication implementation relies on a non-interactive zero-knowledge proof which preserves privacy. We also minimize the computation and communication overhead by designing a two-phase aggregation scheme, while introducing the Edge Devices (EDs) to assist the FL procedure. Finally, we introduce an application of such a model for VANETs. We describe the prototype implementation and experimentally confirm that the aggregation overhead of the client grows linearly and achieves training speed up over the prior work.
更多查看译文
关键词
Aggregation,Privacy-preserving,Authentication,VANETs
AI 理解论文
溯源树
样例
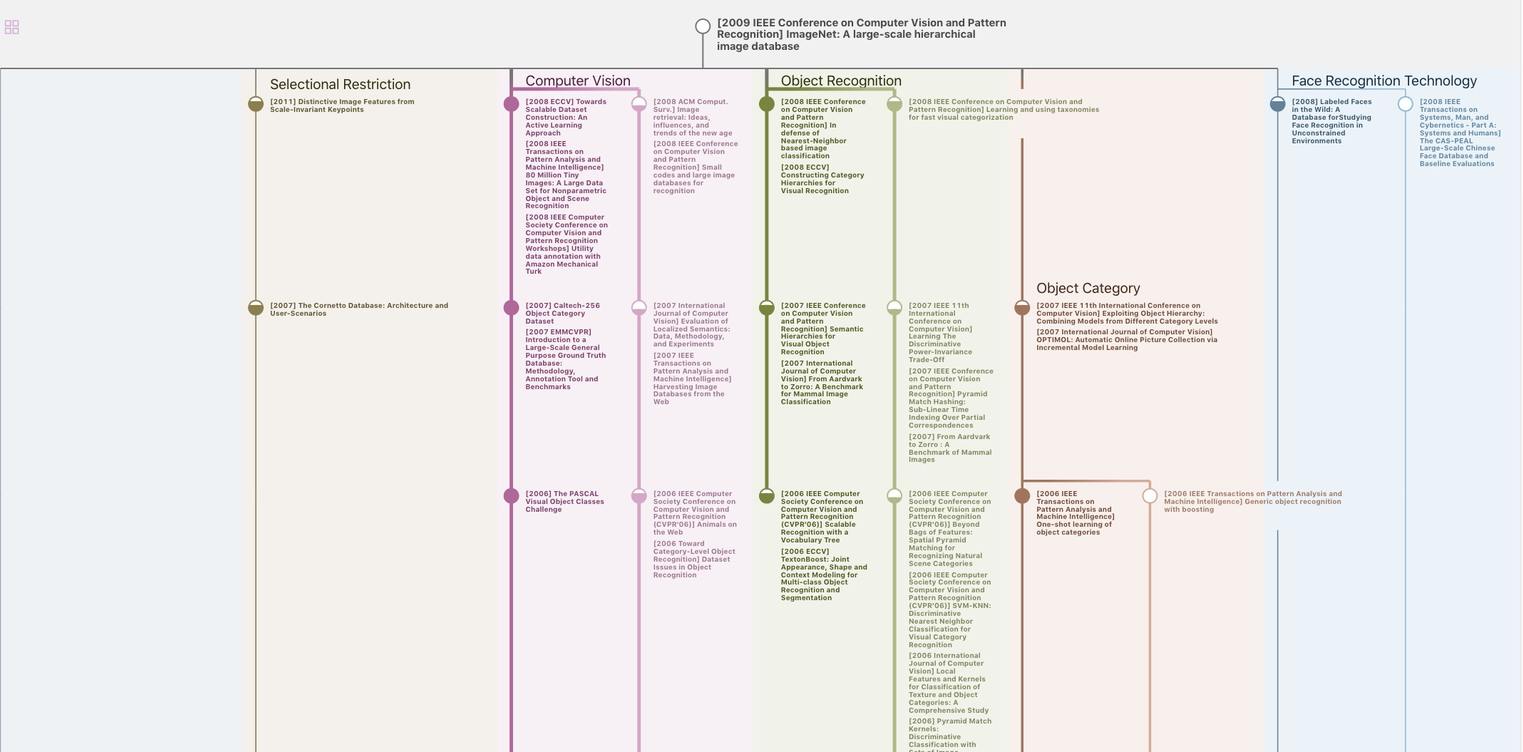
生成溯源树,研究论文发展脉络
Chat Paper
正在生成论文摘要