A Concept Drift Detection Method for Electricity Forecasting Based on Adaptive Window and Transformer
2023 IEEE Smart World Congress (SWC)(2023)
摘要
Electricity forecasting plays a vital role in power production and dispatch by predicting future electricity demand and market trends using historical power load, power market data, and relevant environmental factors. Currently, artificial intelligence methods have been widely employed in electricity prediction with considerable success. However, these methods often rely on offline prediction, making it challenging to adapt to the real-time dynamic changes in power data. If there is a change in the distribution of power data causing concept drift, the accuracy of offline prediction may significantly decrease. The current concept drift detection methods used in electricity prediction are not capable of detecting concept drift, identifying categories correctly, and capturing long-term dependencies effectively. To address this issue, we propose the Meta-ADPTF method, which utilizes Transformers as the prototypical network for embedding space neural networks and adaptive window meta metric learning for detecting concept drift. Our proposed approach significantly enhances accuracy and robustness compared to existing concept drift detection algorithms, according to the experimental results.
更多查看译文
关键词
concept drift,prototypical network,adaptive window,metric learning
AI 理解论文
溯源树
样例
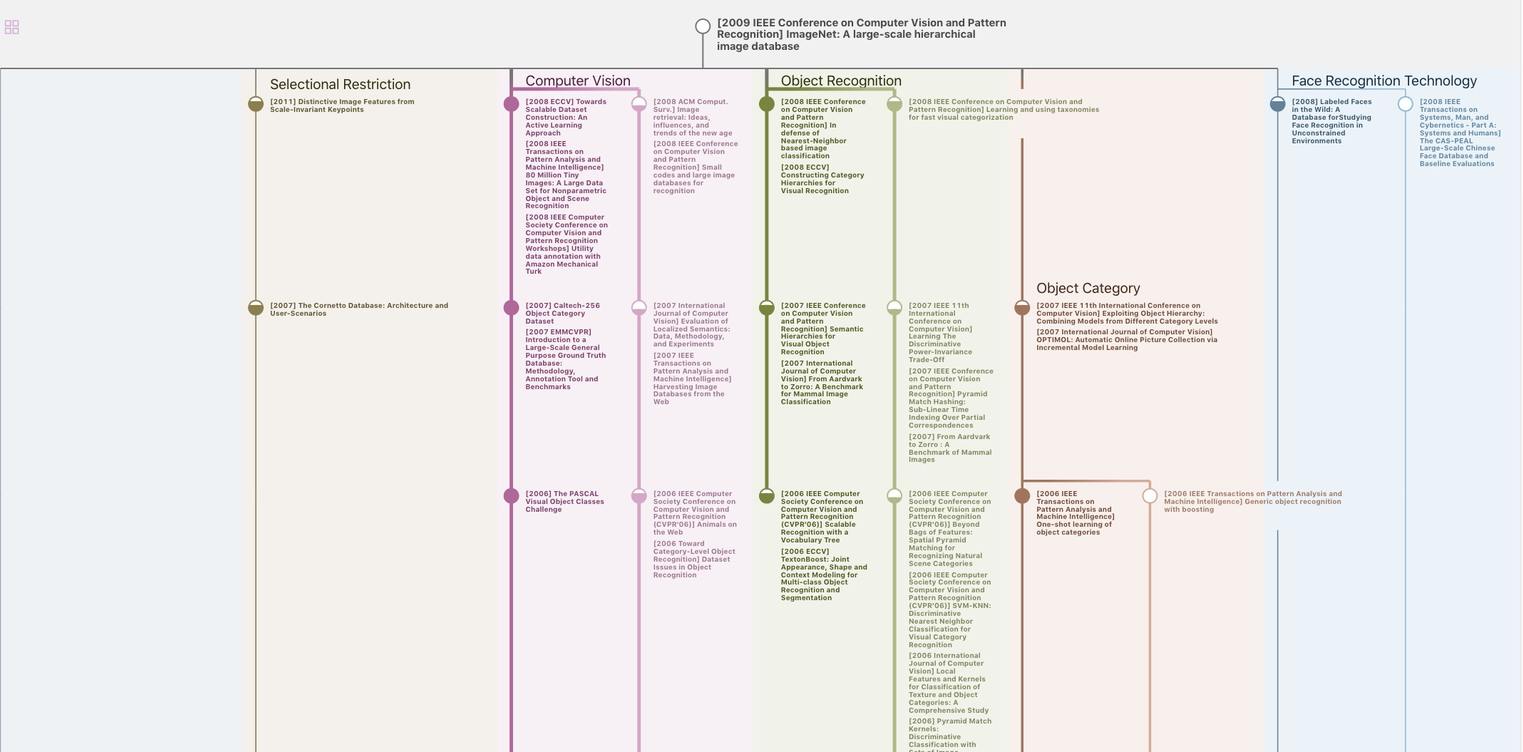
生成溯源树,研究论文发展脉络
Chat Paper
正在生成论文摘要