Examination of saturated hydraulic conductivity using grading curve functions
semanticscholar(2021)
Abstract
In a previous research program 74 artificial soil mixtures of natural fluvial soils were prepared in 4 series of measurements for falling head permeability testing, differing in d10. The conclusions drawn from the original investigations were as follows: the k showed a decreasing tendency with the increase of the uniformity index U for each series of measurements and increasing tendency with d10. In this research these are used (i) to test the value of the grading entropy parameters in case of nonprecise grading curve measurement with missing fines, (ii) to correlate the usual grading curve parameters like d10, d30, d50 and d60 or their ratios and the grading entropy parameters, (iii) to validate some existing permeability – grading curve equations and to elaborate some new permeability – grading curve relationships partly with entropy parameters partly with the usual parameters. For these aims, series I to IV have been started to be reevaluated, some specific surface formulae were derived. Some additional, literature data were also considered. The very first results are presented here. According to the results, the fine fractions significantly influenced the value of the entropy parameters. The base entropy So showed strong relationship with and parameters like d10, d30, d50 and d60. The entropy increment ΔS showed a monotonic increasing relationship with U. The specific surface parameter (containing density info) showed the best relationship with k out of the d-type parameters like d10 or harmonic mean dh. The original conclusions were reformulated in terms of S0 and and ΔS. It was also concluded that those parameters that are based on all measured data are more precise than the single diameter values in the k-regressions. The specific surface parameter is the best in this repect probably since containing density information, too.
MoreTranslated text
PDF
AI Read Science
AI Summary
AI Summary is the key point extracted automatically understanding the full text of the paper, including the background, methods, results, conclusions, icons and other key content, so that you can get the outline of the paper at a glance.
Example
Background
Key content
Introduction
Methods
Results
Related work
Fund
Key content
- Pretraining has recently greatly promoted the development of natural language processing (NLP)
- We show that M6 outperforms the baselines in multimodal downstream tasks, and the large M6 with 10 parameters can reach a better performance
- We propose a method called M6 that is able to process information of multiple modalities and perform both single-modal and cross-modal understanding and generation
- The model is scaled to large model with 10 billion parameters with sophisticated deployment, and the 10 -parameter M6-large is the largest pretrained model in Chinese
- Experimental results show that our proposed M6 outperforms the baseline in a number of downstream tasks concerning both single modality and multiple modalities We will continue the pretraining of extremely large models by increasing data to explore the limit of its performance
Try using models to generate summary,it takes about 60s
Must-Reading Tree
Example
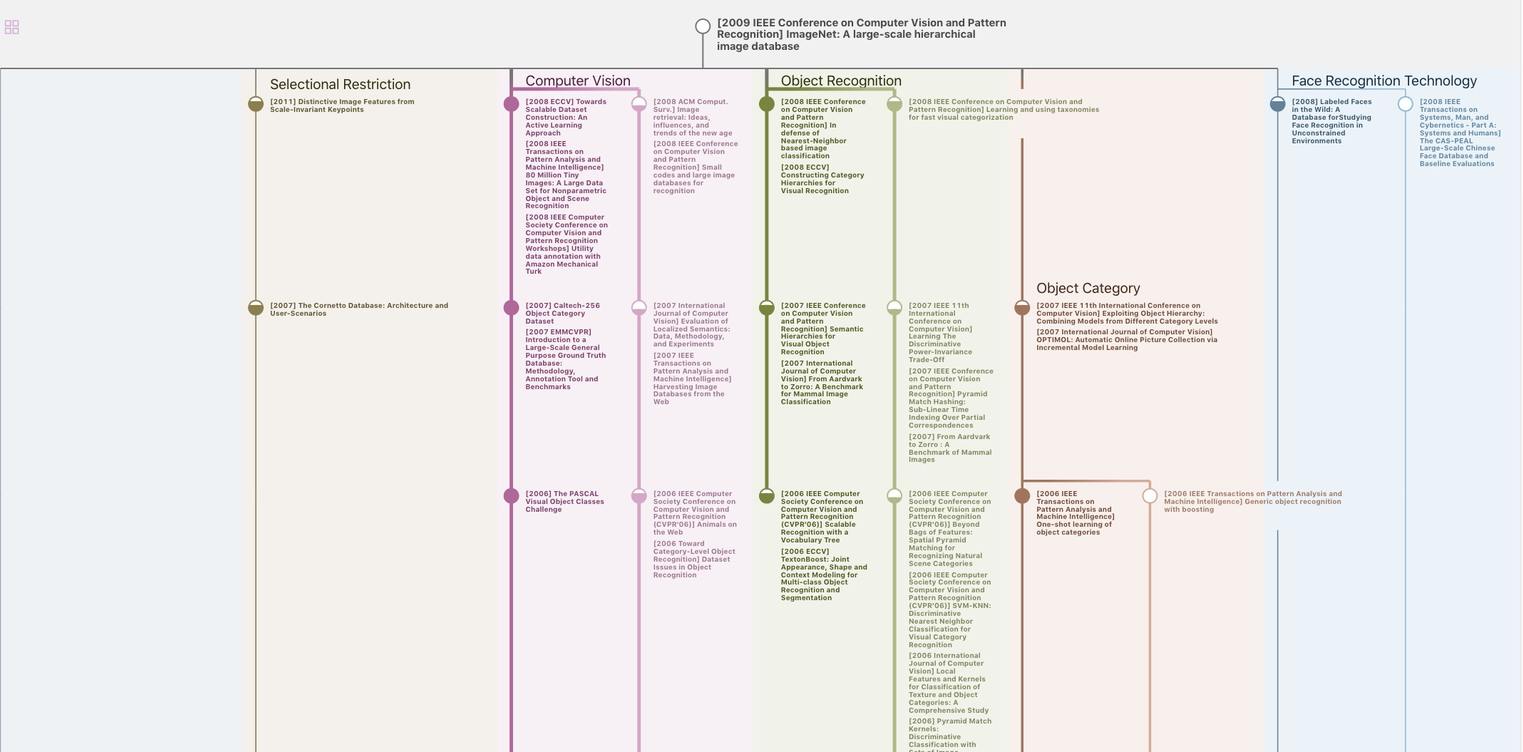
Generate MRT to find the research sequence of this paper
Related Papers
Data Disclaimer
The page data are from open Internet sources, cooperative publishers and automatic analysis results through AI technology. We do not make any commitments and guarantees for the validity, accuracy, correctness, reliability, completeness and timeliness of the page data. If you have any questions, please contact us by email: report@aminer.cn
Chat Paper
去 AI 文献库 对话