Self-Supervised Underwater Image Generation for Underwater Domain Pre-Training
IEEE TRANSACTIONS ON INSTRUMENTATION AND MEASUREMENT(2024)
摘要
The rapid progress in computer vision has presented new opportunities for enhancing the visual capabilities of underwater robots. However, most deep learning-based visual perception algorithms often underperform due to the scarcity of underwater datasets. To address this issue, we propose an underwater image synthesis method for pre-training in the underwater domain. By leveraging self-supervised learning, we simulate the physical imaging process of underwater scenes, allowing for style transfer from in-air images to underwater images using a reduced amount of underwater data. Furthermore, we propose a pre-training strategy that utilizes synthetic underwater images to enhance underwater visual perception. Finally, abundant experiments are conducted, including quantitative and qualitative comparisons. The results validate the effectiveness and superiority of the proposed underwater image synthesis method, highlighting the substantial improvement in underwater environment perception achieved through the underwater domain pre-training (UDP) strategy.
更多查看译文
关键词
Object detection,pre-training,self-supervised learning,semantic segmentation,underwater image generation
AI 理解论文
溯源树
样例
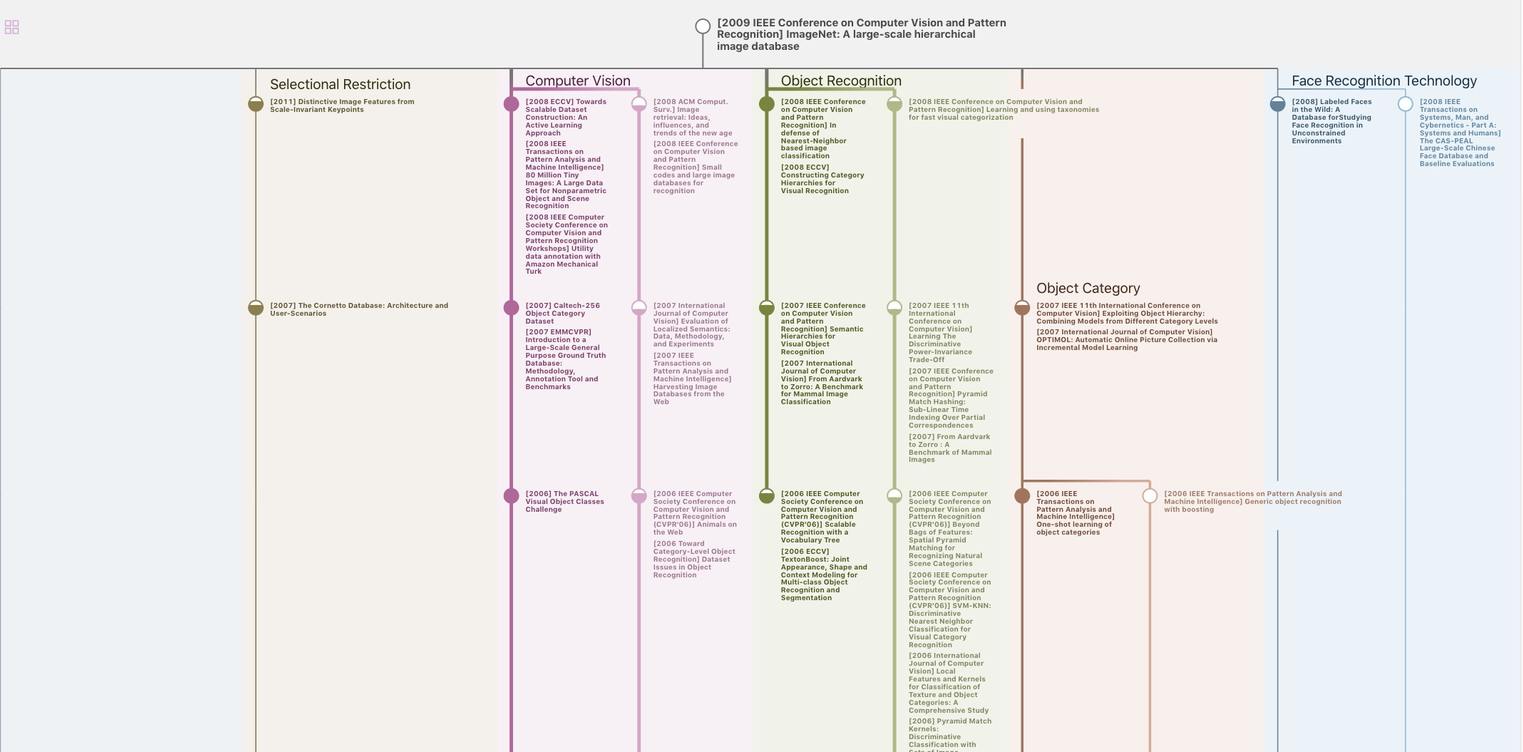
生成溯源树,研究论文发展脉络
Chat Paper
正在生成论文摘要