Fairness-Aware Computation Offloading With Trajectory Optimization and Phase-Shift Design in RIS-Assisted Multi-UAV MEC Network
IEEE Internet of Things Journal(2024)
摘要
Unmanned aerial vehicles (UAVs) are regarded as a promising solution for mobile edge computing (MEC) systems due to their flexibility and capability to provide computing services to ground terminals (GTs). By leveraging UAVs, the latency in computation tasks can be reduced significantly, particularly in disaster scenarios. Additionally, Reconfigurable Intelligent Surfaces (RIS) have emerged as a novel technology for enhancing the wireless propagation environment in wireless networks. This paper proposes a multi-UAV assisted MEC system where computation tasks of GTs can be computed locally or partially offloaded to UAVs. Furthermore, practical RIS phase shift designs are considered to enhance the communication performance between GTs and UAVs. To minimize the system delay and achieve fairness among GTs, the computation offloading strategy, trajectory of the UAVs are optimized using a markov decision process. Simultaneously, the RIS phase shift is optimized through an alternating optimization algorithm. Additionally, a cooperative multi-agent deep reinforcement learning framework is developed to obtain a optimal solution by employing the multi-agent twin delayed deep deterministic policy gradient (MATD3) algorithm. Numerical results indicate that MATD3 can effectively improve the system delay and fairness performance of the RIS-assisted multi-UAV MEC system, as compared to benchmark solutions.
更多查看译文
关键词
Mobile edge computing (MEC),unmanned aerial vehicle (UAV),reconfigurable intelligent surface (RIS),deep reinforcement learning (DRL),fairness
AI 理解论文
溯源树
样例
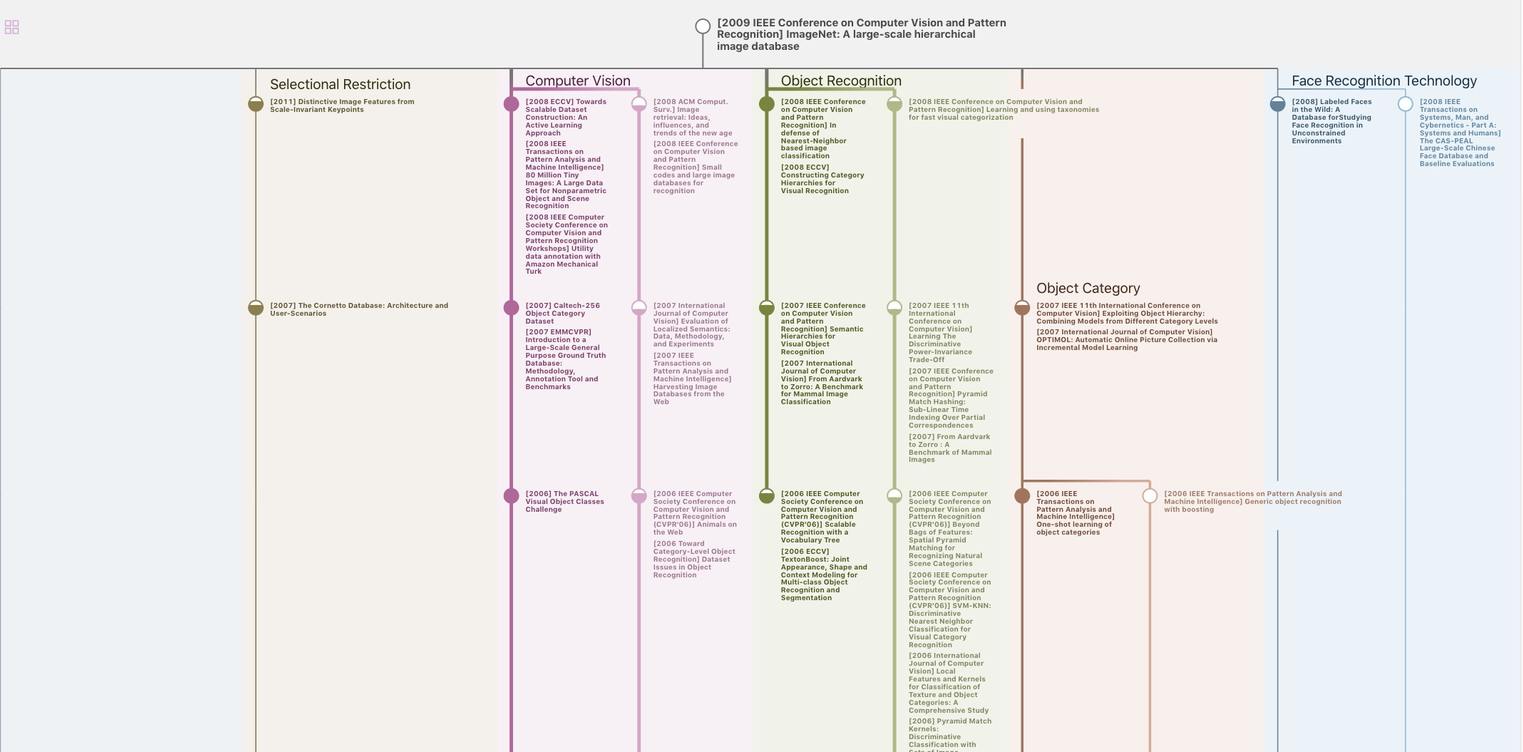
生成溯源树,研究论文发展脉络
Chat Paper
正在生成论文摘要