FingerSTR: Weak Supervised Transformer for Latent Fingerprint Segmentation.
International Joint Conference on Biometrics(2023)
摘要
Latent fingerprint segmentation is a crucial process in contemporary biometric systems utilized in criminal investigations and security applications. Accurately segmenting the fingerprint region from the background noise and artifacts, which can be challenging due to the complexity of the surrounding environment, is the primary goal of this process. Although various methodologies, including binarization-based, texture-based, and deep learning-based segmentation approaches have been proposed, they are often limited by environmental noise and a scarcity of annotated data, resulting in a low segmentation accuracy rate. In this paper, we propose FingerSTR (Finger Segmentation Transformer), a fully Transformer-based latent fingerprint segmentation network, and introduce a new teacher-student training methodology to achieve more precise and robust segmentation results without requiring manual annotation. Based on experimental results of latent fingerprint database NIST SD27, FingerSTR surpasses both deep-learning algorithms and handcraft methods, achieving state-of-the-art performance in the latent fingerprint segmentation task.
更多查看译文
关键词
Latent Fingerprints,Deep Learning,Low Accuracy,Segmentation Results,Segmentation Accuracy,Manual Annotation,Environmental Noise,Fingerprint Region,Criminal Investigations,Convolutional Network,Convolutional Neural Network,Multiple Layers,Reliable Prediction,Teacher Model,Intersection Over Union,Multilayer Perceptron,Training Stage,Semantic Segmentation,Student Model,Teacher Network,Weak Labels,Manual Labeling,Segmentation Labels,Student Network,Unlabeled Examples,Consistency Loss,Inductive Bias,Crime Scene,Class Activation Maps,Impact Of Labels
AI 理解论文
溯源树
样例
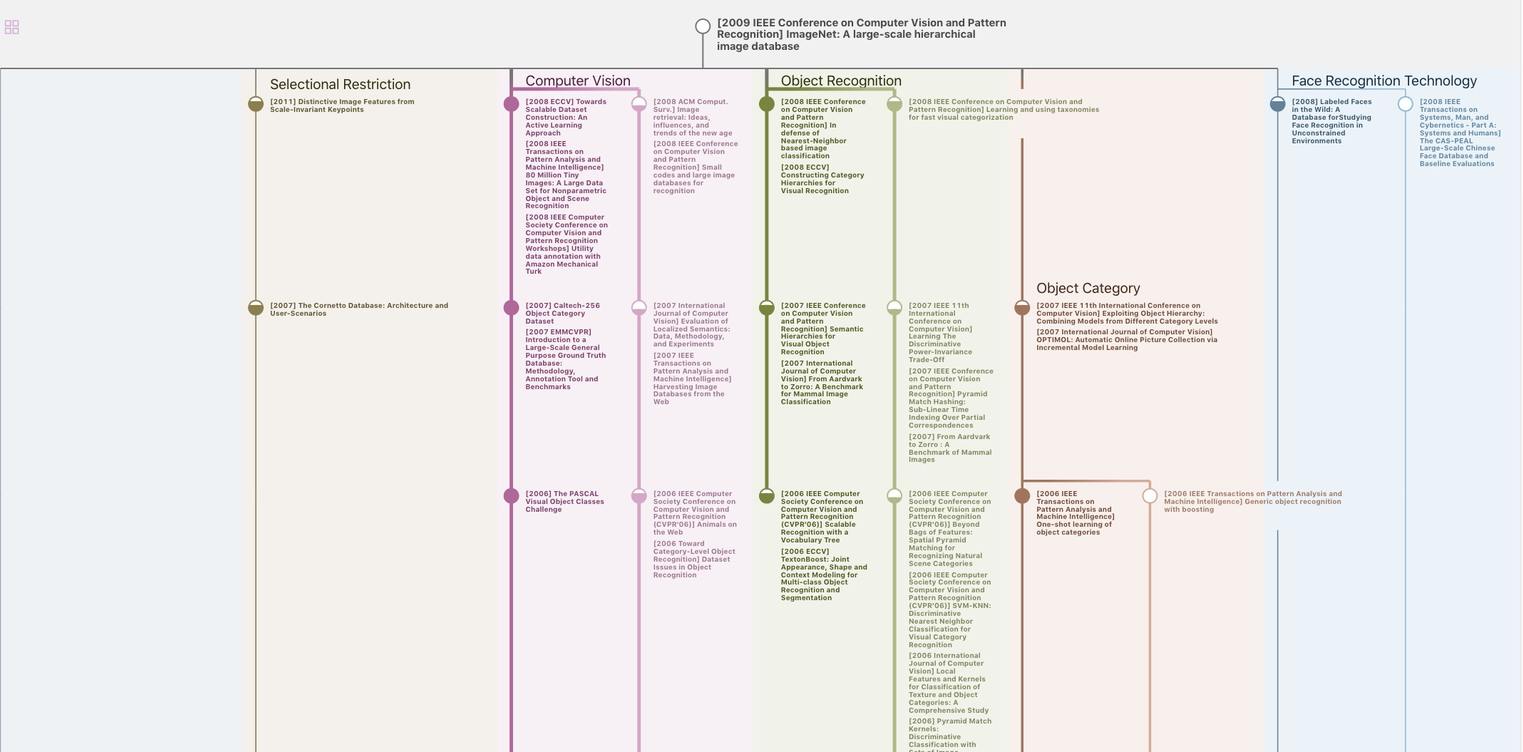
生成溯源树,研究论文发展脉络
Chat Paper
正在生成论文摘要