Empowering Digital Twin: Early Action Decision through GAN-enhanced Predictive Frame Synthesis for Autonomous Vehicles
2023 IEEE/ACM SYMPOSIUM ON EDGE COMPUTING, SEC 2023(2023)
摘要
Safety concerns surrounding autonomous vehicles (AVs) present significant barriers to their widespread adoption. In AVs, it is necessary to make speedy decisions to safely roam through complex dynamic environments. Interestingly, predicting the future environment can aid in making these early decisions from the partially observed data. This proactive approach is becoming increasingly vital as the number of vehicles on the roads continues to rise, necessitating advanced development strategies for AVs. In this scenario, simulations based on Digital Twin (DT) are proving to be effective in necessary computation during the development and inference phase of the AVs. In fact, the DT system can play a crucial role in making early decisions. Thus, in this research, we propose a Generative Adversarial Network (GAN) enhanced frame prediction system for single and multi-time ahead image forecasting to aid in environment prediction and early decision. We demonstrate the relative motion of entities within the frames and evaluate our system's efficacy through qualitative and quantitative analyses. As This GAN-enhanced system-inside a DT-can predict a few frames into the future and check for anomalies, this can help the AVs make swift decisions. Moreover, by harnessing this capability to create diverse synthetic scenarios, we can enhance the development of the DT system, thus unlocking a multitude of opportunities for training various models for autonomous vehicles (AVs).
更多查看译文
关键词
Autonomous Vehicles,Digital Twin,Frame Prediction,GAN,CNN,Image Generation
AI 理解论文
溯源树
样例
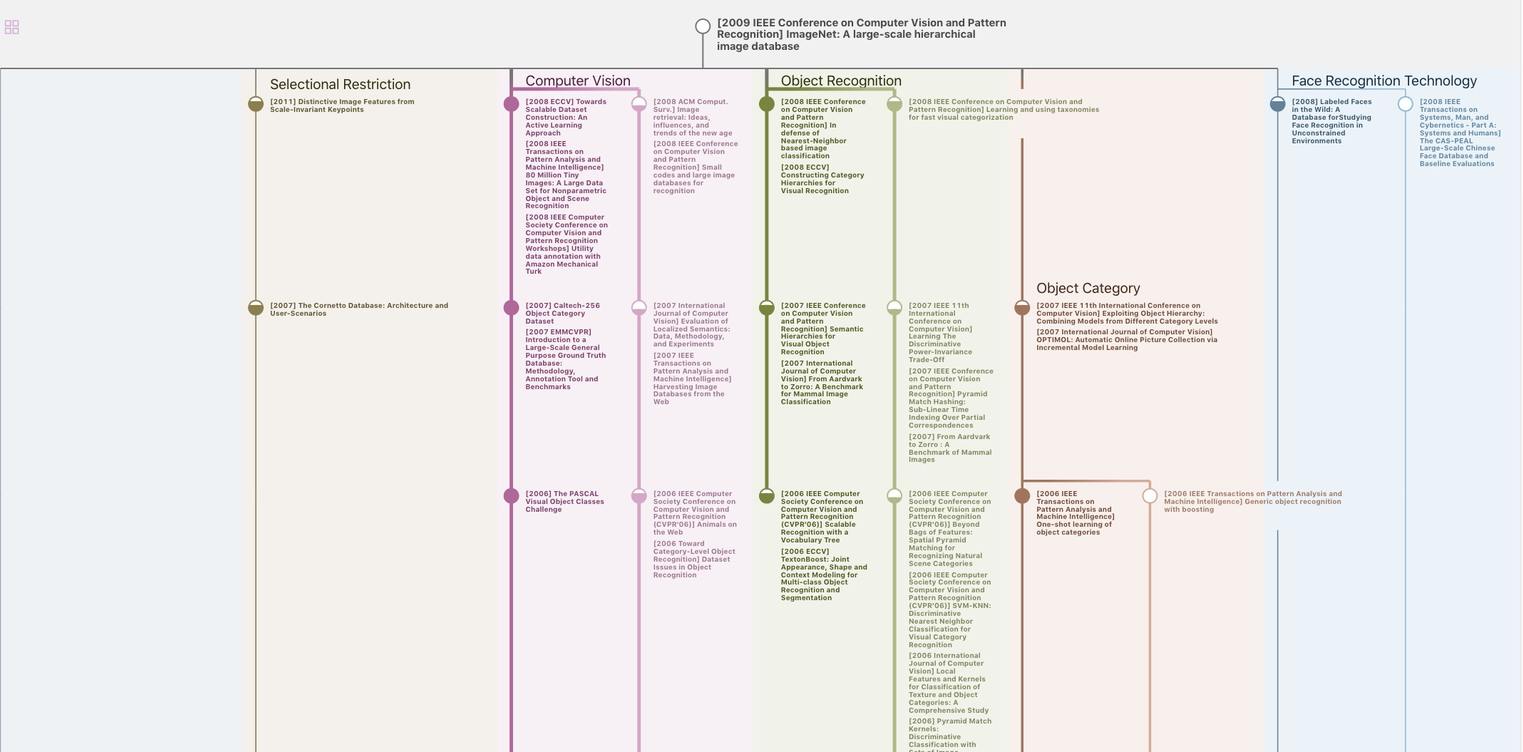
生成溯源树,研究论文发展脉络
Chat Paper
正在生成论文摘要