GraphRCG: Self-conditioned Graph Generation via Bootstrapped Representations
arxiv(2024)
摘要
Graph generation generally aims to create new graphs that closely align with
a specific graph distribution. Existing works often implicitly capture this
distribution through the optimization of generators, potentially overlooking
the intricacies of the distribution itself. Furthermore, these approaches
generally neglect the insights offered by the learned distribution for graph
generation. In contrast, in this work, we propose a novel self-conditioned
graph generation framework designed to explicitly model graph distributions and
employ these distributions to guide the generation process. We first perform
self-conditioned modeling to capture the graph distributions by transforming
each graph sample into a low-dimensional representation and optimizing a
representation generator to create new representations reflective of the
learned distribution. Subsequently, we leverage these bootstrapped
representations as self-conditioned guidance for the generation process,
thereby facilitating the generation of graphs that more accurately reflect the
learned distributions. We conduct extensive experiments on generic and
molecular graph datasets across various fields. Our framework demonstrates
superior performance over existing state-of-the-art graph generation methods in
terms of graph quality and fidelity to training data.
更多查看译文
AI 理解论文
溯源树
样例
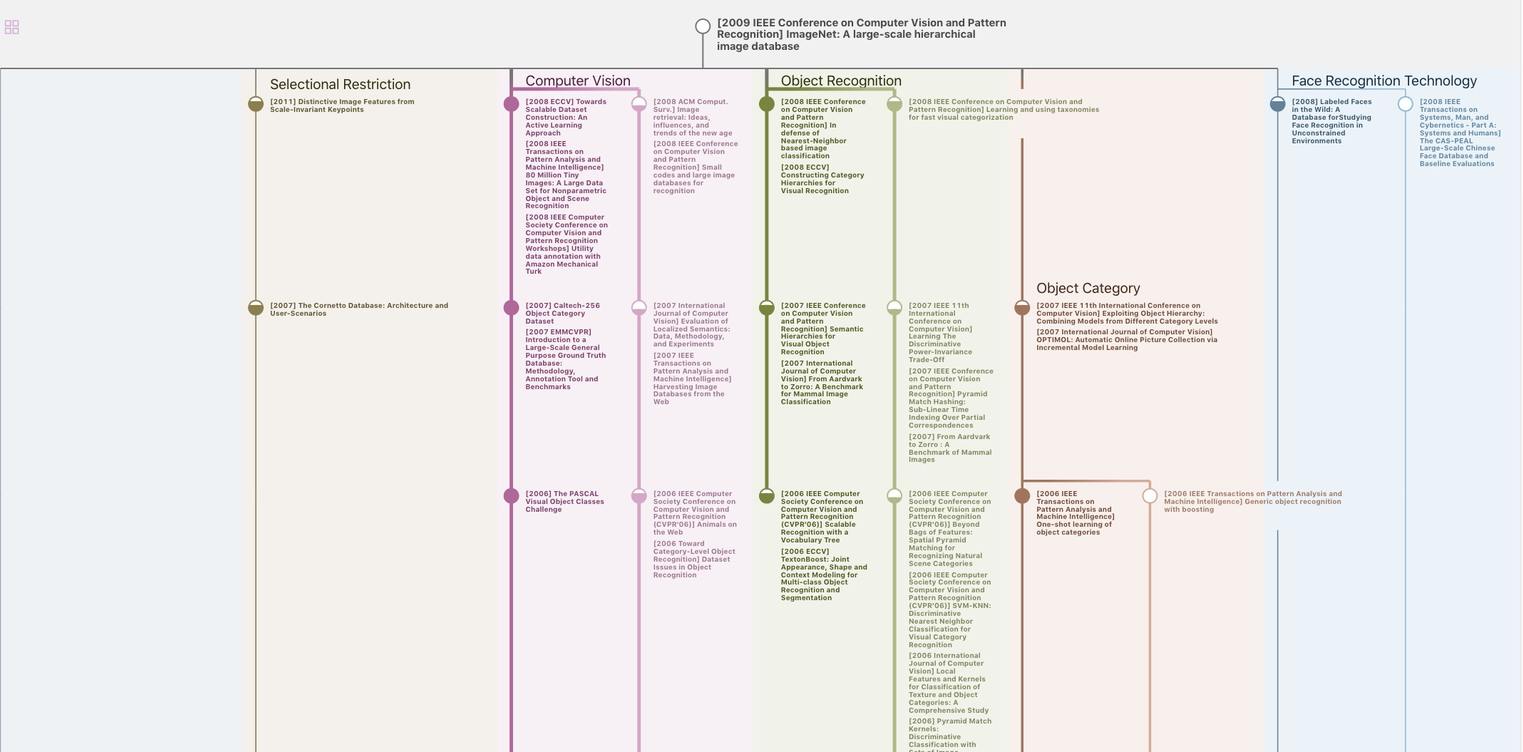
生成溯源树,研究论文发展脉络
Chat Paper
正在生成论文摘要