Category-level Object Pose Estimation in Heavily Cluttered Scenes by Generalized Two-stage Shape Reconstructor
IEEE access(2024)
摘要
In this paper, we propose a method for robust estimation of the pose of an unknown object instance in an object category from a depth image, even if it is occluded. In cluttered scenes, objects are often mutually occluded, and at the same time, objects in a category often have various shapes. For estimating the object pose in such situations, we have previously proposed a Two-stage Shape Reconstructor to extract features by de-occluding the occluded region of a target object and absorbing shape variations in a category. However, the model could not be used except in a situation where the unoccluded mask of the target object is known, and only if the contour of the occluding object is expected to have a linear shape. To cope with this problem, we generalize the previous model to directly extract the feature of a de-occluded object from a depth image containing the object occluded by another object. We also propose a data augmentation method for effectively training the model. Through evaluations on large-scale virtual-world and real-world datasets, we confirm that the proposed method achieves promising results on pose estimation of an unknown occluded object from an observed depth image.
更多查看译文
关键词
Feature extraction,Pose estimation,Image reconstruction,Training,Robot sensing systems,Data augmentation,Encoding,Object pose estimation,AutoEncoder,occlusion
AI 理解论文
溯源树
样例
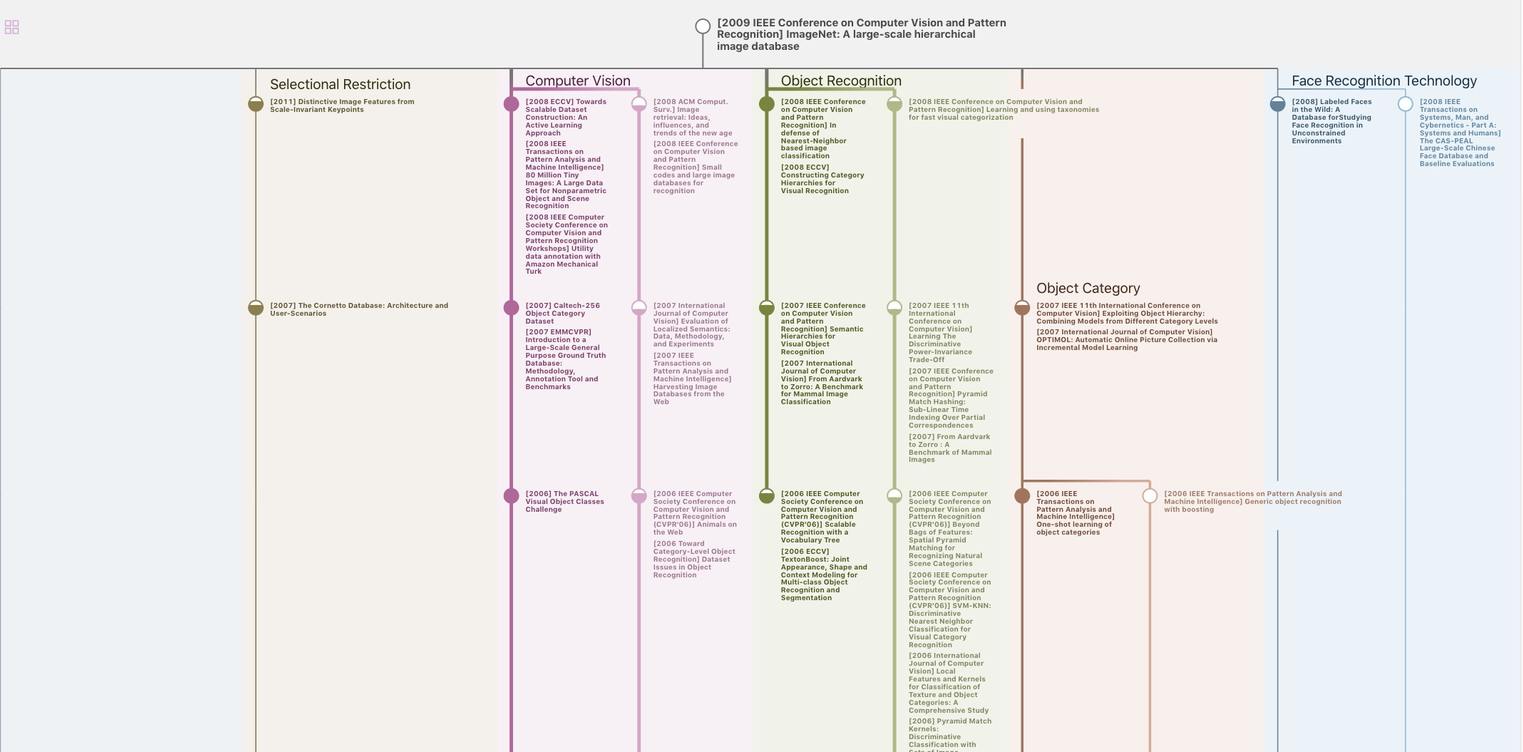
生成溯源树,研究论文发展脉络
Chat Paper
正在生成论文摘要