Jointly Learning Selection Matrices For Transmitters, Receivers And Fourier Coefficients In Multichannel Imaging
ICASSP 2024 - 2024 IEEE International Conference on Acoustics, Speech and Signal Processing (ICASSP)(2024)
摘要
Strategic subsampling has become a focal point due to its effectiveness in
compressing data, particularly in the Full Matrix Capture (FMC) approach in
ultrasonic imaging. This paper introduces the Joint Deep Probabilistic
Subsampling (J-DPS) method, which aims to learn optimal selection matrices
simultaneously for transmitters, receivers, and Fourier coefficients. This
task-based algorithm is realized by introducing a specialized measurement model
and integrating a customized Complex Learned FISTA (CL-FISTA) network. We
propose a parallel network architecture, partitioned into three segments
corresponding to the three matrices, all working toward a shared optimization
objective with adjustable loss allocation. A synthetic dataset is designed to
reflect practical scenarios, and we provide quantitative comparisons with a
traditional CRB-based algorithm, standard DPS, and J-DPS.
更多查看译文
关键词
Compressed Sensing,Multichannel Imaging,Sparse Signal Recovery,Deep Learning,CVNNs
AI 理解论文
溯源树
样例
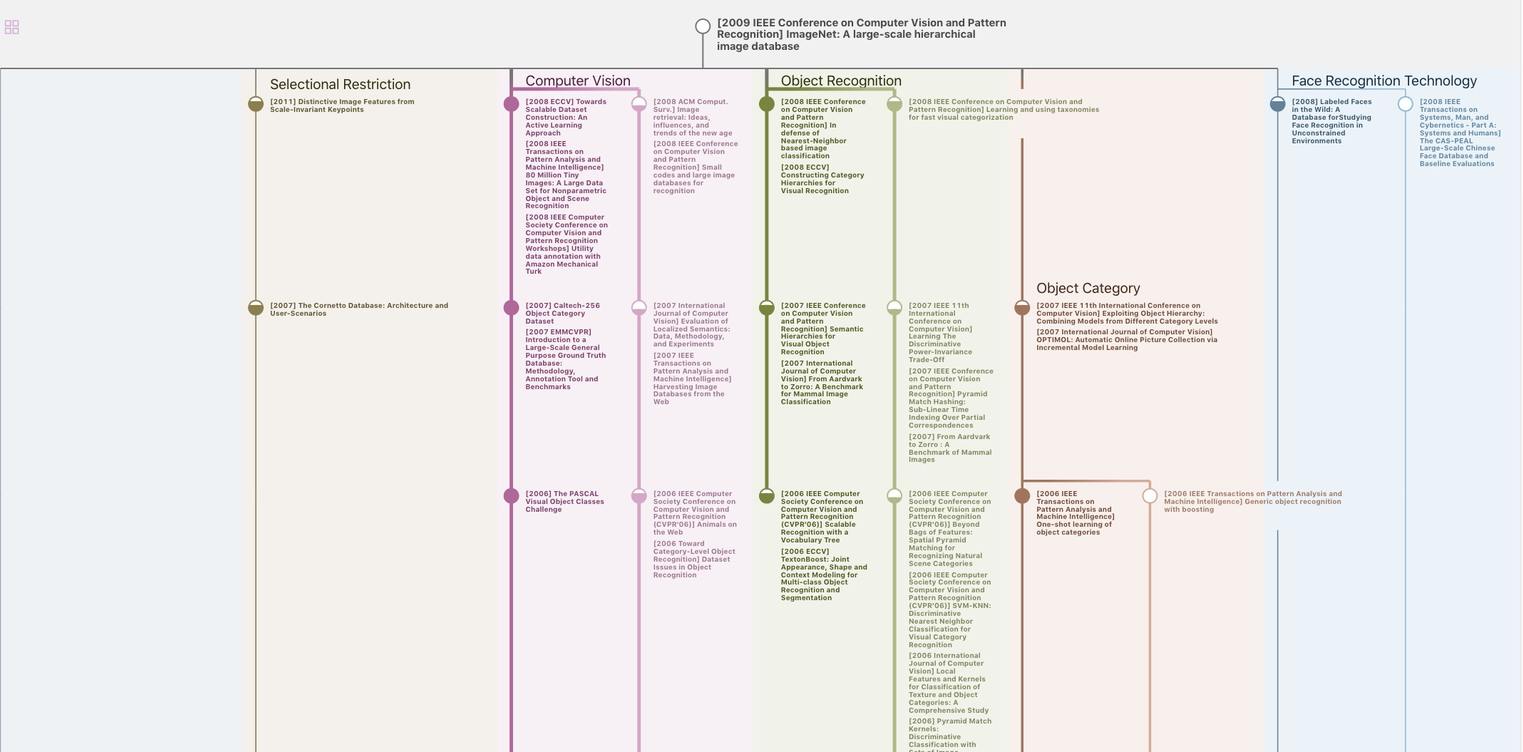
生成溯源树,研究论文发展脉络
Chat Paper
正在生成论文摘要