Deep learning segmentation and registration-driven lung parenchymal volume and movement CT analysis in prone positioning
PLOS ONE(2024)
摘要
Purpose To conduct a volumetric and movement analysis of lung parenchyma in prone positioning using deep neural networks (DNNs). Method We included patients with suspected interstitial lung abnormalities or disease who underwent full-inspiratory supine and prone chest CT at a single institution between June 2021 and March 2022. A thoracic radiologist visually assessed the fibrosis extent in the total lung (using units of 10%) on supine CT. After preprocessing the images into 192x192x192 resolution, a DNN automatically segmented the whole lung and pulmonary lobes in prone and supine CT images. Affine registration matched the patient's center and location, and the DNN deformably registered prone and supine CT images to calculate the x-, y-, z-axis, and 3D pixel movements. Results In total, 108 CT pairs had successful registration. Prone positioning significantly increased the left lower (90.2 +/- 69.5 mL, P = 0.000) and right lower lobar volumes (52.5 +/- 74.2 mL, P = 0.000). During deformable registration, the average maximum whole-lung pixel movements between the two positions were 1.5, 1.9, 1.6, and 2.8 cm in each axis and 3D plane. Compared to patients with <30% fibrosis, those with >= 30% fibrosis had smaller volume changes (P<0.001) and smaller pixel movements in all axes between the positions (P = 0.000-0.007). Forced vital capacity (FVC) correlated with the left lower lobar volume increase (Spearman correlation coefficient, 0.238) and the maximum whole-lung pixel movements in all axes (coefficients, 0.311 to 0.357). Conclusions Prone positioning led to the preferential expansion of the lower lobes, correlated with FVC, and lung fibrosis limited lung expansion during prone positioning.
更多查看译文
AI 理解论文
溯源树
样例
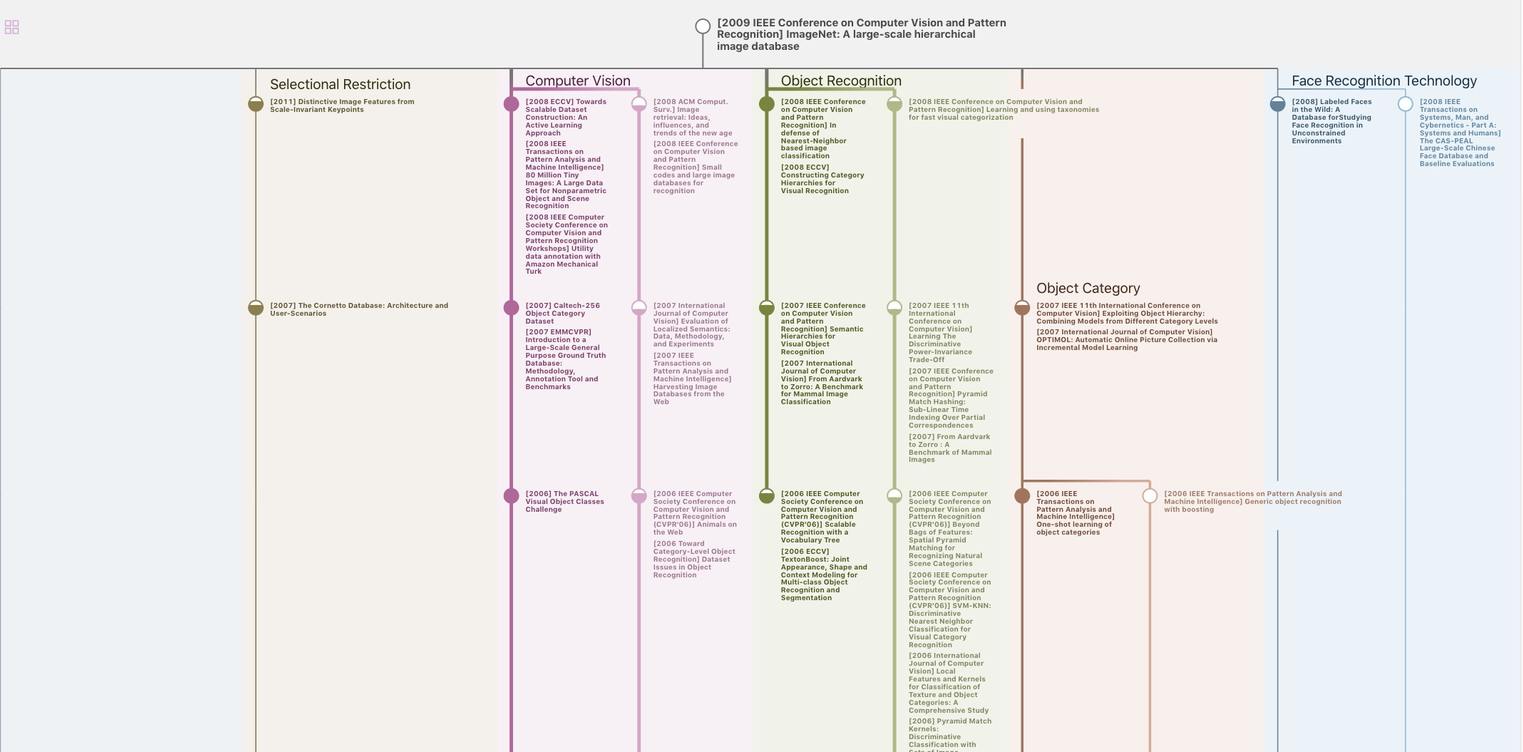
生成溯源树,研究论文发展脉络
Chat Paper
正在生成论文摘要