Compressed Gradient Tracking Algorithm for Distributed Aggregative Optimization
IEEE Transactions on Automatic Control(2024)
摘要
This paper is devoted to addressing the distributed aggregative optimization (DAO) problem via compressed gradient tracking algorithms, where the cost function of each agent relies on the aggregation of other agents' decisions as well as its own decision. To this end, a new kind of distributed aggregative gradient tracking algorithm with compression communication is developed based upon the gradient tracking algorithm and the technique of communication compression. Under the scenario with a time-invariant and balanced graph, it is theoretically shown that the present algorithm owns a linear convergence rate (in the mean square error sense) with the strongly convex and smooth cost functions. Furthermore, the result is extended to a more general case with the time-varying graphs considered. Specifically, it is proven that the developed algorithm could converge linearly to the optimal solution of the DAO problem (in the mean square error sense) if the time-varying balanced graph is jointly strongly connected and some suitable conditions are satisfied. With the optimal placement problem considered, some numerical simulation results are performed to validate the theoretical results.
更多查看译文
关键词
Communication compression,distributed aggregative optimization,gradient tracking algorithm,time-varying graph
AI 理解论文
溯源树
样例
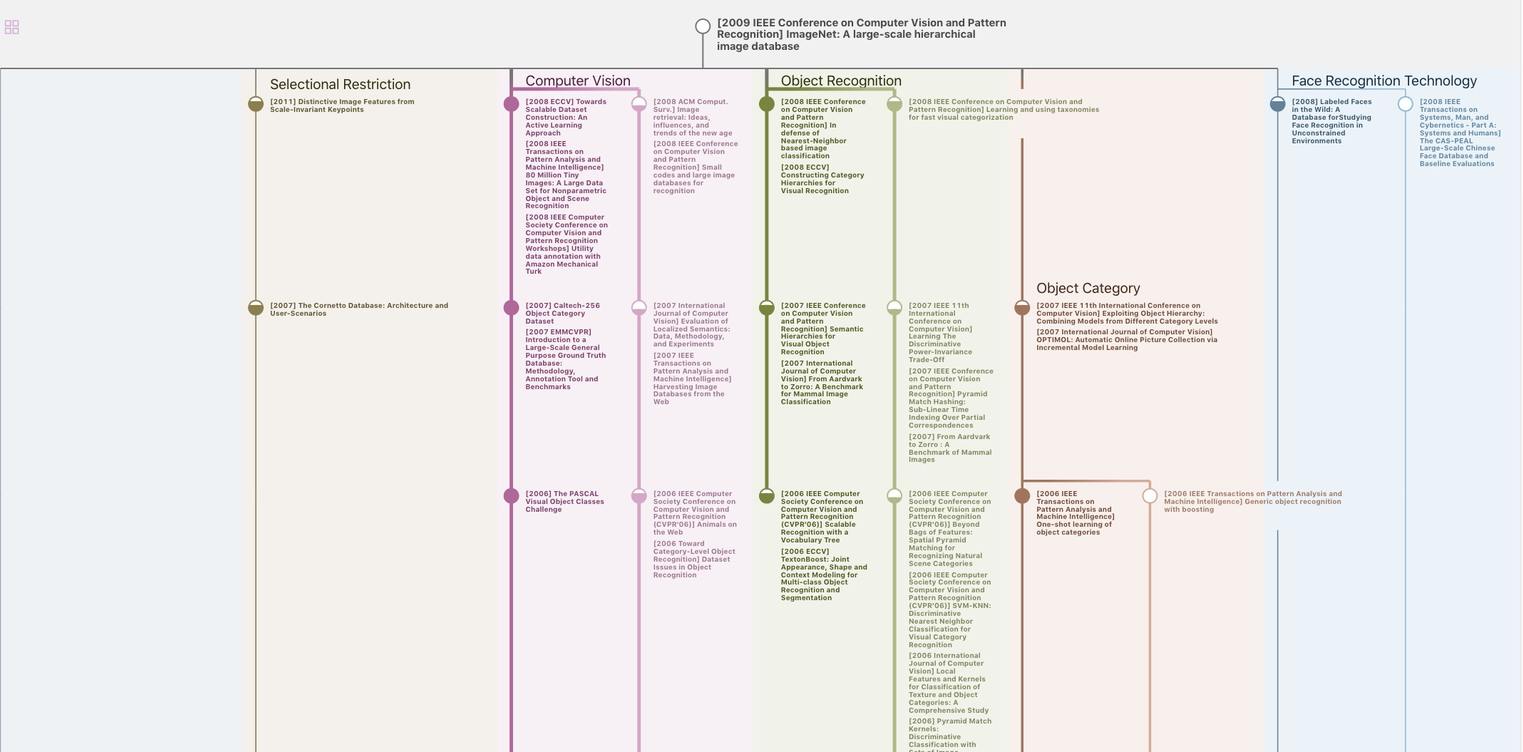
生成溯源树,研究论文发展脉络
Chat Paper
正在生成论文摘要