MolFeSCue: Enhancing Molecular Property Prediction in Data-Limited and Imbalanced Contexts Using Few-Shot and Contrastive Learning.
Bioinformatics (Oxford, England)(2024)
摘要
Motivation Predicting molecular properties is a pivotal task in various scientific domains, including drug discovery, material science, and computational chemistry. This problem is often hindered by the lack of annotated data and imbalanced class distributions, which pose significant challenges in developing accurate and robust predictive models.Results This study tackles these issues by employing pretrained molecular models within a few-shot learning framework. A novel dynamic contrastive loss function is utilized to further improve model performance in the situation of class imbalance. The proposed MolFeSCue framework not only facilitates rapid generalization from minimal samples, but also employs a contrastive loss function to extract meaningful molecular representations from imbalanced datasets. Extensive evaluations and comparisons of MolFeSCue and state-of-the-art algorithms have been conducted on multiple benchmark datasets, and the experimental data demonstrate our algorithm's effectiveness in molecular representations and its broad applicability across various pretrained models. Our findings underscore MolFeSCues potential to accelerate advancements in drug discovery.Availability and implementation We have made all the source code utilized in this study publicly accessible via GitHub at http://www.healthinformaticslab.org/supp/ or https://github.com/zhangruochi/MolFeSCue. The code (MolFeSCue-v1-00) is also available as the supplementary file of this paper.
更多查看译文
关键词
Molecular Dynamics
AI 理解论文
溯源树
样例
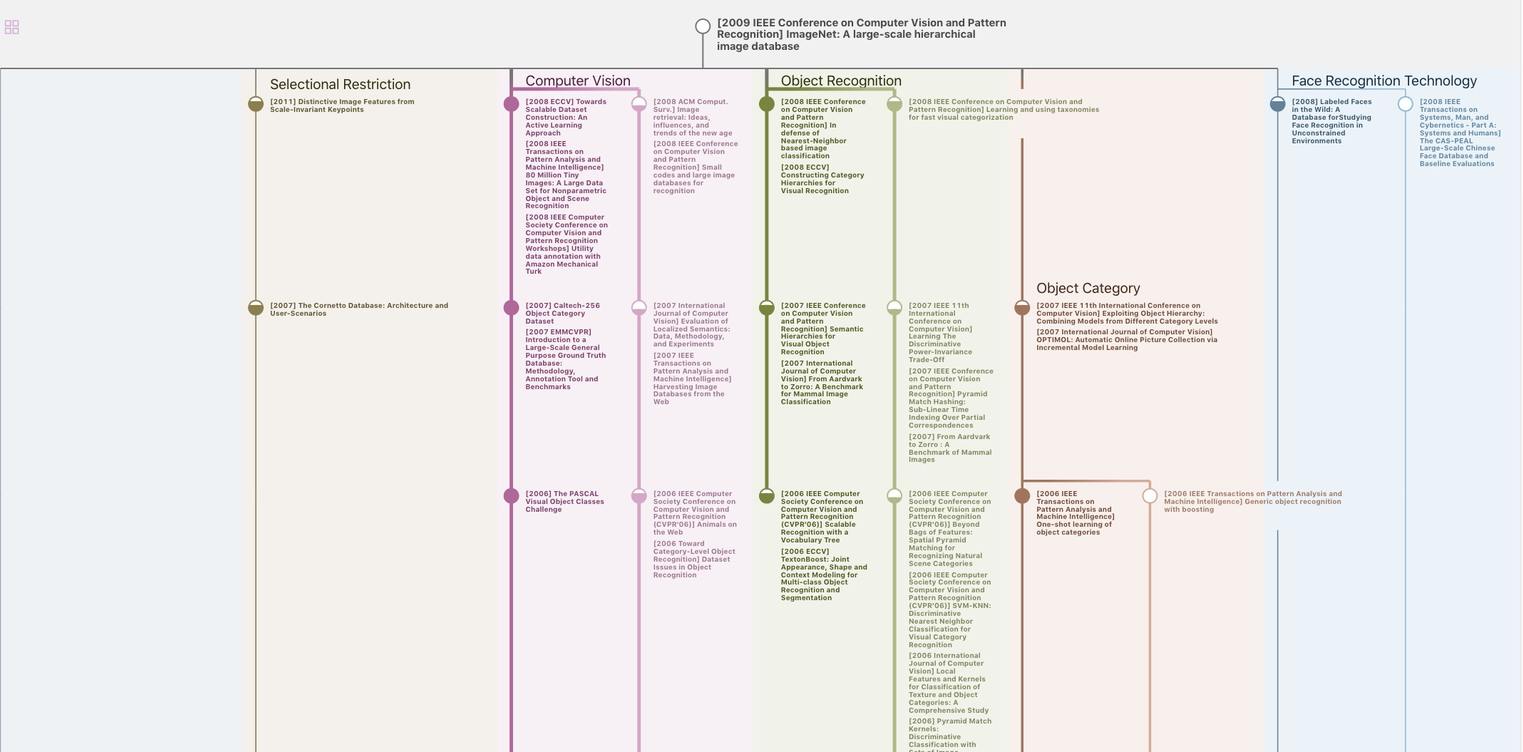
生成溯源树,研究论文发展脉络
Chat Paper
正在生成论文摘要