Scalable Reinforcement Learning Framework for Traffic Signal Control Under Communication Delays
IEEE OPEN JOURNAL OF VEHICULAR TECHNOLOGY(2024)
摘要
Vehicle-to-everything (V2X) technology is pivotal for enhancing road safety, traffic efficiency, and energy conservation through the communication of vehicles with their surrounding entities such as other vehicles, pedestrians, roadside infrastructure, and networks. Among these, traffic signal control (TSC) plays a significant role in roadside infrastructure for V2X. However, most existing works on TSC design assume that real-time traffic flow information is accessible, which does not hold in real-world deployment. This study proposes a two-stage framework to address this issue. In the first stage, a scene prediction module and a scene context encoder are utilized to process historical and current traffic data to generate preliminary traffic signal actions. In the second stage, an action refinement module, informed by human-defined traffic rules and real-time traffic metrics, adjusts the preliminary actions to account for the latency in observations. This modular design allows device deployment with varying computational resources while facilitating system customization, ensuring both adaptability and scalability, particularly in edge-computing environments. Through extensive simulations on the SUMO platform, the proposed framework demonstrates robustness and superior performance in diverse traffic scenarios under varying communication delays. The related code is available at https://github.com/Traffic-Alpha/TSC-DelayLight.
更多查看译文
关键词
Delays,Green products,Computational modeling,Vehicle-to-everything,Real-time systems,Predictive models,Feature extraction,Traffic signal control,scalable framework,historical-future data fusion,reinforcement learning
AI 理解论文
溯源树
样例
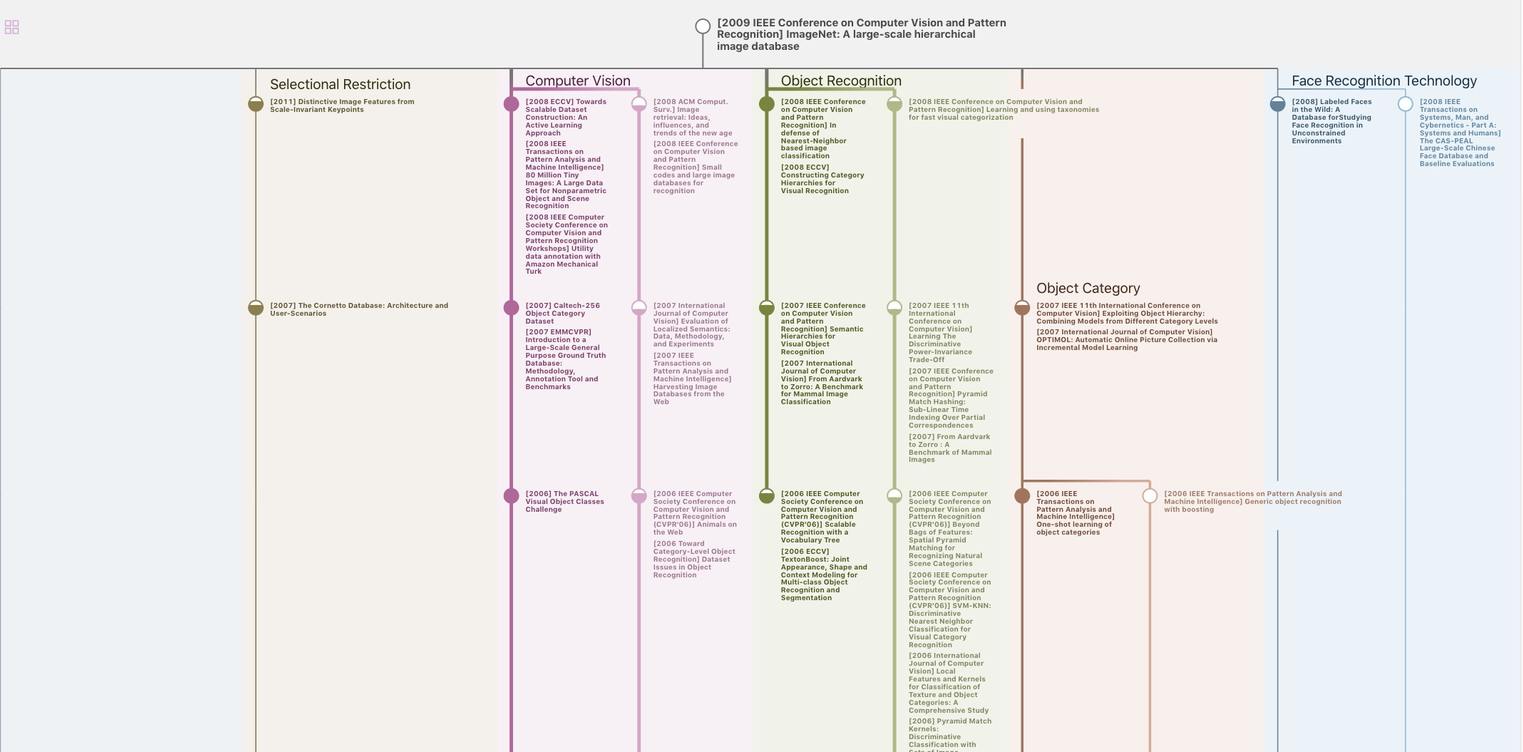
生成溯源树,研究论文发展脉络
Chat Paper
正在生成论文摘要