Semi-supervised medical image classification based on class prototype matching for soft pseudo labels with consistent regularization
Multimedia Tools and Applications(2024)
摘要
Due to the potentially high cost and difficulty of obtaining labeled data in medical image classification, semi-supervised learning can effectively utilize limited labeled data and large amounts of unlabeled data to enhance the performance of classification models. However, most existing methods utilize pseudo-labeling techniques that filter out a large amount of data, which makes the unlabeled data underutilized. Moreover, the consistency regularization methods rely heavily on the perturbation function suffering from a lack of generalization ability. In this paper, we propose a semi-supervised medical image classification approach based on class prototype matching for soft pseudo-labels with consistency regularization. A class prototype matching module is used to predict soft pseudo-labels for unlabeled data, and the quality of the predicted pseudo-labels is improved by a meticulously designed cache queue with dynamic and unbiased updates. The linear mixture strategy is employed to handle both labeled and unlabeled data supplying the model with more complex data inputs, which boosts the ability of the model to learn intra- and inter-class features. Extensive experiments on ISIC2018 skin lesion dataset and the Chexpert chest disease dataset demonstrate that our approach has satisfactory performance and generalization ability on the medical image classification than other state-of-the-art semi-supervised methods.
更多查看译文
关键词
Semi-supervised deep learning,Medical image classification,Pseudo-label estimation,Consistency regularization
AI 理解论文
溯源树
样例
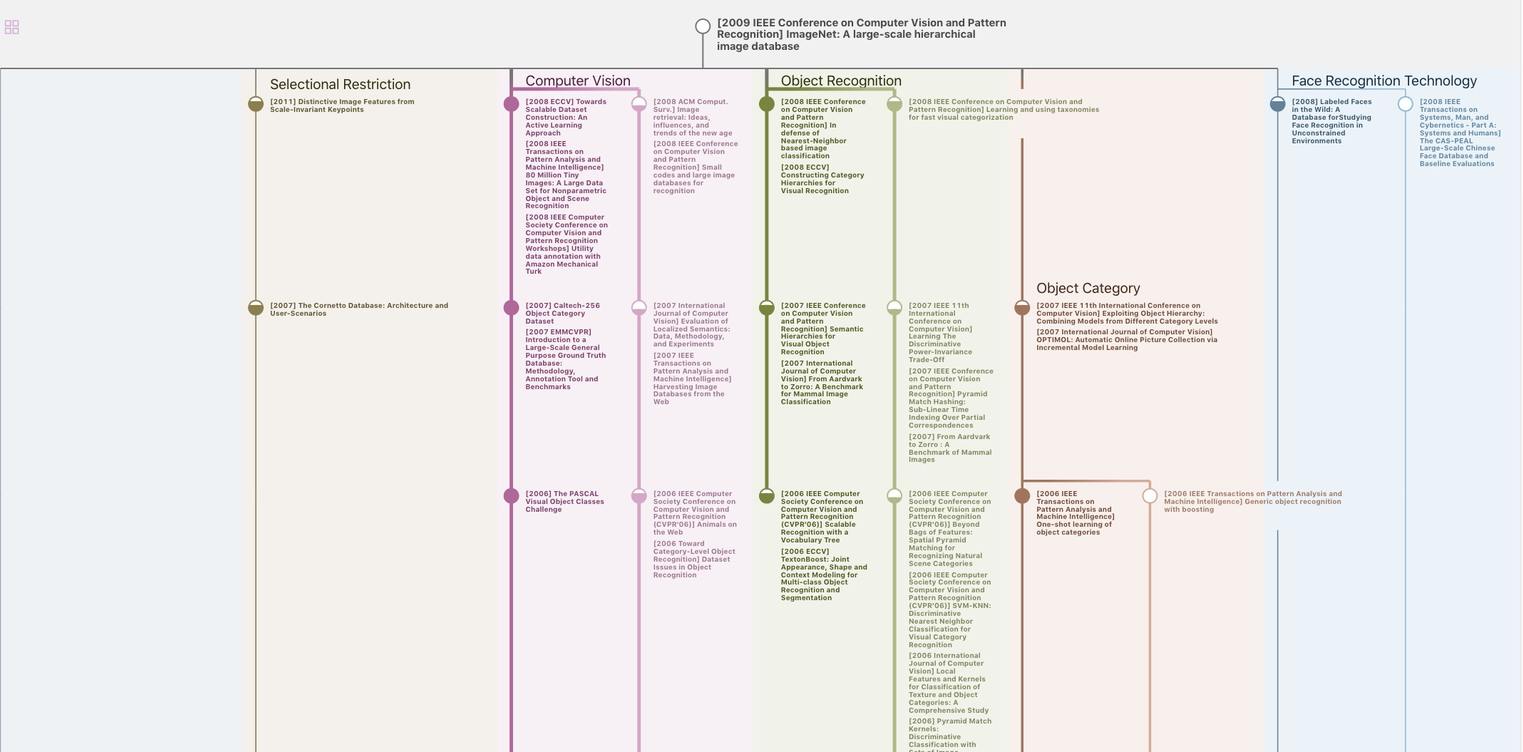
生成溯源树,研究论文发展脉络
Chat Paper
正在生成论文摘要