Improvement of power equipment defect text quality based on improved BI-LSTM
CSEE Journal of Power and Energy Systems(2024)
摘要
The power equipment defect text is an important data generated by operation and maintenance of power grid system. It is characterized by having a large number of professional words from the power field and high complexity. During model training, there are some problems such as difficulty in semantic understanding, gradient disappearance, negative information loss, and data imbalance, which hurt the text quality and defect analysis effect. In order to deal with these issues, this paper proposes an ULF-BI-LSTM text quality improvement algorithm that integrates UCNN, LeakyRelu activation function, and Focal Loss function. Then, we correctly separate professional vocabulary, delete invalid vocabulary, and normalize the object description by text data preprocessing. Finally, we fill the missed data and correct the wrong data by the ULF-BI-LSTM algorithm. The experimental results show that, the three improvement strategies effectively improve the accuracy, precision, F1, and recall of the algorithm, and the proposed algorithm outperforms the mainstream algorithm TextCNN, SVM, BI-LSTM, which alleviates the above problems well.
更多查看译文
关键词
BI-LSTM,natural language processing,power equipment defect text,text quality improvement
AI 理解论文
溯源树
样例
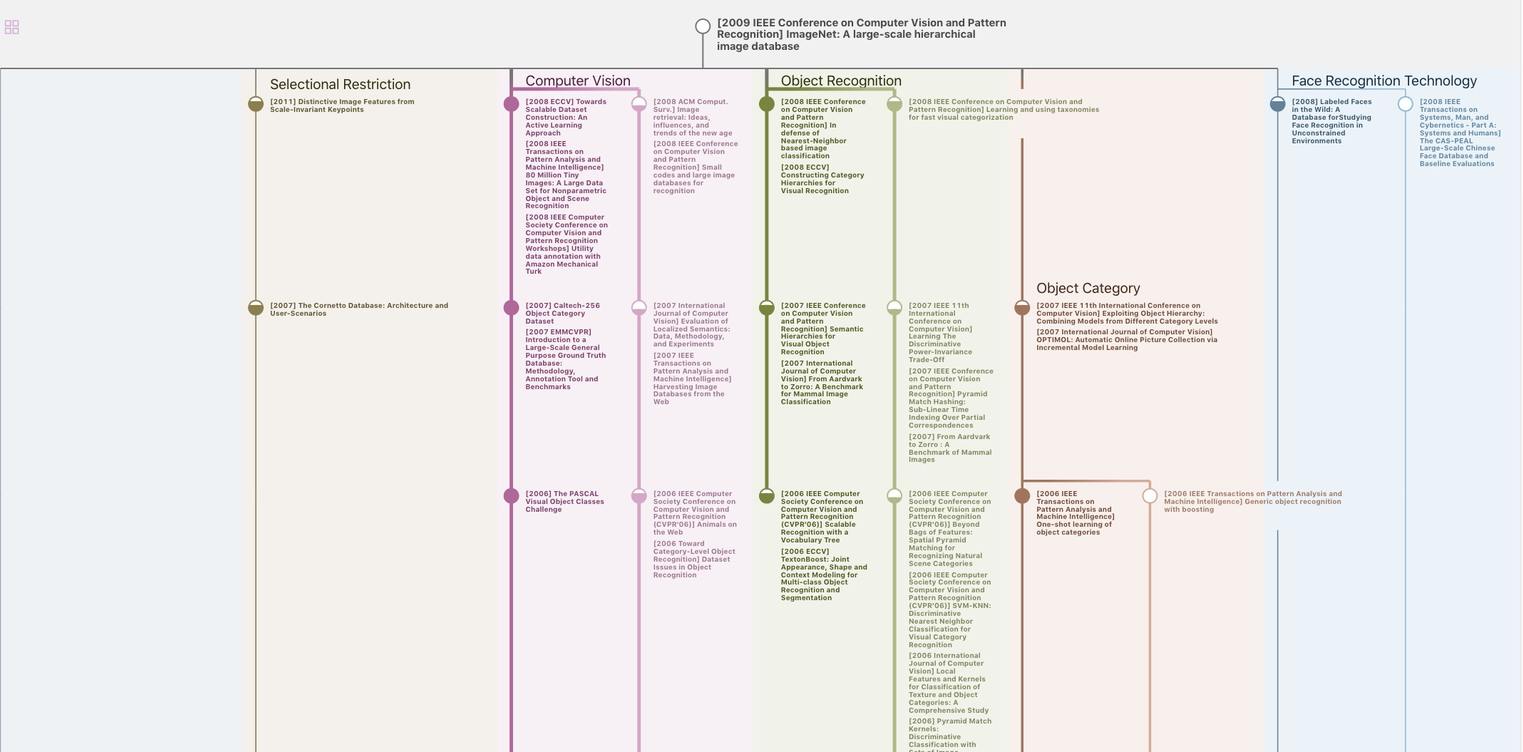
生成溯源树,研究论文发展脉络
Chat Paper
正在生成论文摘要