Assessment of Stacked LSTM, Bidirectional LSTM, ConvLSTM2D, and Auto Encoders LSTM Time Series Regression Analysis at ADITYA-U Tokamak
IEEE TRANSACTIONS ON PLASMA SCIENCE(2024)
摘要
Disruptions are events in which large fractions of the plasma thermal energy are lost due to the uncontrolled growth of some large-scale plasma instability. Major disruptions lead to the sudden termination of plasma current in a very short timescale. A data-driven methodology employing time series analysis using relevant plasma parameters for prediction of the disruption event before sufficient alarming time is useful due to the absence of first-principle physics methods. ADITYA ( R 0 = 75 cm and a = 25 cm), an ohmically heated circular limiter tokamak, has been upgraded to a tokamak named the ADITYA Upgrade (ADITYA-U) with an open diverter configuration. Based on previous ADITYA similar to 8000 discharges, time series regression analysis has been developed using various long short-term memory (LSTM)-based deep learning methods such as Stacked, Bidirectional, ConvLSTM2D, and Auto Encoder LSTM. The results are assessed with ADITYA data and benchmarked to optimize results for final model deployment. Root-mean-square error (RMSE) is used to measure error during training and testing the neural network prediction. The anomalies in the signal prediction are closely analyzed in each LSTM method. Furthermore, the data sequence is modified for time series data to get higher optimization in training time. The analytical data using the model achieve sufficient anticipation time to predict the plasma disruption event as per the desired requirement. ConvLSTM2D gives promising results among other methods, but Auto Encoder LSTM provides higher accuracy for unseen data in real-time prediction with the lowest RMSE of 1.79. The final model will be used for inference and deployment for accurate prediction of the disruption event at ADITYA-U tokamak.
更多查看译文
关键词
Plasmas,Data models,Predictive models,Training,Tokamak devices,Discharges (electric),Neural networks,ADITYA-U tokamak,deep learning,disruption,long short-term memory (LSTM)
AI 理解论文
溯源树
样例
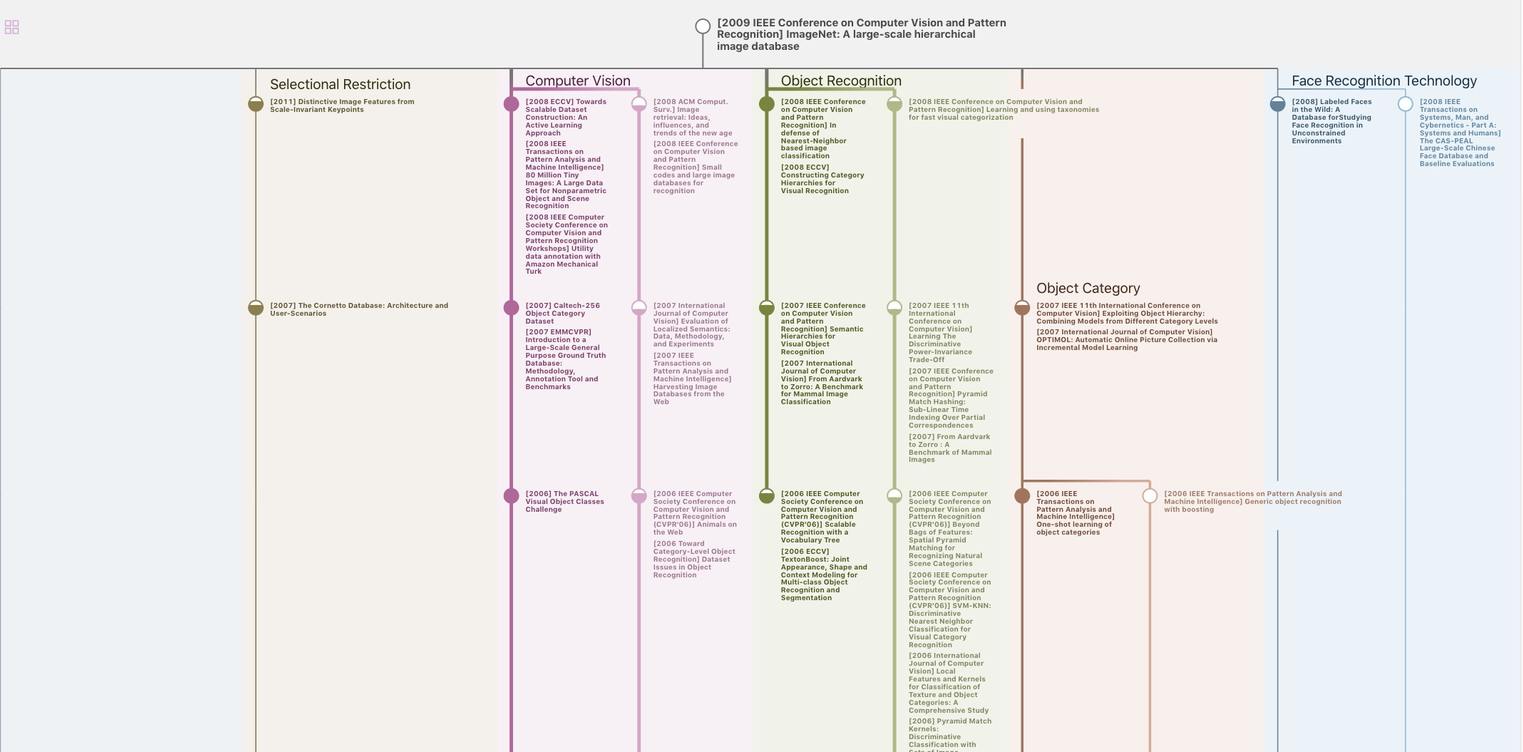
生成溯源树,研究论文发展脉络
Chat Paper
正在生成论文摘要