Novel Long Short-Term Memory Model Based on the Attention Mechanism for the Leakage Detection of Water Supply Processes
IEEE TRANSACTIONS ON SYSTEMS MAN CYBERNETICS-SYSTEMS(2024)
摘要
With the development of urban water supply systems, the leakage detection of water supply pipe networks is of great significance for the safe operation of urban water supply systems. In practice, due to the short of the important data, traditional detection models often fail to achieve good detection results. Therefore, this article proposes a novel pipeline attention integrating the long short-term memory (LSTM) to detect the leakage. The density-based spatial clustering of applications with noise (DBSCAN) method divides the water supply network into several regions according to the leakage characteristics of pipelines. Then, the LSTM extracts dynamic time-varying hydraulic features of pipelines, and the pipeline attention extracts the dynamically changing features between pipelines. Finally, the Attention-LSTM model is used to detect the leak region of urban water supply systems. Compared with multilayer perceptron classifier, K neighbors classifier, decision tree classifier, support vector machine classifier, multiscale fully convolutional network, one-dimensional multichannel convolution neural network, and variational autoencoder, the F1-Score of the Attention-LSTM is greatly improved, which are 116%, 120%, 254%, 180%, 21%, 26%, and 27%, respectively. Therefore, the proposed model can accurately detect the leakage of water supply network and effectively reduce the waste of resources.
更多查看译文
关键词
Attention mechanism,density-based spatial clus- tering of applications with noise (DBSCAN),leakage detection,long short-term memory (LSTM),water supply systems
AI 理解论文
溯源树
样例
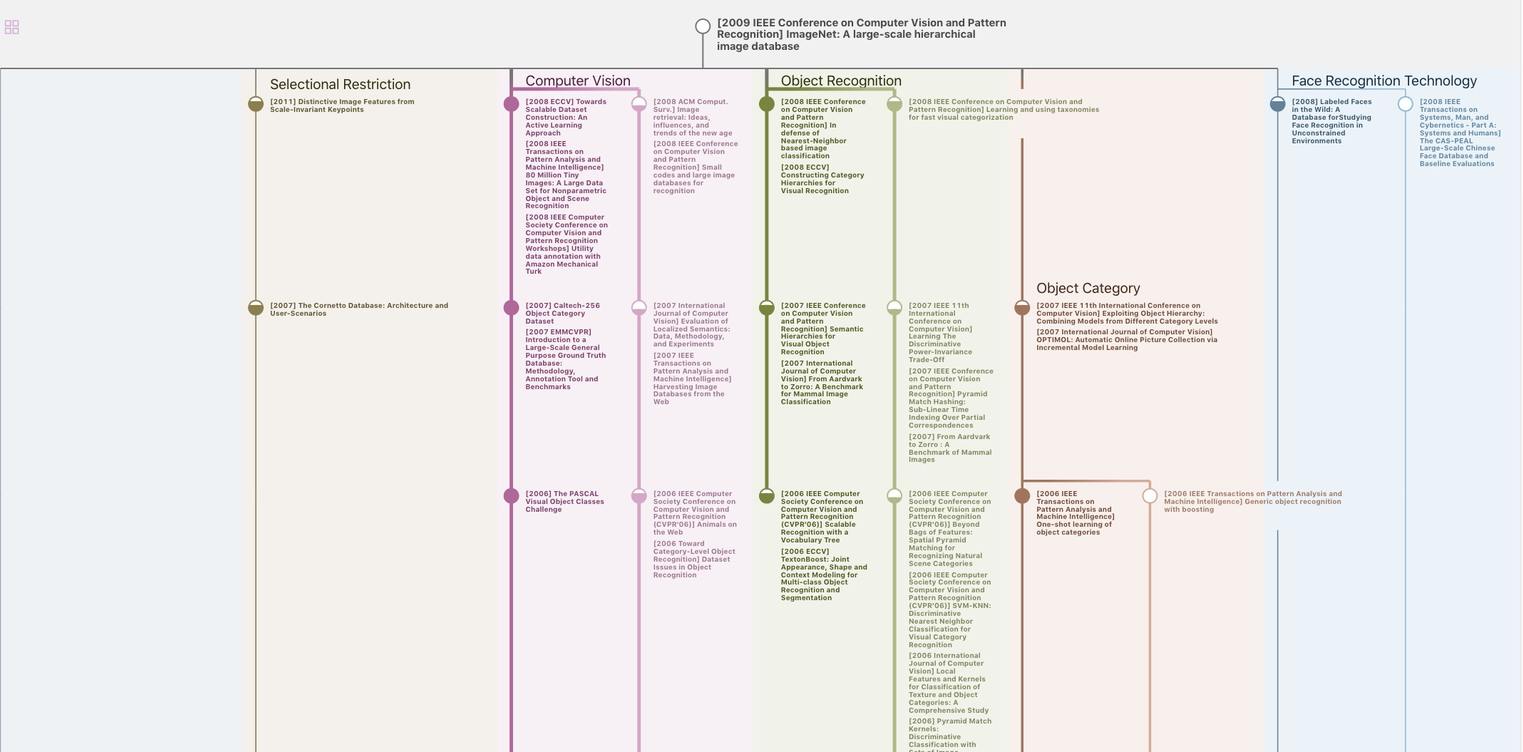
生成溯源树,研究论文发展脉络
Chat Paper
正在生成论文摘要