Loose LIPS Sink Ships: Asking Questions in Battleship with Language-Informed Program Sampling
CoRR(2024)
摘要
Questions combine our mastery of language with our remarkable facility for
reasoning about uncertainty. How do people navigate vast hypothesis spaces to
pose informative questions given limited cognitive resources? We study these
tradeoffs in a classic grounded question-asking task based on the board game
Battleship. Our language-informed program sampling (LIPS) model uses large
language models (LLMs) to generate natural language questions, translate them
into symbolic programs, and evaluate their expected information gain. We find
that with a surprisingly modest resource budget, this simple Monte Carlo
optimization strategy yields informative questions that mirror human
performance across varied Battleship board scenarios. In contrast, LLM-only
baselines struggle to ground questions in the board state; notably, GPT-4V
provides no improvement over non-visual baselines. Our results illustrate how
Bayesian models of question-asking can leverage the statistics of language to
capture human priors, while highlighting some shortcomings of pure LLMs as
grounded reasoners.
更多查看译文
AI 理解论文
溯源树
样例
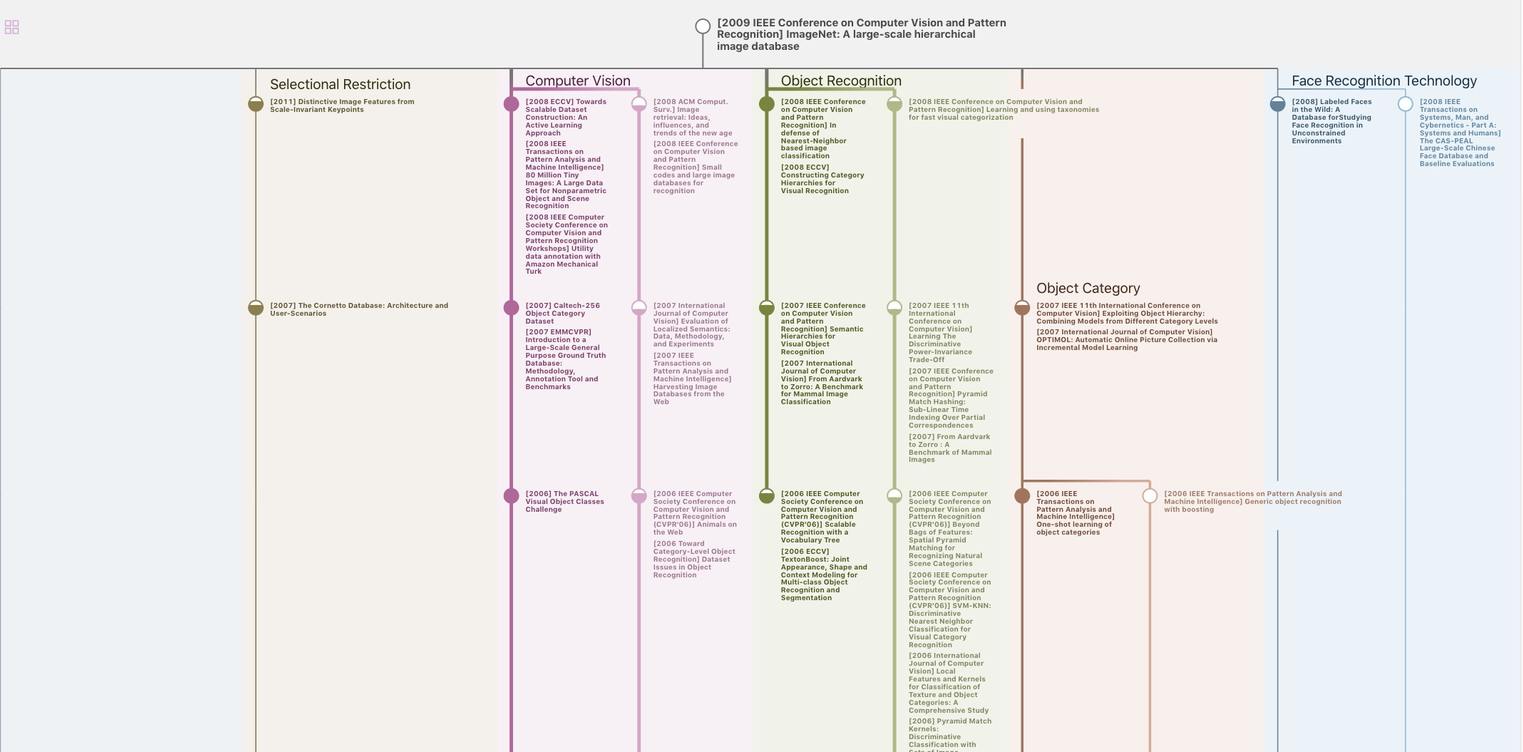
生成溯源树,研究论文发展脉络
Chat Paper
正在生成论文摘要