Multipath-Assisted Single-Anchor Localization via Deep Variational Learning
IEEE Transactions on Wireless Communications(2024)
摘要
Location awareness plays an increasingly important role in wireless network applications. However, accurate localization in complex indoor environments remains challenging for existing radio frequency (RF)-based systems, among which the ultra-wide bandwidth (UWB) technology ranks to be the most promising one due to its capability in providing channel information with fine time resolution. In this paper, we propose a multipath-assisted single-anchor localization framework that can provide high-accuracy positional information in complex indoor environments. Specifically, a deep variational learning method is proposed to produce calibrated estimates of position-related parameters, including distance, time-difference-of-arrival and angle-of-arrival, which are then fed into a multipath-assisted single-anchor localization algorithm. The proposed method is implemented on self-built UWB transceivers and assessed with real-world data from an indoor measurement campaign. Extensive experimental results show that the proposed method outperforms conventional machine learning-based error mitigation approaches and can achieve 0.15m root mean square position error in non-line-of-sight scenarios.
更多查看译文
关键词
Single-anchor localization,ultra-wide bandwidth,channel impulse response,multipath components,variational inference,deep learning
AI 理解论文
溯源树
样例
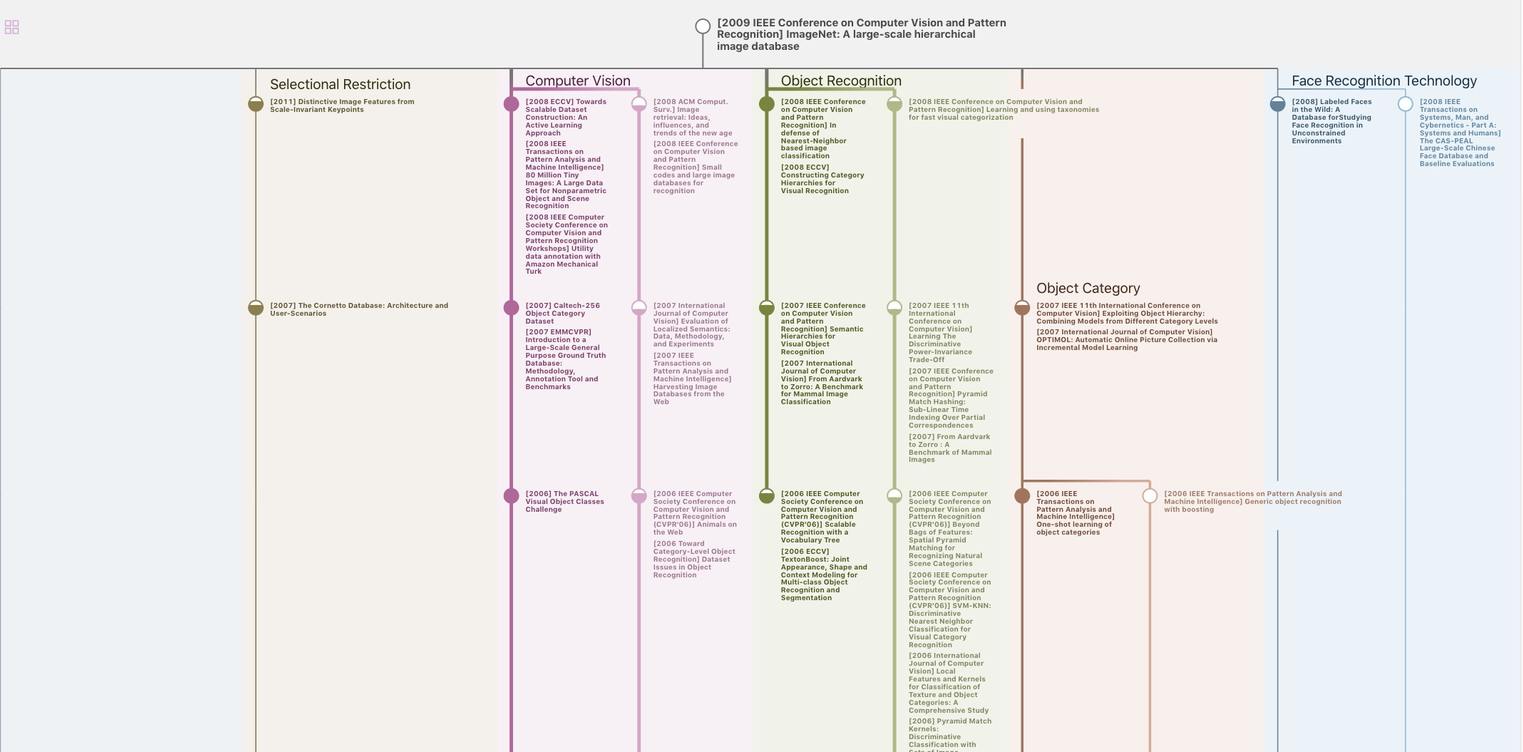
生成溯源树,研究论文发展脉络
Chat Paper
正在生成论文摘要