ATGAN: A SAR Target Image Generation Method for Automatic Target Recognition
IEEE JOURNAL OF SELECTED TOPICS IN APPLIED EARTH OBSERVATIONS AND REMOTE SENSING(2024)
摘要
The performance of a deep learning-based synthetic aperture radar (SAR) automatic target recognition (ATR) model largely relies on the scale and quality of training samples. However, it is time-consuming and expensive to collect sufficient data in practice. Although generative adversarial network (GAN) provides a way for SAR target image generation, existing GAN-based methods cannot confirm what features the generator learns, thus they struggle in generating precise SAR target images. In this article, we propose an angle transformation GAN (ATGAN) that can generate azimuth-controllable SAR target images while preserving the target details. The key idea of our ATGAN is to reframe the generation task from the perspective of image-to-image translation. To this end, ATGAN consists of two modules, a coarse-to-fine generator that aims to learn the angle transformation in the deep feature space, and then, apply it to manipulate the representation of an input SAR target image to generate a new one, while a spectral-normalized patch discriminator that tries to estimate the probability that an input SAR target image is real rather than fake using a patch-averaged strategy. Combining with spatial transformer and adversarial training paradigm, ATGAN can generate precise SAR target images for ATR. Extensive experiments verify the effectiveness of the proposed ATGAN, and our method outperforms the state-of-the-art method qualitatively and quantitatively.
更多查看译文
关键词
Angle transformation (AT),automatic target recognition (ATR),generative adversarial network (GAN),synthetic aperture radar (SAR) target image generation
AI 理解论文
溯源树
样例
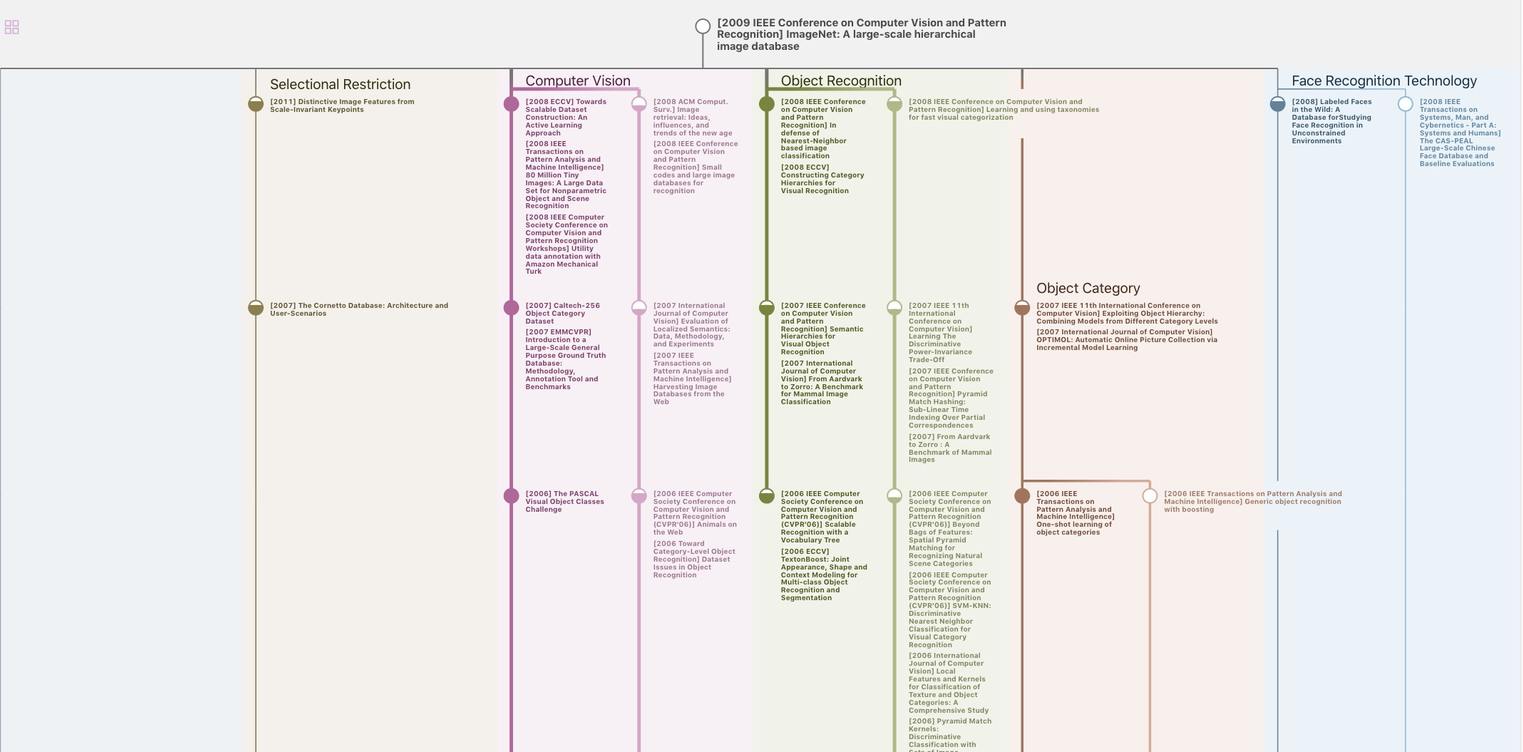
生成溯源树,研究论文发展脉络
Chat Paper
正在生成论文摘要