PMF-CNN: parallel multi-band fusion convolutional neural network for SSVEP-EEG decoding
BIOMEDICAL PHYSICS & ENGINEERING EXPRESS(2024)
摘要
Steady-state visual evoked potential (SSVEP) is a key technique of electroencephalography (EEG)-based brain-computer interfaces (BCI), which has been widely applied to neurological function assessment and postoperative rehabilitation. However, accurate decoding of the user's intended based on the SSVEP-EEG signals is challenging due to the low signal-to-noise ratio and large individual variability of the signals. To address these issues, we proposed a parallel multi-band fusion convolutional neural network (PMF-CNN). Multi frequency band signals were served as the input of PMF-CNN to fully utilize the time-frequency information of EEG. Three parallel modules, spatial self-attention (SAM), temporal self-attention (TAM), and squeeze-excitation (SEM), were proposed to automatically extract multi-dimensional features from spatial, temporal, and frequency domains, respectively. A novel spatial-temporal-frequency representation were designed to capture the correlation of electrode channels, time intervals, and different sub-harmonics by using SAM, TAM, and SEM, respectively. The three parallel modules operate independently and simultaneously. A four layers CNN classification module was designed to fuse parallel multi-dimensional features and achieve the accurate classification of SSVEP-EEG signals. The PMF-CNN was further interpreted by using brain functional connectivity analysis. The proposed method was validated using two large publicly available datasets. After trained using our proposed dual-stage training pattern, the classification accuracies were 99.37% and 93.96%, respectively, which are superior to the current state-of-the-art SSVEP-EEG classification algorithms. The algorithm exhibits high classification accuracy and good robustness, which has the potential to be applied to postoperative rehabilitation.
更多查看译文
关键词
deep learning,SSVEP-EEG,accurate decoding,multi-dimensional,BCI
AI 理解论文
溯源树
样例
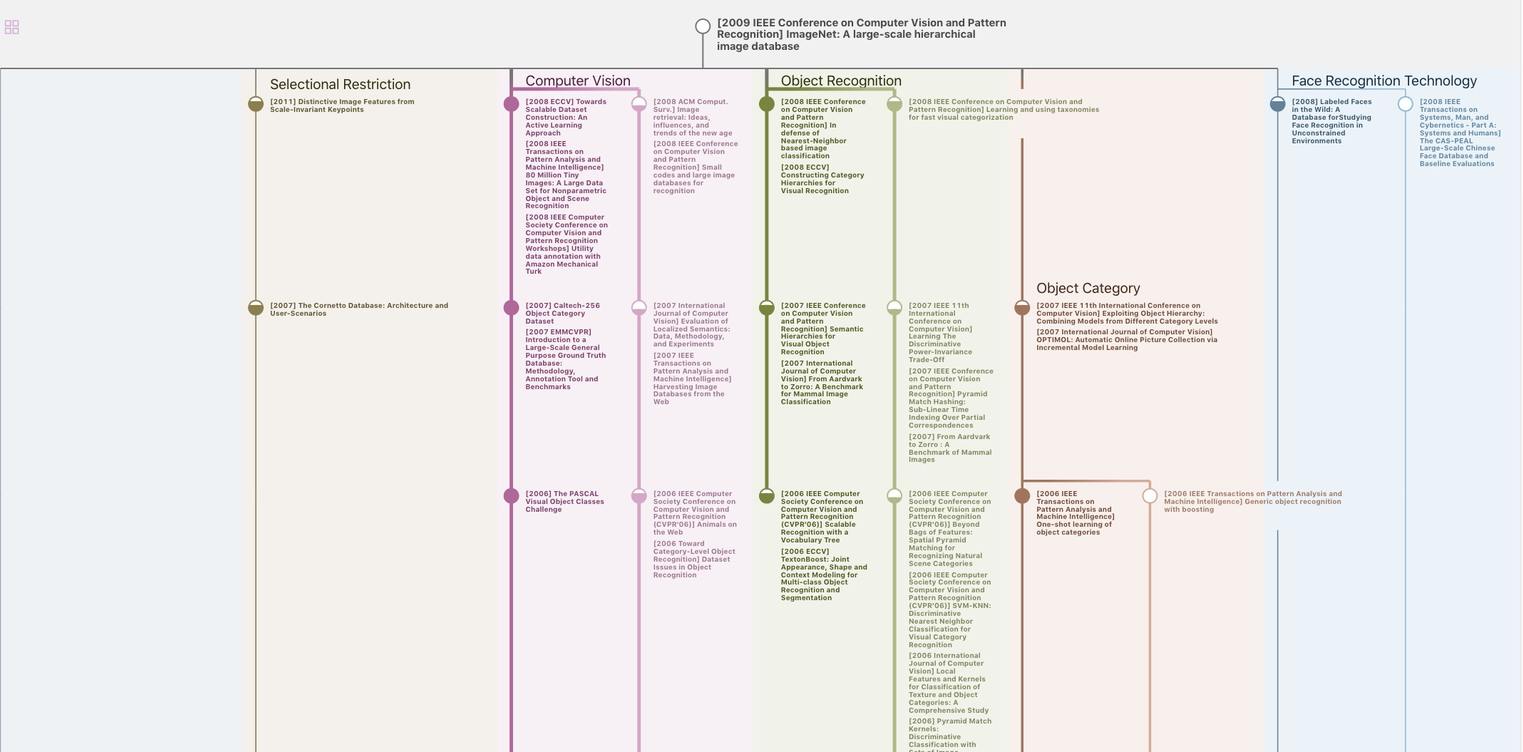
生成溯源树,研究论文发展脉络
Chat Paper
正在生成论文摘要