BSSE: Design Space Exploration on the BOOM with Semi-Supervised Learning
IEEE transactions on very large scale integration (VLSI) systems(2024)
摘要
With the rising prominence of RISC-V-based microprocessors in processor design, the challenge of exploring the vast and complex RISC-V microarchitecture design space has become increasingly apparent. We propose the Berkeley Out-of-Order Machine Semi-Supervised Explorer (BSSE)—a novel framework leveraging the semi-supervised learning method and parallel emulation to speed up and make tradeoffs on the RISC-V microarchitecture design space exploration (DSE). BSSE constructs the initial training dataset with the microarchitecture experimental design sampling (MEDS) method and then employs the cotraining-style k-nearest neighbors (Co-KNN) model to fit the microarchitecture features to the architectural metric value space. The trained Co-KNN model assists in searching a Pareto-optimal set with parallel emulation. Finally, a distance-based method is proposed to select a designer-preferred microarchitecture from the identified Pareto-optimal set. Extensive experiments on the Berkeley Out-of-Order Machine (BOOM) show that our proposed BSSE method can search for a better Pareto-optimal set with less time consumption compared to the state-of-the-art methods and can find microarchitectures that are equivalent to or even better than the existing manually designed BOOM microarchitectures.
更多查看译文
关键词
Microarchitecture,Measurement,Computer architecture,Training,Semisupervised learning,Data models,Space exploration,Berkeley Out-of-Order Machine (BOOM),design space exploration (DSE),microarchitecture,Pareto set,RISC-V
AI 理解论文
溯源树
样例
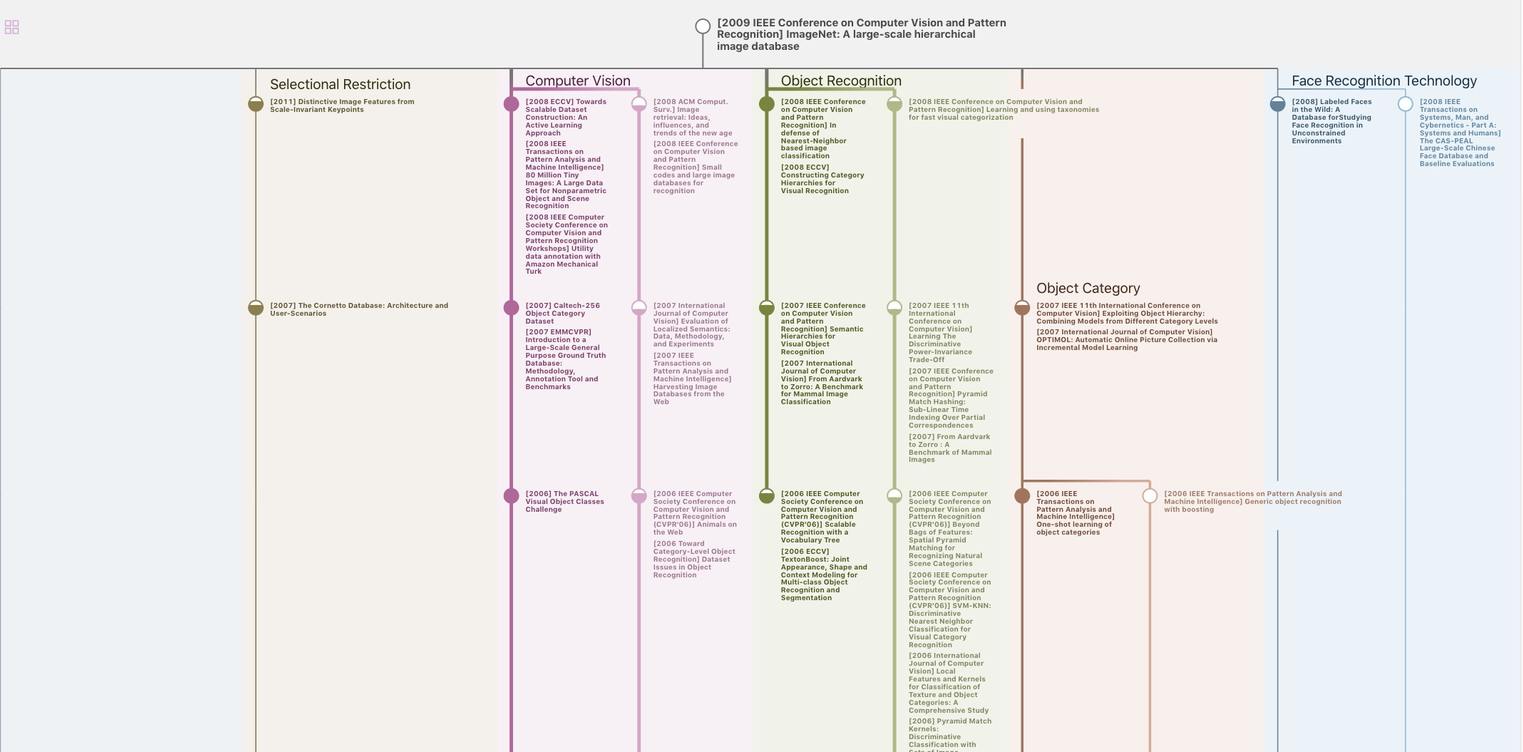
生成溯源树,研究论文发展脉络
Chat Paper
正在生成论文摘要