PROC2PDDL: Open-Domain Planning Representations from Texts
arxiv(2024)
摘要
Planning in a text-based environment continues to be a major challenge for AI
systems. Recent approaches have used language models to predict a planning
domain definition (e.g., PDDL) but have only been evaluated in closed-domain
simulated environments. To address this, we present Proc2PDDL , the first
dataset containing open-domain procedural texts paired with expert-annotated
PDDL representations. Using this dataset, we evaluate state-of-the-art models
on defining the preconditions and effects of actions. We show that Proc2PDDL is
highly challenging, with GPT-3.5's success rate close to 0
35
deficiency in both generating domain-specific prgorams and reasoning about
events. We hope this analysis and dataset helps future progress towards
integrating the best of LMs and formal planning.
更多查看译文
AI 理解论文
溯源树
样例
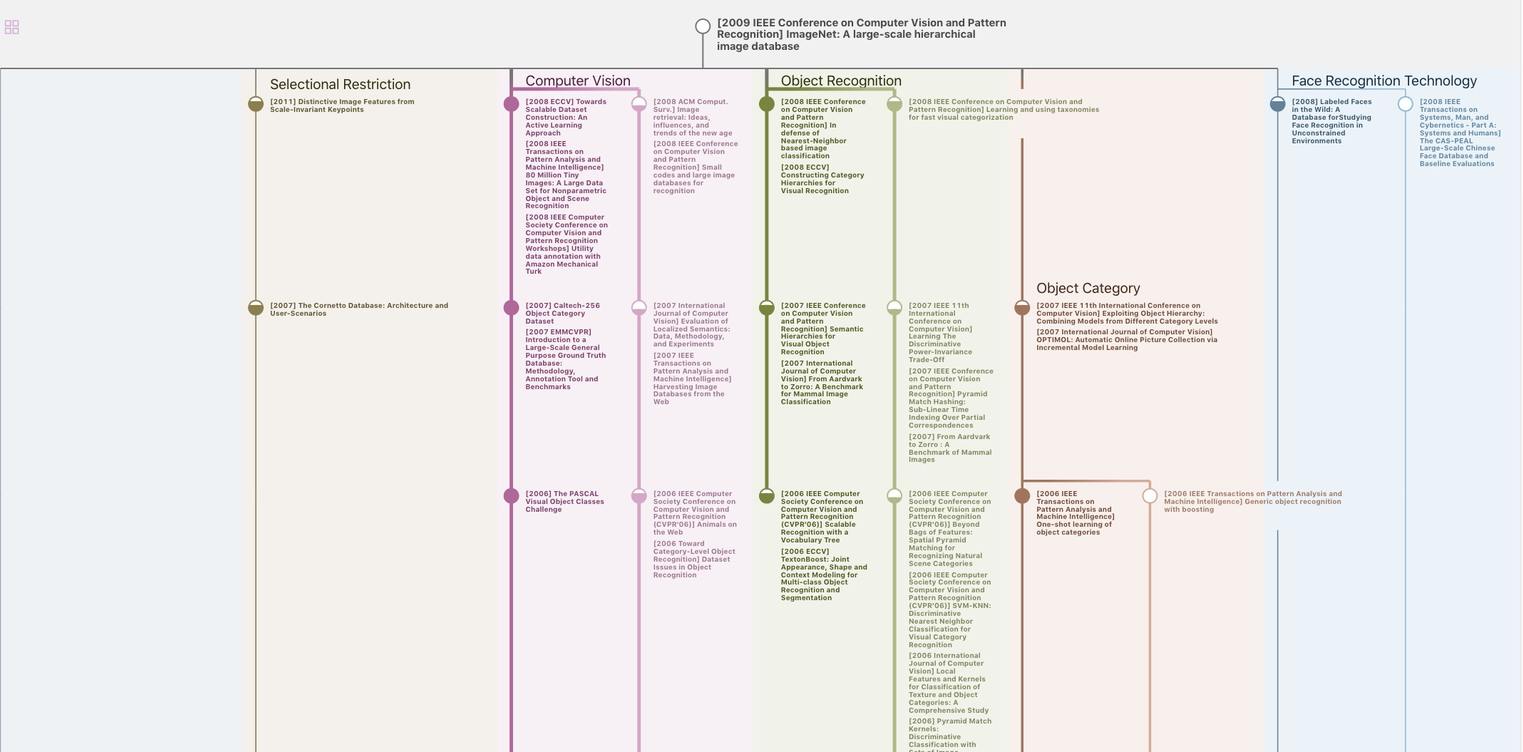
生成溯源树,研究论文发展脉络
Chat Paper
正在生成论文摘要