Incremental online non-parametric modeling of surface vehicle dynamics using adaptive spectral metric Gaussian processes learning
OCEAN ENGINEERING(2024)
摘要
High -precision online modeling is the basis for various marine control and navigation of surface vehicles. An incremental Gaussian processes learning based on subspace index and adaptive spectral metric kernels is proposed for incremental online non -parametric identification of surface vehicle dynamics. The scheme utilizes streaming data for online identification without special designs of training data and can consider environmental interference. The subspaces are updated online by similarity, so the proposed subspace index can be viewed as a sparse technique for streaming online identification data. In order to improve the online model accuracy, an adaptive version of spectral metric kernels is designed in parallel utilizing novel adaptive moment estimation. The feasibility of the proposed scheme is verified through random steering tests of a 4 -degree -of -freedom surface vehicle. Compared with traditional non -parametric identification methods, the proposed online identification scheme is effective in prediction accuracy and model generalization. The results show that this online scheme can provide a model basis for intelligent surface vehicle automation.
更多查看译文
关键词
Incremental online non-parametric modeling,Ship dynamics model,Incremental Gaussian processes learning,Spectral metric kernel,Subspace index
AI 理解论文
溯源树
样例
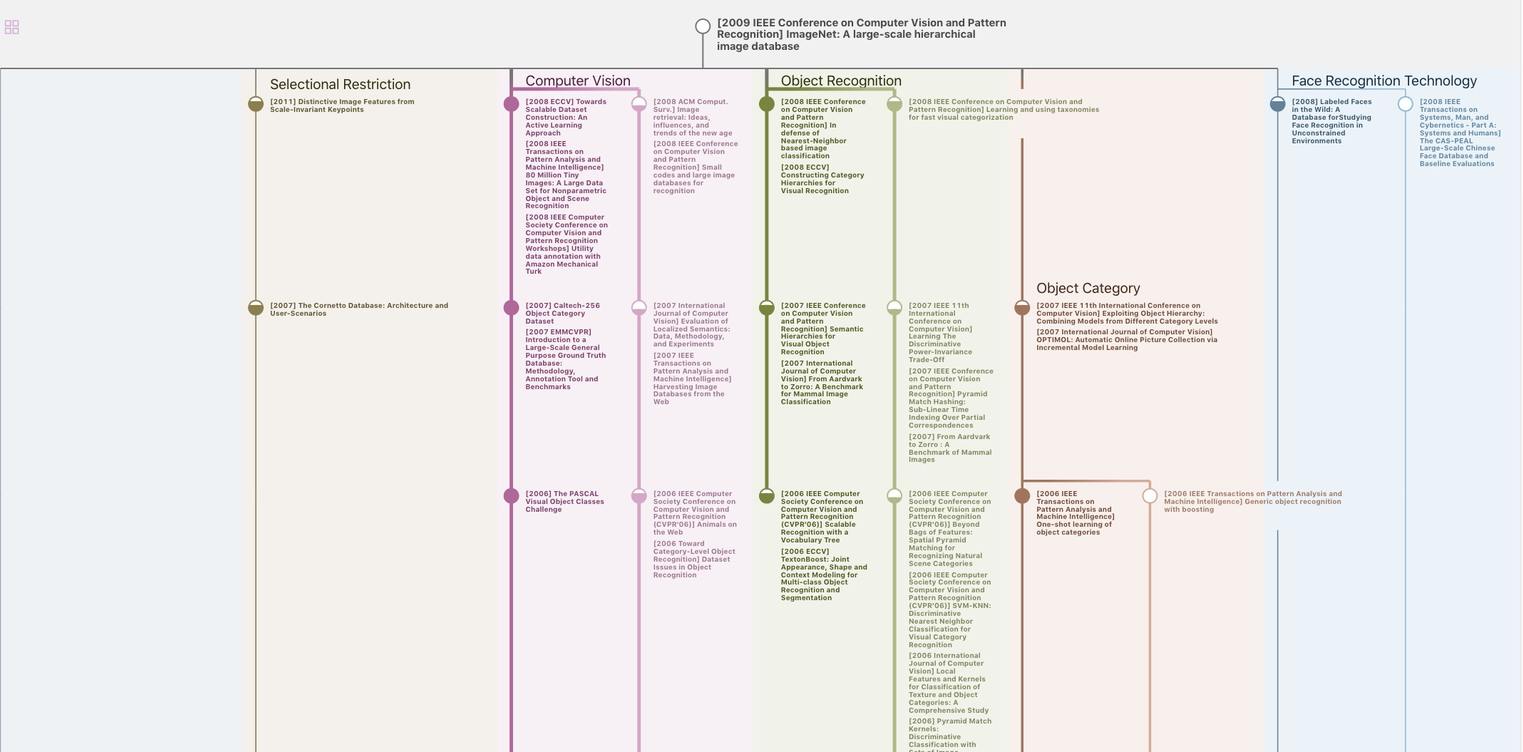
生成溯源树,研究论文发展脉络
Chat Paper
正在生成论文摘要