Machine learning that predicts well may not learn the correct physical descriptions of glassy systems
arxiv(2024)
摘要
The complexity of glasses makes it challenging to explain their dynamics.
Machine Learning (ML) has emerged as a promising pathway for understanding
glassy dynamics by linking their structural features to rearrangement dynamics.
Support Vector Machine (SVM) was one of the first methods used to detect such
correlations. Specifically, a certain output of SVMs trained to predict
dynamics from structure, the distance from the separating hyperplane, was
interpreted as being linearly related to the activation energy for the
rearrangement. By numerical analysis of toy models, we explore under which
conditions it is possible to infer the energy barrier to rearrangements from
the distance to the separating hyperplane. We observe that such successful
inference is possible only under very restricted conditions. Typical tests,
such as the apparent Arrhenius dependence of the probability of rearrangement
on the inferred energy and the temperature, or high cross-validation accuracy
do not guarantee success. We propose practical approaches for measuring the
quality of the energy inference and for modifying the inferred model to improve
the inference, which should be usable in the context of realistic datasets.
更多查看译文
AI 理解论文
溯源树
样例
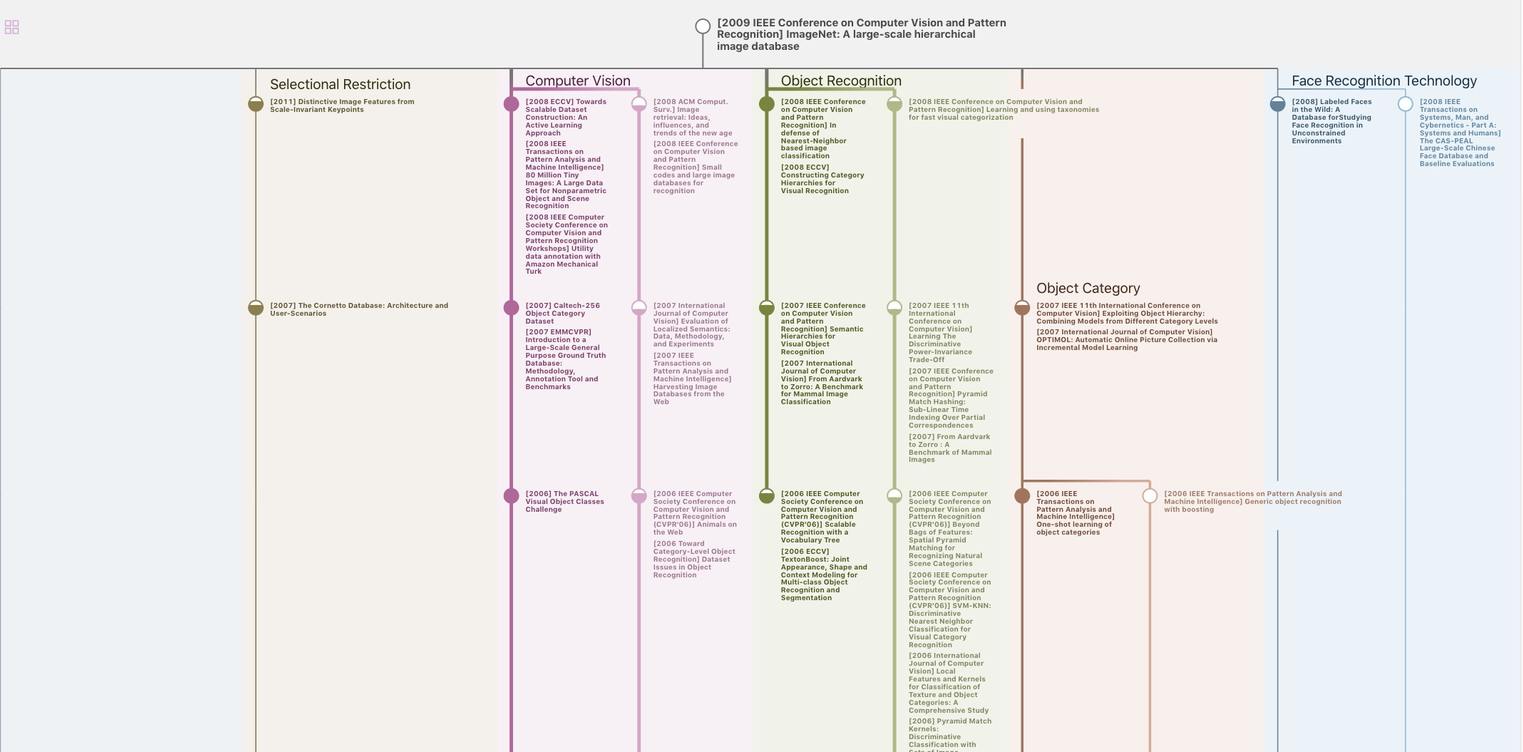
生成溯源树,研究论文发展脉络
Chat Paper
正在生成论文摘要