A Spatial-Temporal Gated Hypergraph Convolution Network for Traffic Prediction
IEEE Transactions on Vehicular Technology(2024)
摘要
As one of the most significant components of Intelligent Transportation Systems (ITS), traffic prediction has gained much popularity given its enormous application value in vehicular communications, traffic management, and traffic control. As such, many traffic prediction models have been proposed. The current methods commonly adopt graph convolution networks (GCNs) to capture spatial correlations. GCN-based approaches mainly focus on pair-wise interactions between road vertices (i.e. dyadic relations). However, the interactions between road vertices are not necessarily dyadic, but also can be high-order (i.e., multivariate relations). Further, few existing works focus on how to mine correlations between different types of traffic data. To this end, we develop STGHCN, a spatial-temporal gated hypergraph convolution network, that not only captures pair-wise and high-order spatial patterns between vertices but also characterizes the type-aware traffic data influences. Specifically, we adopt a spatial gated graph convolution and a hypergraph convolution to explore pair-wise and high-order interactions. More importantly, we design a spatial-temporal aware channel attention mechanism to mine hidden patterns between cross-type traffic features. Extensive experiments conducted on four real-world traffic datasets validate the effectiveness of STGHCN, with STGHCN's MAE, RMSE, and MAPE at between 6.91%-10.00%, 5.42%-8.90%, and 2.54%-5.03% lower than the state-of-the-art methods.
更多查看译文
关键词
Intelligent transportation systems,traffic prediction,hypergraph convolution,channel attention mechanism,spatial-temporal correlations
AI 理解论文
溯源树
样例
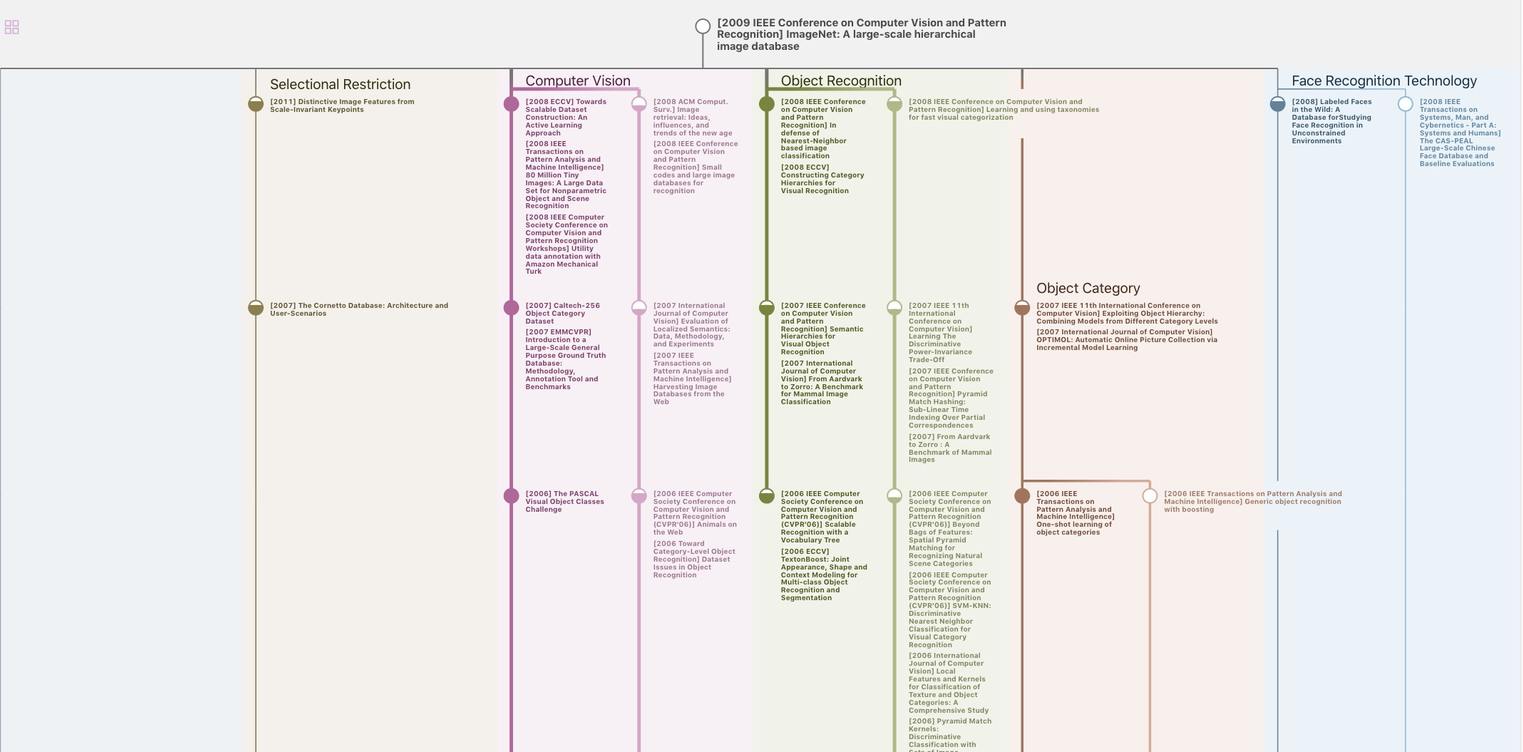
生成溯源树,研究论文发展脉络
Chat Paper
正在生成论文摘要