Multi-Modal and Multi-Task Depression Detection with Sentiment Assistance.
IEEE International Conference on Consumer Electronics(2024)
摘要
Depression, a multifaceted mental health disorder, is characterized by persistent feelings of sorrow, hopelessness, and a pervasive loss of interest or pleasure in once-enjoyed activities. It often manifests with physical and cognitive symptoms, including alterations in appetite and sleep patterns, overwhelming fatigue, difficulty in maintaining focus, and recurrent contemplations of death or suicide. Psychological research has unveiled a profound connection between depressive emotions, the expression of those emotions, and their perception. This intricate relationship underscores the paramount importance of comprehending how individuals with depression both undergo and convey their emotional experiences. This study enhances the precision of depression detection through a multimodal, multi-task learning approach. It combines the depression detection dataset(the AVEC 2019 Detecting Depression with AI Sub-challenge_with the sentiment analysis dataset, CMU-MOSEI. By harnessing emotional data, this method significantly augments the accuracy of depression detection. The efficacy of this proposed approach is validated using a publicly available dataset, AVEC 2019. It surpasses existing state-of-the-art methods, achieving a Concordance Correlation Coefficient (CCC) of 0.466 and a Mean Absolute Error (MAE) of 5.21 on the AVEC 2019 DDS test set. This represents a notable 5.4% improvement over the accuracy achieved by the current state-of-the-art method, which had a CCC of 0.442.
更多查看译文
关键词
Depression detection,Sentiment analysis,Multi-task Learning,Multimodality
AI 理解论文
溯源树
样例
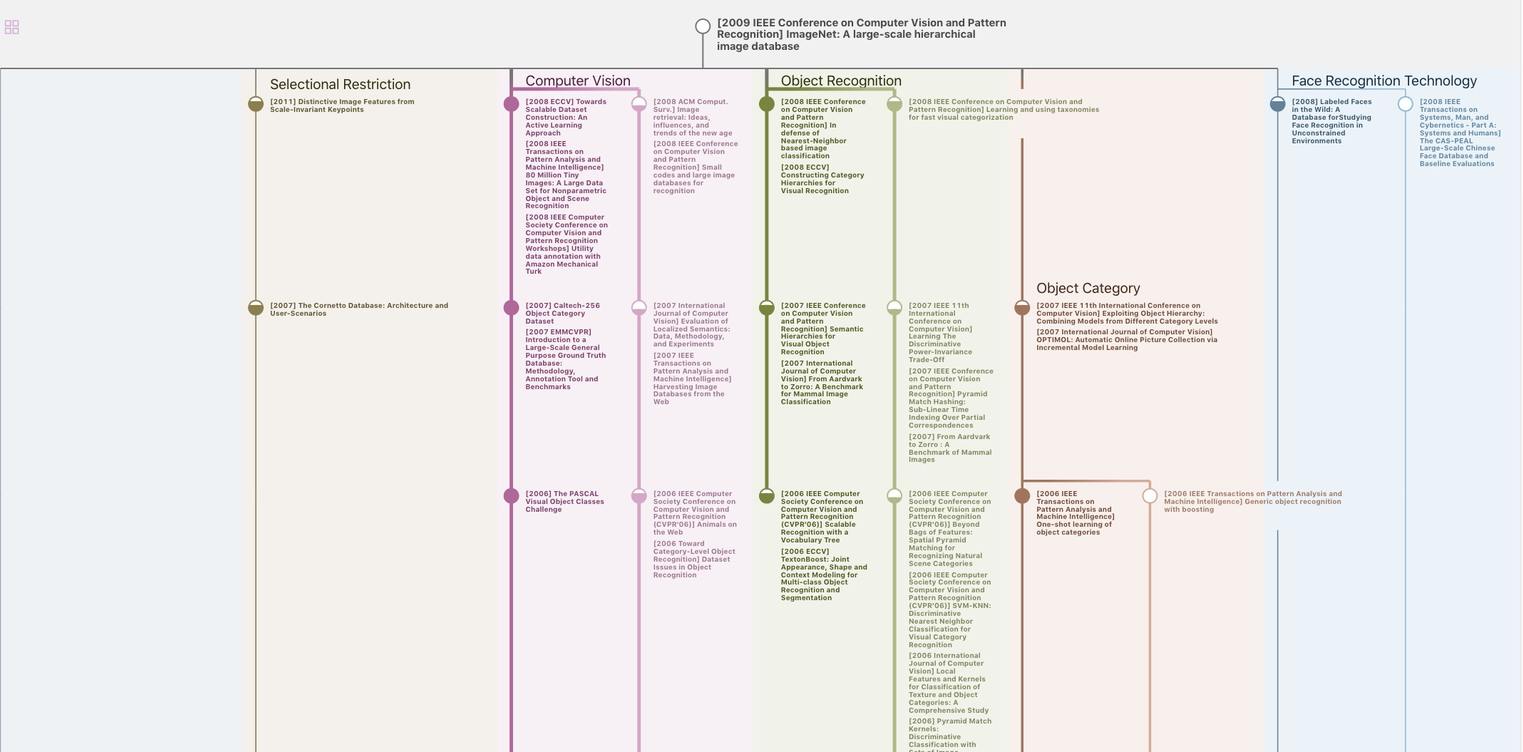
生成溯源树,研究论文发展脉络
Chat Paper
正在生成论文摘要