Geometry-free renormalization of directed networks: scale-invariance and reciprocity
arxiv(2024)
摘要
Recent research has tried to extend the concept of renormalization, which is
naturally defined for geometric objects, to more general networks with
arbitrary topology. The current attempts do not naturally apply to directed
networks, for instance because they are based on the identification of
(necessarily symmetric) inter-node distances arising from geometric embeddings
or on the definition of Hermitian Laplacian operators requiring symmetric
adjacency matrices in spectral approaches. Here we show that the
Scale-Invariant Model, recently proposed as an approach to consistently model
undirected networks at arbitrary (and possibly multi-scale) resolution levels,
can be extended coherently to directed networks without the requirement of an
embedding geometry or Laplacian structure. Moreover, it can account for
nontrivial reciprocity, i.e. the empirically well-documented tendency of links
to occur in mutual pairs more or less often than predicted by chance. After
deriving the renormalization rules for networks with arbitrary reciprocity, we
consider various examples. In particular we propose the first multiscale model
of the international trade network with nontrivial reciprocity and an annealed
model where positive reciprocity emerges spontaneously from coarse-graining. In
the latter case, the renormalization process defines a group, not only a
semigroup, and therefore allows to fine-grain networks to arbitrarily small
scales. These results strengthen the notion that network renormalization can be
defined in a much more general way than required by geometric or spectral
approaches, because it needs only node-specific (metric-free) features and can
coexist with the asymmetry of directed networks.
更多查看译文
AI 理解论文
溯源树
样例
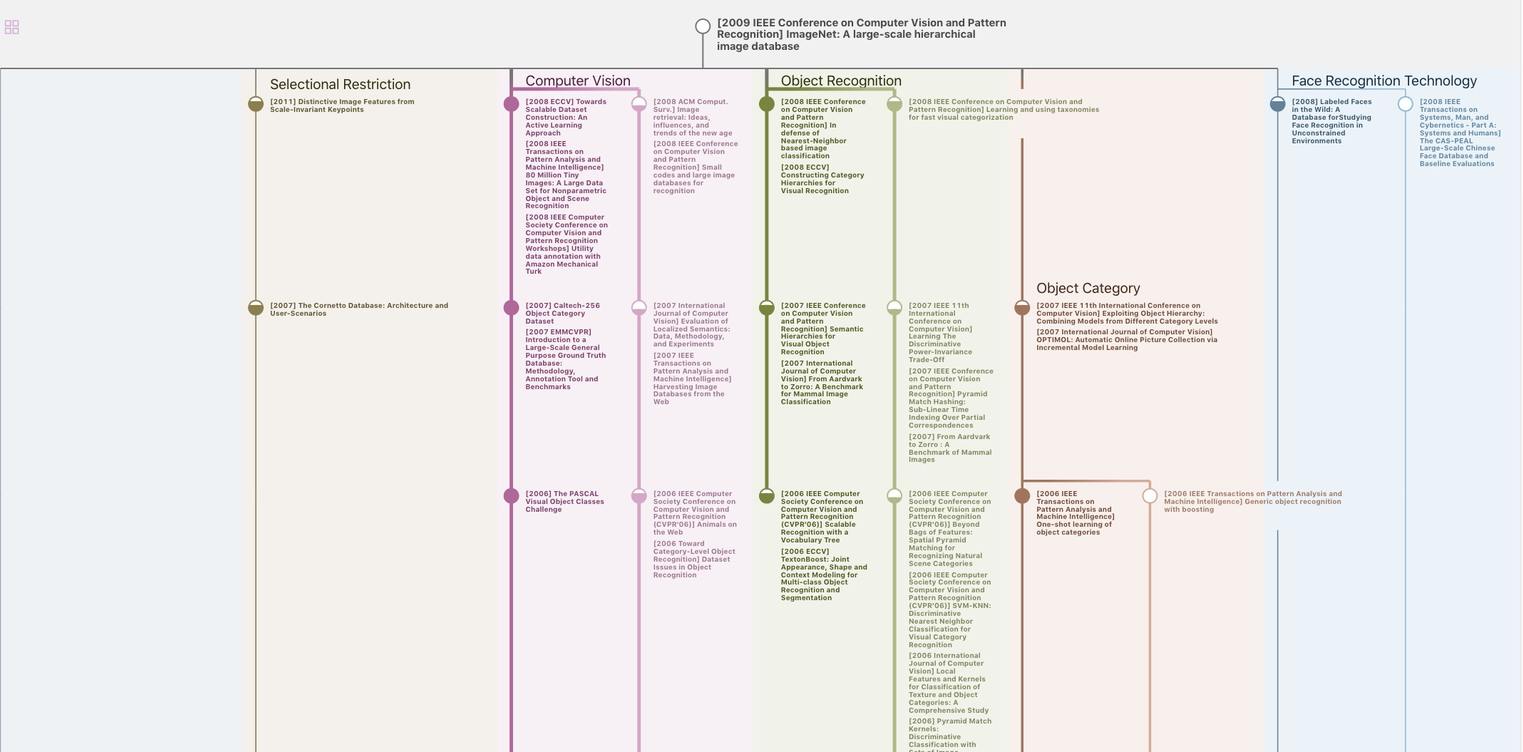
生成溯源树,研究论文发展脉络
Chat Paper
正在生成论文摘要