A fusion gas load prediction model with three-way residual error amendment
Energy(2024)
摘要
Accurately predicting gas load is crucial for optimal planning and scheduling of natural gas production. Existing machine learning or deep learning-based prediction methods primarily aim to improve accuracy. However, these methods face challenges such as overlapped features, significant background noise, and residual errors. In response to these challenges, we proposed a fusion prediction model, known as the Double Temporal Convolutional Network (TCN) and Light Gradient Boosting Machine (LightGBM) with Three-way Residual Error Amendment (DT-LGBM-3WREA). To address the overlapped feature issue in time series data, we utilize the STL module for data decomposition. To handle background noise, the network incorporates a double TCN module specifically designed for trend and seasonal components. Thus, it can better avoid the transmission of noise between modules. To address residual error, we introduce three-way decision methodology and develop the 3WREA module, which aims to avoid local optima and random factors from perturbing the prediction accuracy. This approach mitigates the influence of remainder item on overall prediction performance. Experimental results demonstrate that the DT-LGBM-3WREA model excels in gas load prediction scenarios, surpassing state-of-the-art methods.
更多查看译文
关键词
Gas load prediction,Residual error,Three-way decision,Temporal convolutional network
AI 理解论文
溯源树
样例
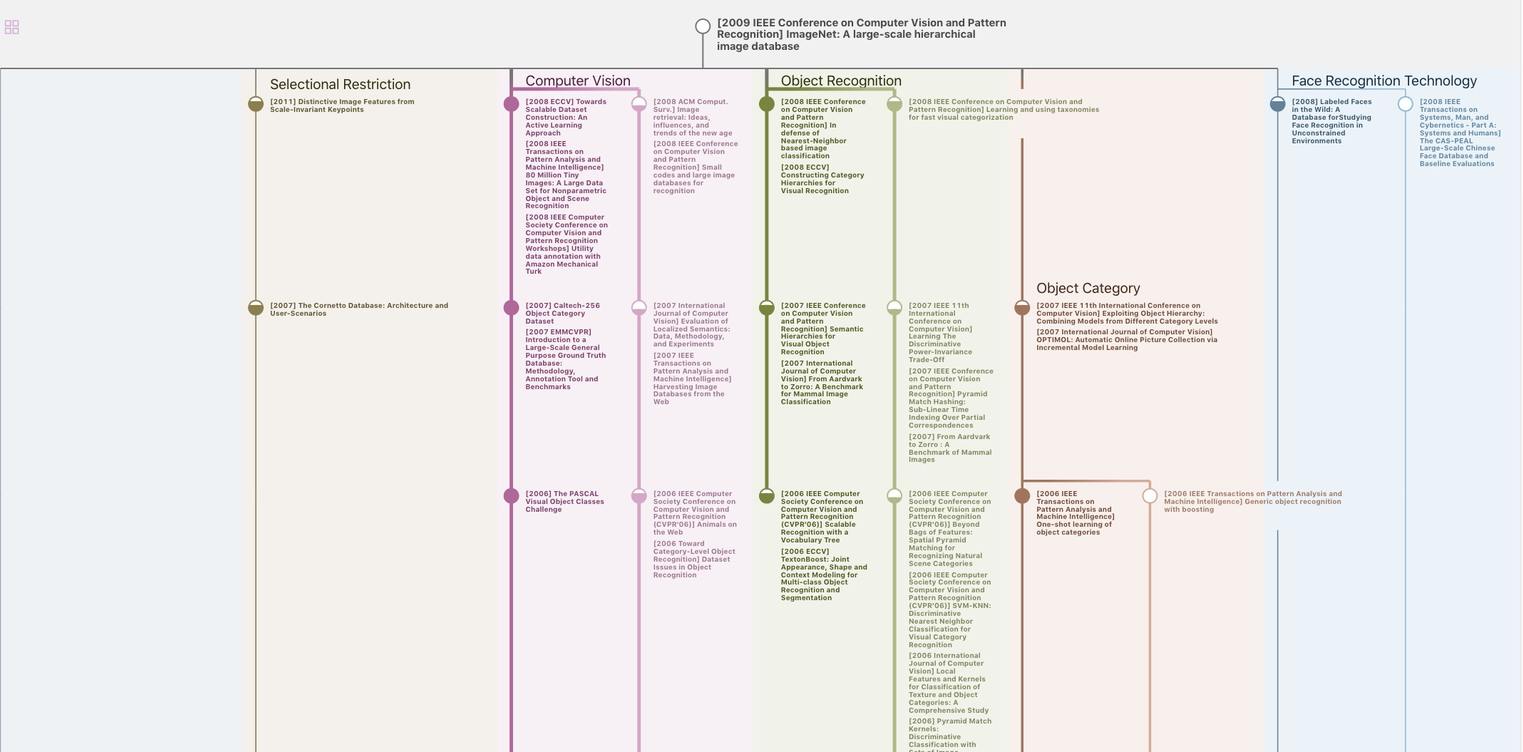
生成溯源树,研究论文发展脉络
Chat Paper
正在生成论文摘要