A Priori Knowledge-Based Physics-Informed Neural Networks for Electromagnetic Inverse Scattering
IEEE transactions on geoscience and remote sensing(2024)
Abstract
Based on the physics-informed neural network (PINN) method, a two-step inverse scattering method is proposed to improve the efficiency and accuracy of the inversion in this work. The first step is to calculate the total fields and the initial solution of permittivity distribution in the domain of interest (DoI) by a traditional inversion algorithm, the distorted finite-difference-frequency-domain-based iterative method (DFIM), as a priori information for the cascaded PINNs. The second step is to use the calculated a priori information as additional parts of the data loss term in the proposed PINN framework for network training. Several typical numerical examples and one experimental example are considered to validate the proposed method. Inversion results show that the proposed method has good accuracy, efficiency, and robustness to noise. Compared with the data-driven deep learning methods in electromagnetic inversion, the proposed method belongs to an unsupervised learning framework and can handle more general problems. Compared with the traditional inverse algorithms, it is more efficient and accurate. In general, the proposed two-step method inherits the advantages of both traditional deep learning methods and inverse scattering methods. Importantly, it also establishes the bridge between traditional inverse scattering algorithms and deep learning methods.
MoreTranslated text
Key words
Deep learning,electromagnetic inverse scattering,full-wave inversion,physics-informed neural network (PINN)
AI Read Science
Must-Reading Tree
Example
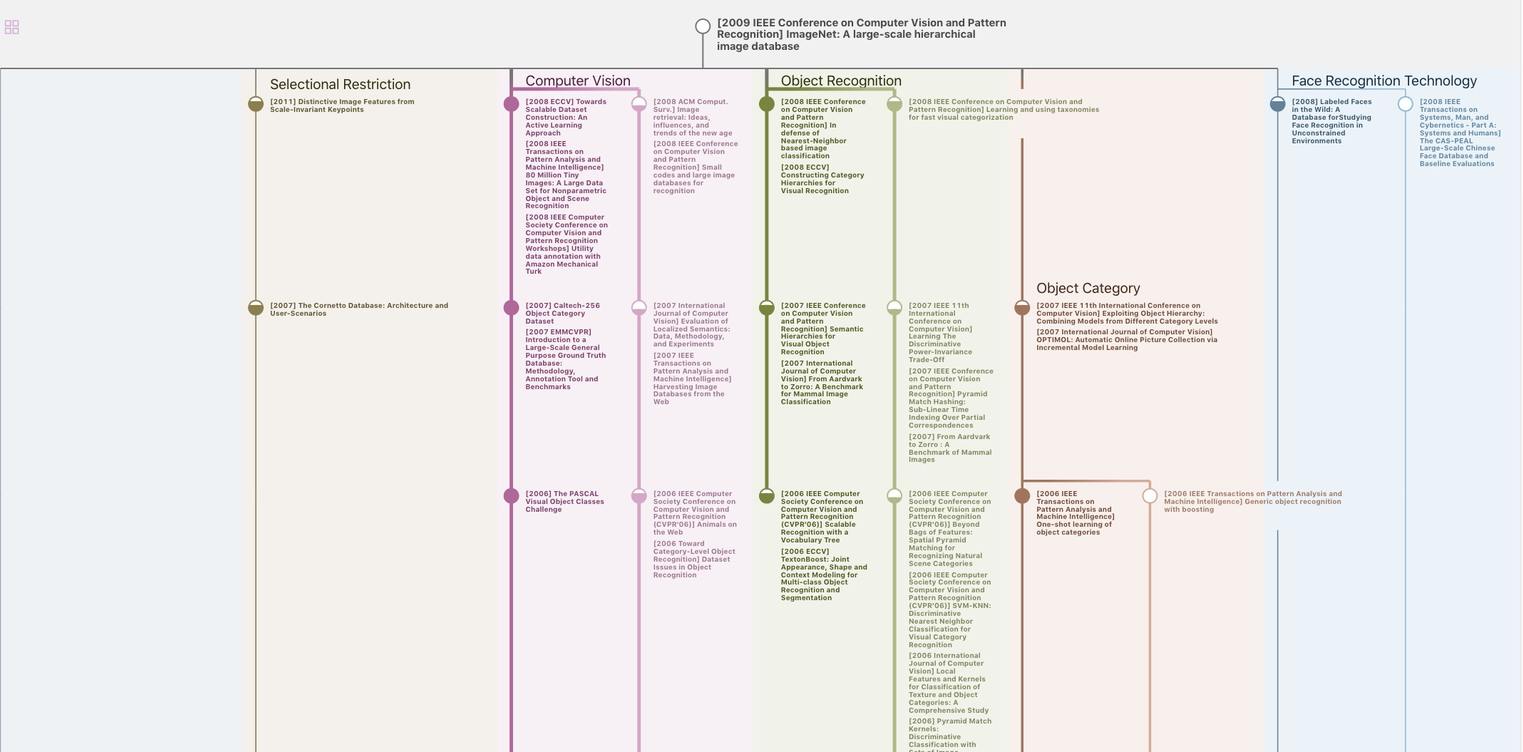
Generate MRT to find the research sequence of this paper
Chat Paper
Summary is being generated by the instructions you defined