Prediction of Solar PV Power Using Deep Learning With Correlation-Based Signal Synthesis
IEEE ACCESS(2024)
摘要
Enhancement of the dispatching capacity and grid management efficiency requires knowledge of photovoltaic power generation beforehand. Intrinsically, photovoltaic power generation is highly volatile and irregular, which impedes its prediction accuracy. This paper proposes deep learning-based approaches and a pre-processing algorithm to handle these constraints. The proposed scheme employs Pearson's Correlation Coefficient to find the similarity between atmospheric variables and PV power generation. Based on high PCC values, top atmospheric variables and PV power generated time series data are passed through the Empirical Mode Decomposition (EMD) to simplify the complex data streams into Intrinsic Mode Functions (IMFs). Further, to streamline the prediction process, the proposed correlation-based signal synthesis (CBSS) algorithm finds combinations of these IMFs, which have a high correlation value between atmospheric variables and PV power data. Deep learning models of algorithms Long Short Term Memory (LSTM) and Nonlinear Autoregressive Network with Exogenous Inputs (NARX) network with the configurations of three networks, a single network, and the direct approach employed for the prediction of IMFs combinations. The LSTM network was analyzed under the Adaptive moment estimation (ADAM), Stochastic Gradient Descent with Momentum (SGDM), and Root Mean Square Propagation (RMSP) optimization. Extensive experimentation was evaluated using atmospheric data from the Climate, Energy, and Water Research Institute (CEWRI), NARC, Islamabad, Pakistan. RMSE, MAE, MAPE, and $R<^>{2}$ performance measures show promising prediction results for the LSTM under the configuration of three networks and ADAM optimization.
更多查看译文
关键词
Photovoltaic power prediction,Pearson correlation coefficient,empirical mode decomposition,correlation-based signal synthesis,long short-term memory,nonlinear autoregressive exogenous
AI 理解论文
溯源树
样例
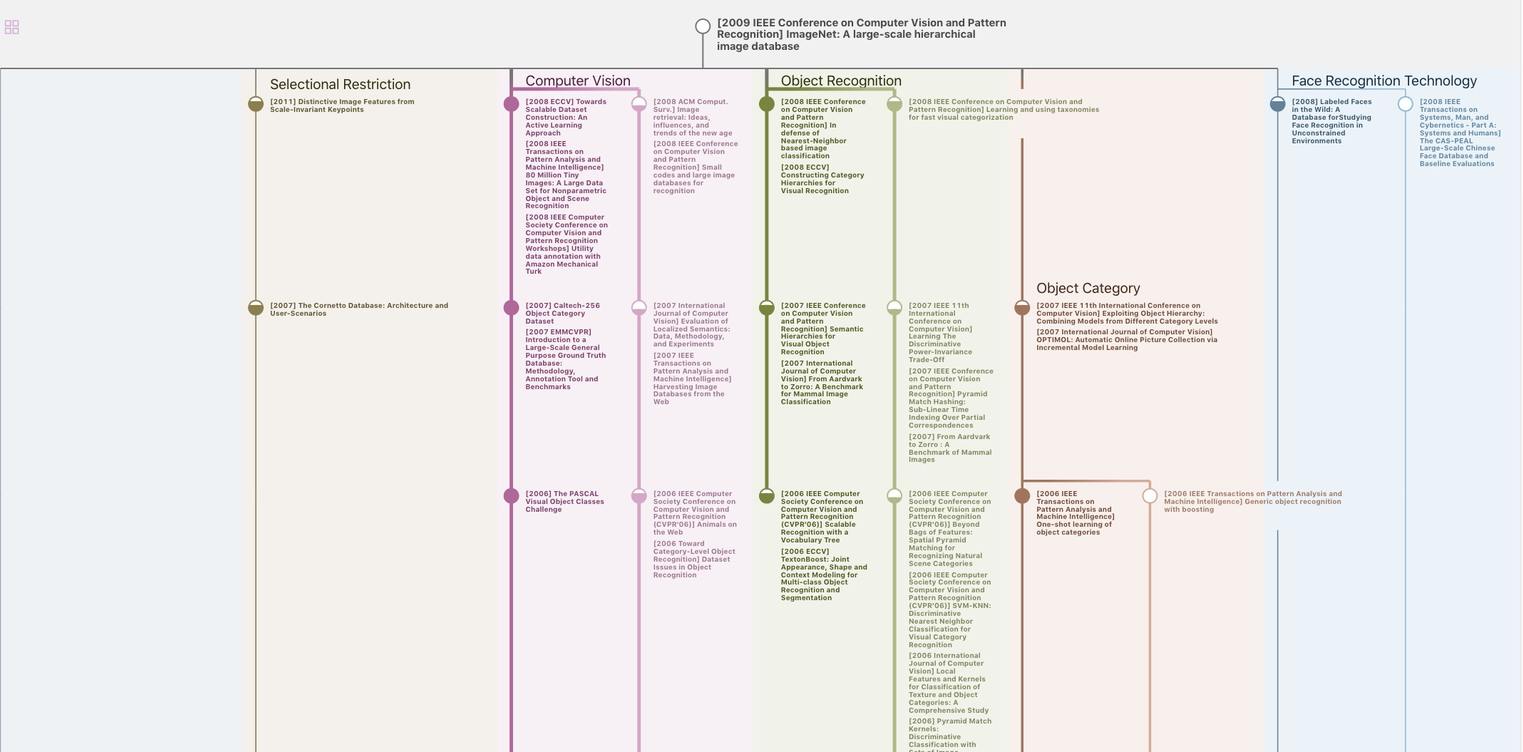
生成溯源树,研究论文发展脉络
Chat Paper
正在生成论文摘要