Twofold Sparsity: Joint Bit- and Network-Level Sparsity for Energy-Efficient Deep Neural Network Using RRAM Based Compute-In-Memory
IEEE ACCESS(2024)
摘要
On-device intelligence and AI-powered edge devices require compressed deep learning algorithm and energy efficient hardware. Compute-in-memory (CIM) architecture is a more suitable candidate than traditional Complementary Metal-Oxide-Semiconductor (CMOS) technology for deep learning applications since computations are performed directly within the memory itself, reducing the need for data movement between memory and processing units. However, the current deep learning compression techniques are not designed to take advantage of CIM architecture. In this work, we proposed Twofold Sparsity, a joint bit- and network-level sparsity method to highly sparsify the deep leaning models by taking advantage of CIM architecture for energy-efficient computations. Twofold Sparsity method sparsify the network during training by adding two regularizations, one to sparsify the weights using Linear Feedback Shift Register (LFSR) mask, and the other one to sparsify the values in the bit-level by making bits zero. During inference, the same LFSRs is used to choose the correct sparsed weights for multiplication between input and weights and 2bit/cell RRAM based CIM is responsible to do the computation. Twofold Sparsity method achieved 1.3x to 4.35x energy efficiency in different sparsity rates compared to baselines and eventually enabling powerful deep learning models to be run on power constrained edge devices.
更多查看译文
关键词
Deep learning,In-memory computing,Training,Energy efficiency,Common Information Model (computing),System-on-chip,Semiconductor device modeling,Edge computing,Artificial intelligence,Compressed sensing,Computing-in-memory,deep learning compression,edge computing,quantization,sparsity
AI 理解论文
溯源树
样例
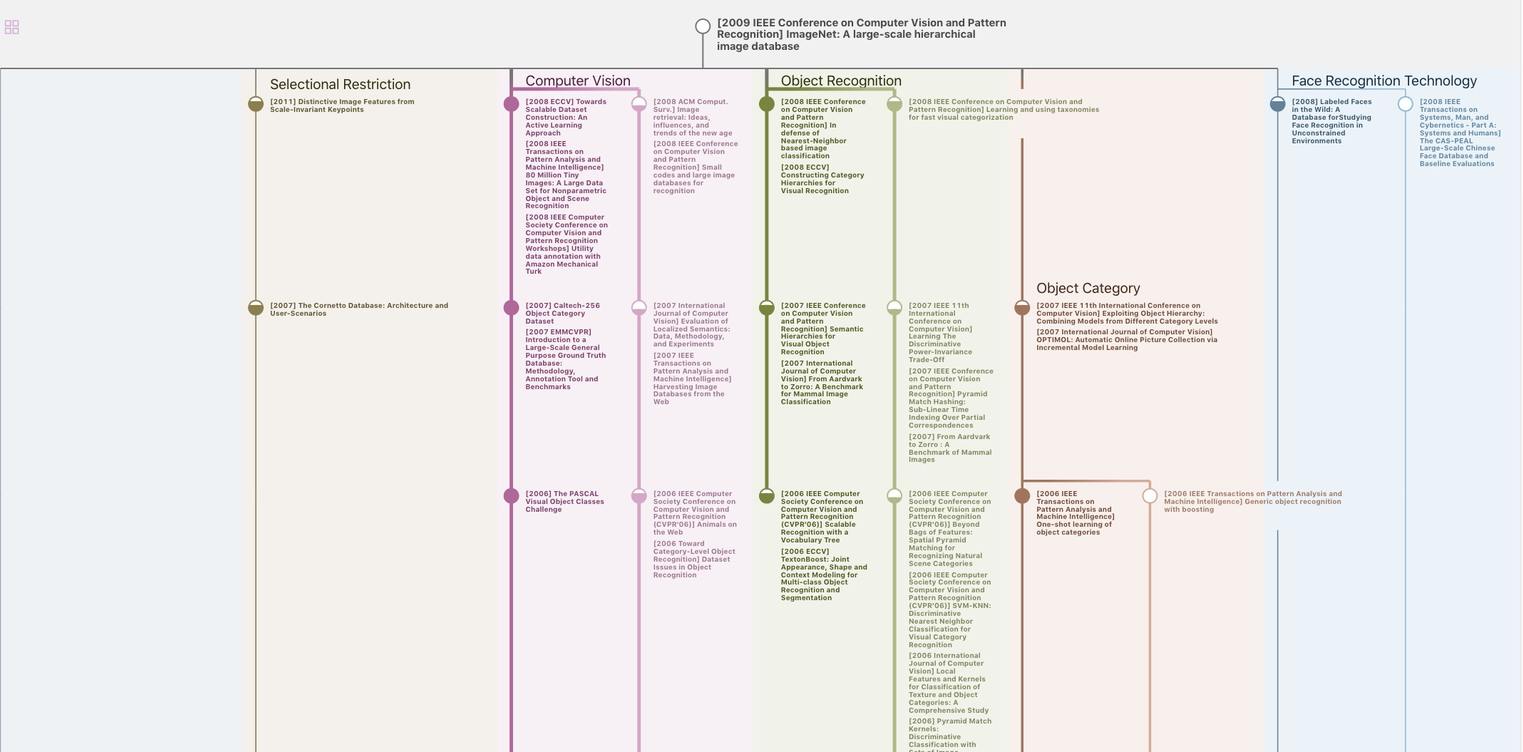
生成溯源树,研究论文发展脉络
Chat Paper
正在生成论文摘要