Compound Jamming Recognition via Contrastive Learning for Distributed MIMO Radars
IEEE Transactions on Vehicular Technology(2024)
摘要
As new active deception jamming technologies are emerging, it has become increasingly crucial to have accurate sensing of such jamming to facilitate effective radar anti-jamming and target detection, especially for distributed MIMO radars operating in complex electromagnetic environments. Deep learning, specifically convolutional neural networks (CNN), has been increasingly utilized in radar jamming recognition in recent years. However, enhancing the accuracy of deep learning algorithms with limited data remains a challenge. Furthermore, contrastive learning based on CNN has proven to be effective in enhancing the model's generalization performance. This article proposes a recognition algorithm for radar active deception jamming based on contrastive learning and tensor decomposition (CL-TD), specifically designed for recognizing compound jamming with relatively large sub-jamming energy difference in scenarios with limited samples. Specifically, the proposed method aims to decompose the compound jamming signal into single jamming signal resorting to tensor decomposition algorithm. Then, the signal features of single jamming are then extracted by applying contrastive learning, even with a small number of labeled samples. Finally, by supervised training of both the feature extraction and classification modules, the classification results of jamming signals can be obtained. The proposed method outperforms several state-of-the-art methods in terms of recognition performance, as demonstrated in experiments conducted on a simulated dataset consisting of 12 different sample types.
更多查看译文
关键词
Compound jamming recognition,small sample,contrastive learning,tensor decomposition,distributed MIMO radars
AI 理解论文
溯源树
样例
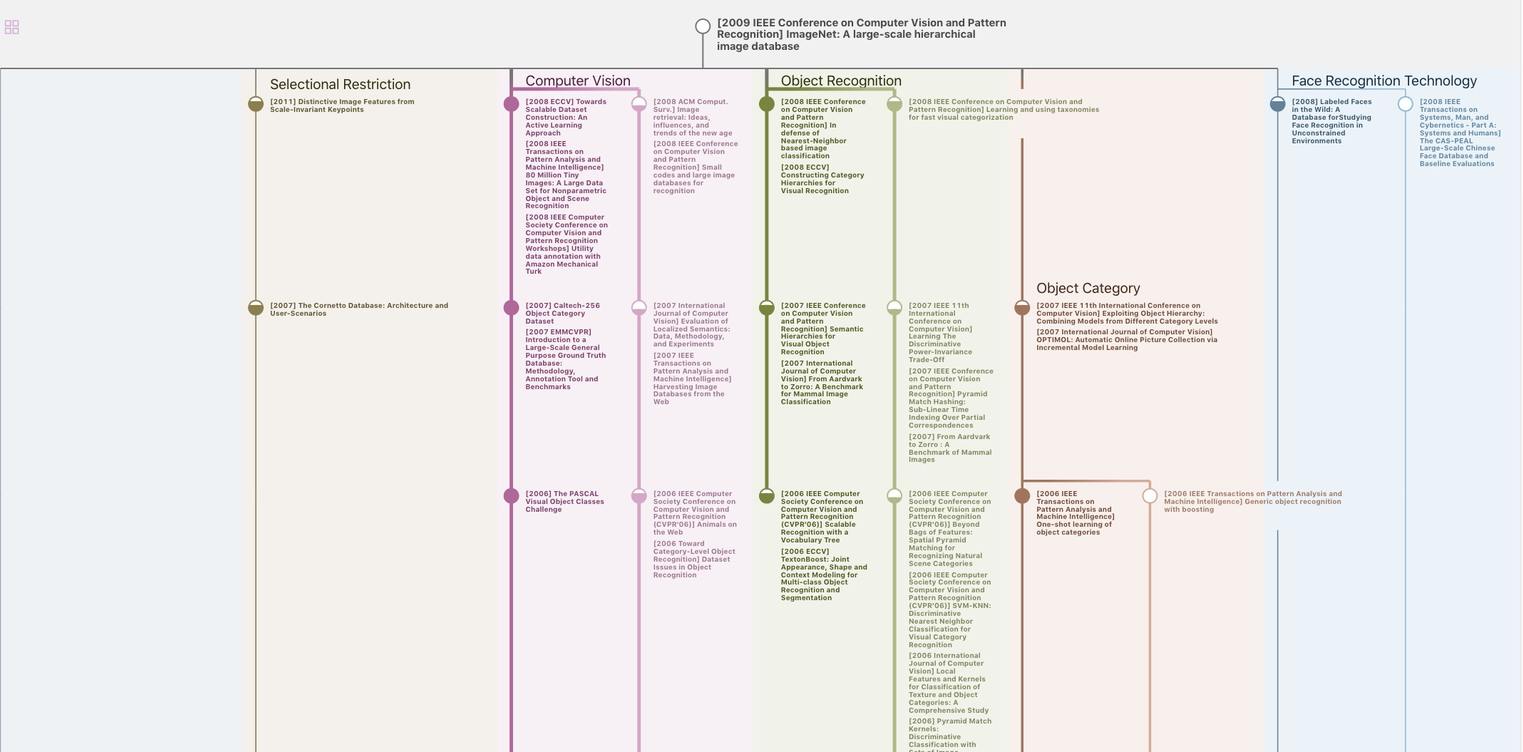
生成溯源树,研究论文发展脉络
Chat Paper
正在生成论文摘要