SBS feature selection and AdaBoost classifier for specialization/major recommendation for undergraduate students
Education and Information Technologies(2024)
摘要
Selecting undergraduate majors or specializations is a crucial decision for students since it considerably impacts their educational and career paths. Moreover, their decisions should match their academic background, interests, and goals to pursue their passions and discover various career paths with motivation. However, such a decision remains challenging are unfamiliar with the job market, the demand for the required skills, and being in the proper placement in a major is not straightforward. Thus, an automatic recommendation system can be helpful for students to assist and guide them in the right decision. In this context, we developed a machine learning model to predict and recommend suitable specializations for undergraduate students according to the job market and student’s academic history. Two hundred twenty-five records of students are considered to establish this work. The proposed approach encompasses four major steps, including data preprocessing to clean, scale, and prepare the data for training to avoid obtaining suboptimal results, accompanied by an oversampling process to equal the samples’ distribution to prevent the model from being biased or poorly generalized. Furthermore, we conducted a feature selection step using Sequential Backward Selection (SBS) to extract the relevant features to improve the outcomes and reduce the risk of noise. The selected subset is used to train the model using the AdaBoost classifier. We deployed the Genetic algorithm to optimize the classifier’s hyperparameters to maximize results. As a result, the findings of this study exhibit noticeable results compared to existing models, with an accuracy of 98.1
更多查看译文
关键词
Specialization/major,Recommender systems,Machine learning,Feature selection,Genetic algorithm,Hyperparameters optimization
AI 理解论文
溯源树
样例
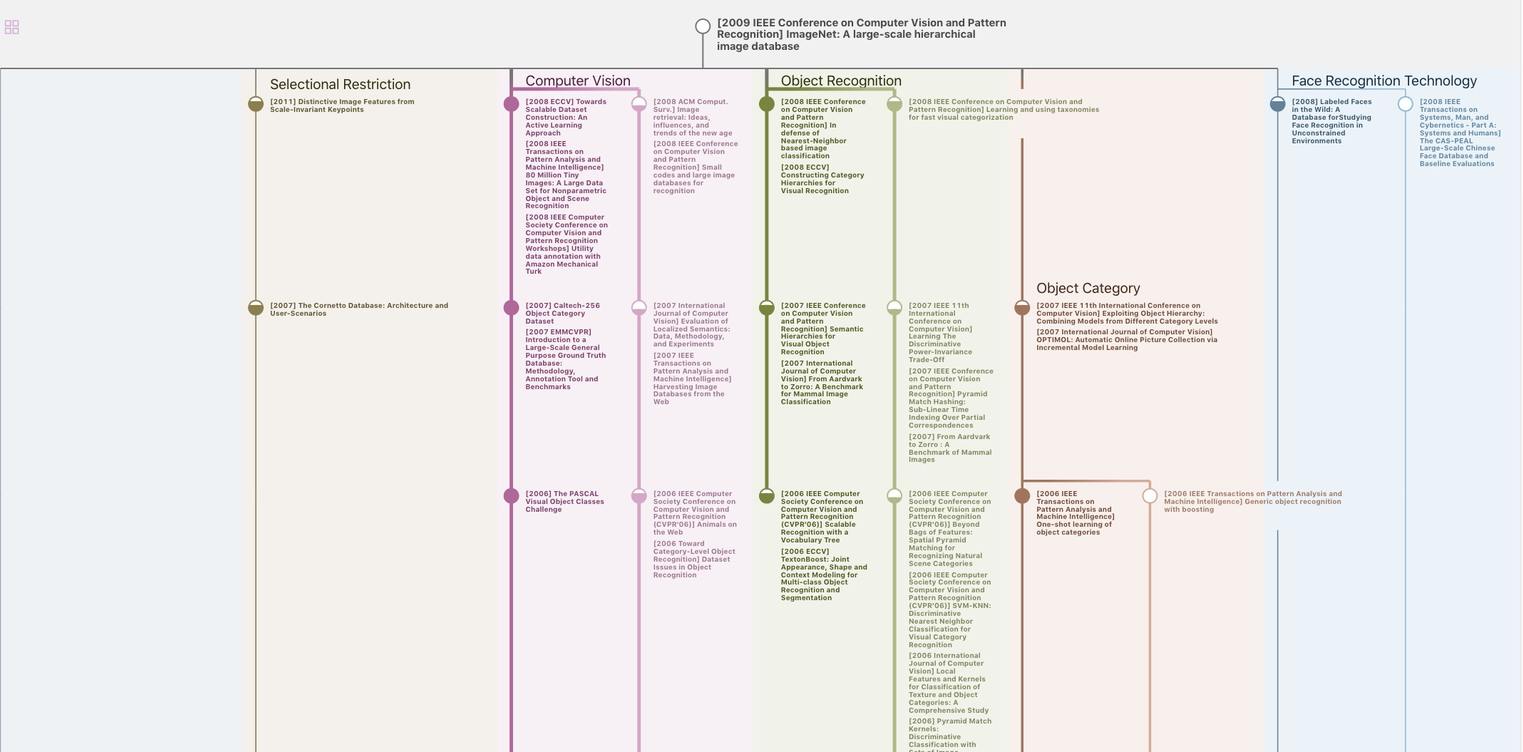
生成溯源树,研究论文发展脉络
Chat Paper
正在生成论文摘要