Physics-informed Machine Learning for Low-Cycle Fatigue Life Prediction of 316 Stainless Steels
International journal of fatigue(2024)
摘要
Degraded fatigue performance has been frequently reported for metallic materials at elevated temperatures, which is further deteriorated by reducing the loading frequency due to creep deformation. The evaluation of fatigue life for materials and structures typically relies on a large number of experiments and simulations, which is time-consuming and expensive. To address these challenges, a novel physical informed-based multi-layer nested neural network framework is proposed in this work to predict the low-cycle fatigue life of 316 stainless steels at different temperatures and strain rates. The proposed framework integrates physical constraints into a multi-layer nested neural network. Introducing physical constraints into the neural network can enhance the prediction accuracy of the machine learning approach. All predicted fatigue lives fall within the twice error band, with the majority of them falling within the 1.5 times error band. This approach has the potential to significantly improve computational efficiency and reduce resource consumption in the fatigue life prediction of materials.
更多查看译文
关键词
316 stainless steels,Fatigue life prediction,Machine learning,Physical constraints,Neural network
AI 理解论文
溯源树
样例
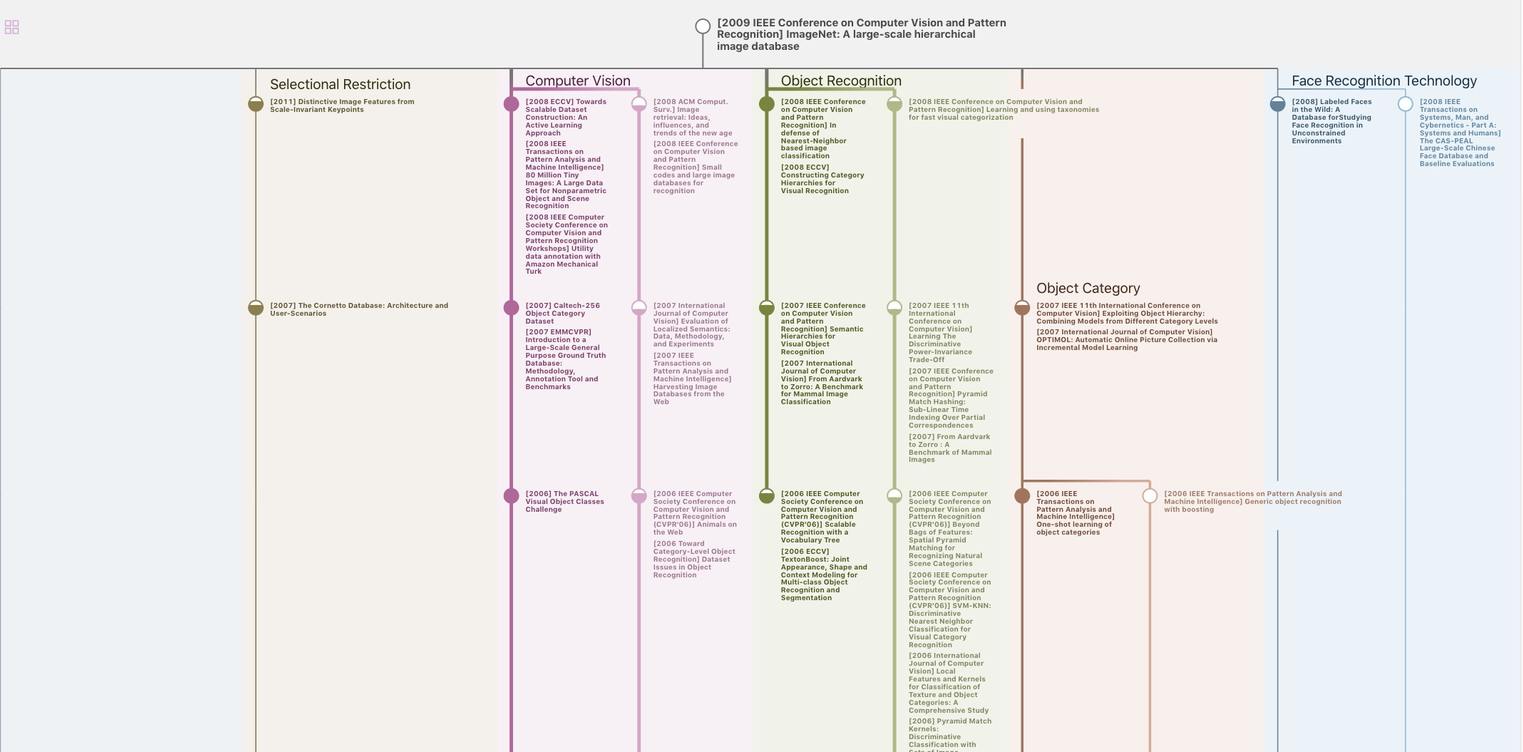
生成溯源树,研究论文发展脉络
Chat Paper
正在生成论文摘要