Extraction of Roads from Satellite Images Using U-Net-Based Semantic Segmentation Architecture
2023 3rd International Conference on Smart Generation Computing, Communication and Networking (SMART GENCON)(2023)
摘要
Roads are one of the most essential man-made things, and their autonomous extraction is a major worry. This research suggests extracting roads based on image segmentation applied to high-resolution satellite images through transfer learning using an encoder-decoder architectural framework based on U-Net with ResNet-50 as the encoder backbone, which is a pre-trained model on ImageNet dataset. The training of the model was performed on the open-source Massachusetts roads dataset, which is one of the biggest and hardest aerial image labelling datasets consisting of a huge range of urban, suburban, and rural places. The goal is to extract roads out of this dataset. The suggested approach boosts the mean Dice coefficient (mDC) to a value of 0.782 and raises the mean intersection-over-union (mIoU) score to 0.643.
更多查看译文
关键词
automatic road extraction,Massachusetts roads dataset,ResNet-50,satellite images,semantic segmentation,U-Net
AI 理解论文
溯源树
样例
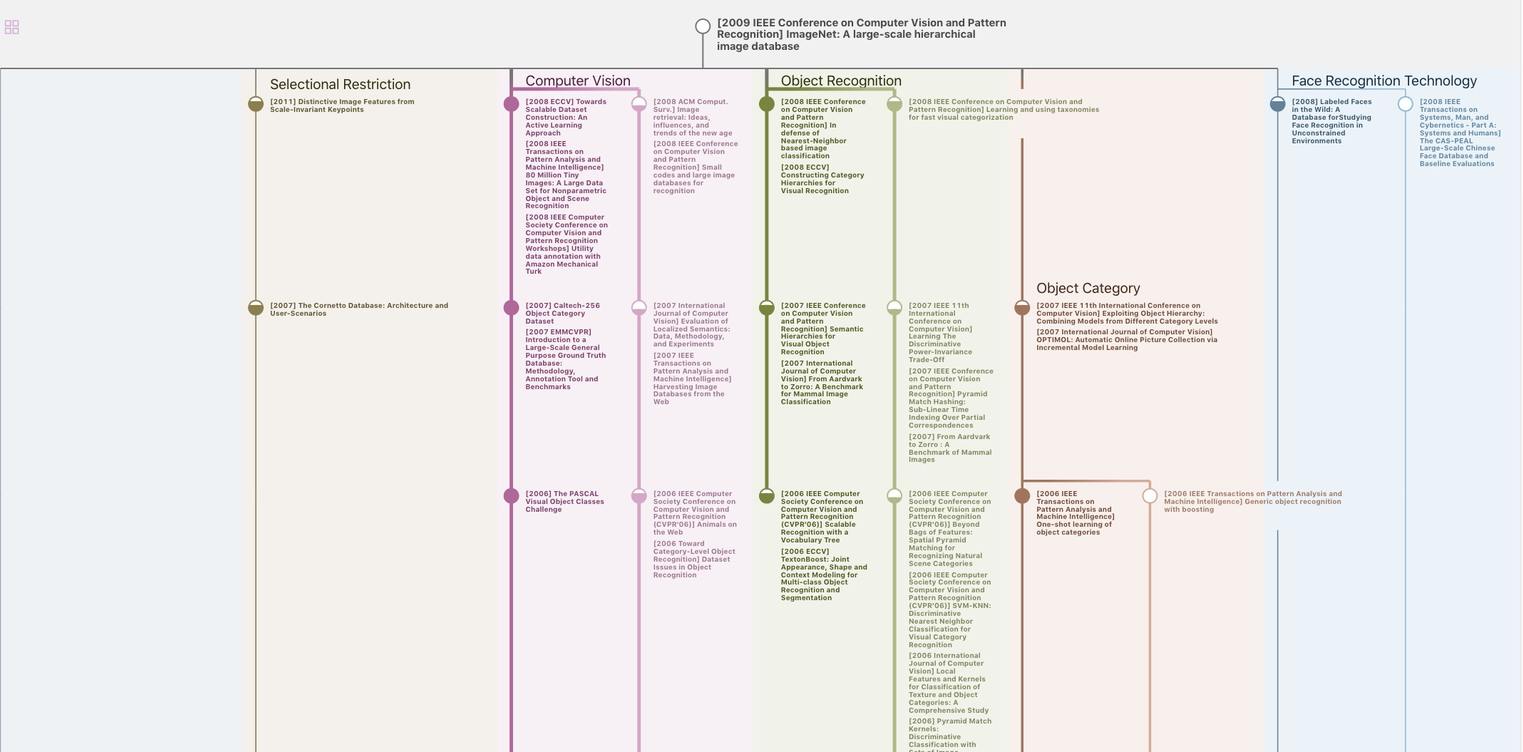
生成溯源树,研究论文发展脉络
Chat Paper
正在生成论文摘要