Mdpg: a novel multi-disease diagnosis prediction method based on patient knowledge graphs
Health Information Science and Systems(2024)
摘要
Diagnosis prediction, a key factor in enhancing healthcare efficiency, remains a focal point in clinical decision support research. However, the time-series, sparse and multi-noise characteristics of electronic health record (EHR) data make it a great challenge. Existing methods commonly address these issues using RNNs and incorporating medical prior knowledge from medical knowledge bases, but they neglect the local spatial characteristics and spatial–temporal correlation of the data. Consequently, we propose MDPG, a diagnosis prediction model based on patient knowledge graphs. Initially, we represent the electronic visit records of patients as a patient-centered temporal knowledge graph, capturing the local spatial structure and temporal characteristics of the visit information. Subsequently, we design the spatial graph convolution block, temporal self-attention block, and spatial–temporal synchronous graph convolution block to capture the spatial, temporal, and spatial–temporal correlations embedded in them, respectively. Ultimately, we accomplish the prediction of patients’ future states through multi-label classification. We conduct comprehensive experiments on two real-world datasets independently and evaluate the results using visit-level precision@k and code-level accuracy@k metrics. The experimental results demonstrate that MDPG outperforms all baseline models, yielding the best performance.
更多查看译文
关键词
Medical knowledge graphs,Patient knowledge graphs,Healthcare representation learning,Diagnosis prediction,Patient risk prediction
AI 理解论文
溯源树
样例
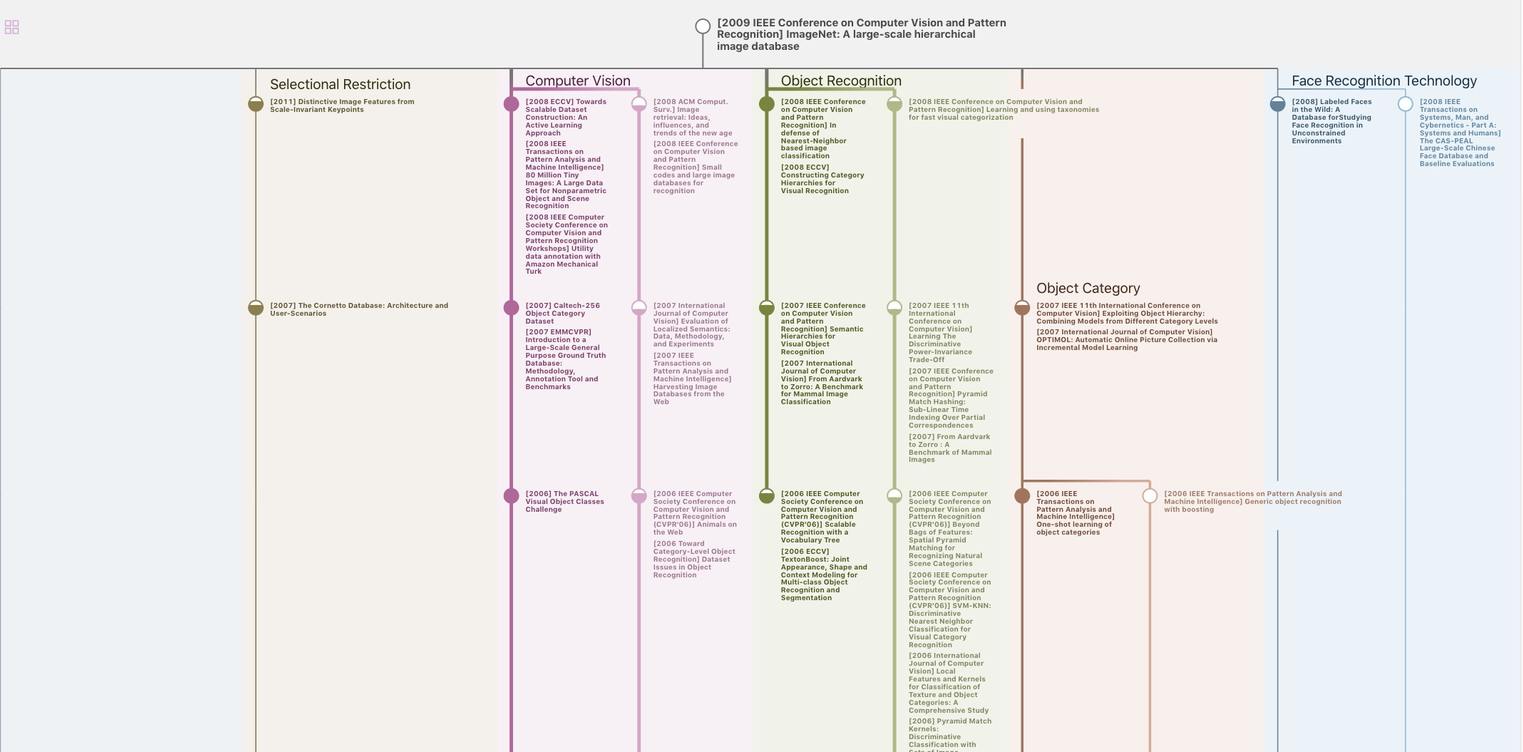
生成溯源树,研究论文发展脉络
Chat Paper
正在生成论文摘要