A Novel Approach to Industrial Defect Generation through Blended Latent Diffusion Model with Online Adaptation
CoRR(2024)
摘要
Effectively addressing the challenge of industrial Anomaly Detection (AD)
necessitates an ample supply of defective samples, a constraint often hindered
by their scarcity in industrial contexts. This paper introduces a novel
algorithm designed to augment defective samples, thereby enhancing AD
performance. The proposed method tailors the blended latent diffusion model for
defect sample generation, employing a diffusion model to generate defective
samples in the latent space. A feature editing process, controlled by a
"trimap" mask and text prompts, refines the generated samples. The image
generation inference process is structured into three stages: a free diffusion
stage, an editing diffusion stage, and an online decoder adaptation stage. This
sophisticated inference strategy yields high-quality synthetic defective
samples with diverse pattern variations, leading to significantly improved AD
accuracies based on the augmented training set. Specifically, on the widely
recognized MVTec AD dataset, the proposed method elevates the state-of-the-art
(SOTA) performance of AD with augmented data by 1.5
metrics AP, IAP, and IAP90, respectively. The implementation code of this work
can be found at the GitHub repository
https://github.com/GrandpaXun242/AdaBLDM.git
更多查看译文
AI 理解论文
溯源树
样例
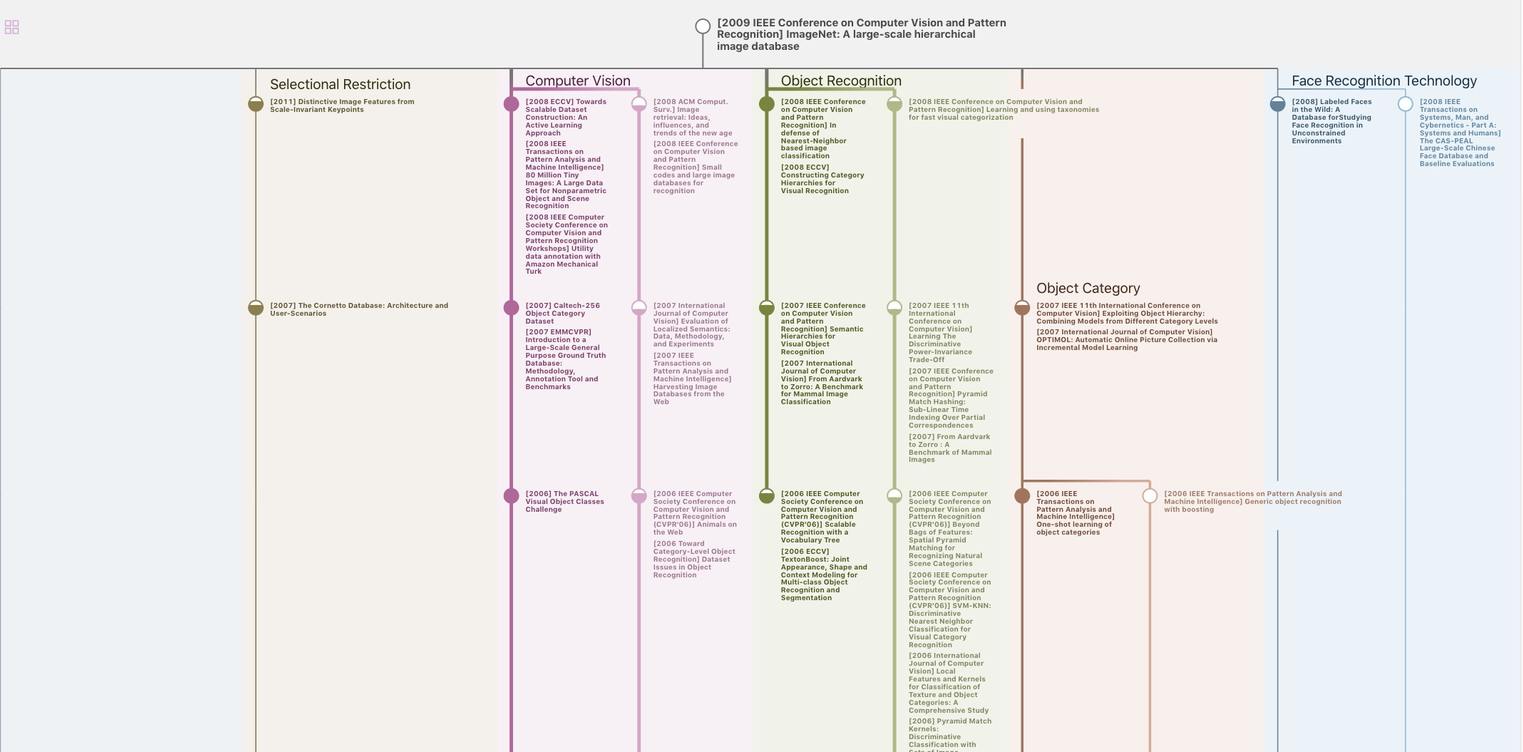
生成溯源树,研究论文发展脉络
Chat Paper
正在生成论文摘要