Data-Driven Electromagnetic Susceptibility Modeling Method for Analog Sensors
IEEE Sensors Journal(2024)
摘要
Analog sensors that operate in weak signal systems are susceptible to external complex electromagnetic environment, which is typically reflected in their output offsets. Electromagnetic susceptibility (EMS) modeling of sensors can evaluate their operational status and predict their output offsets with monitored electromagnetic interference (EMI) signal parameters. Forward EMS modeling for a specific sensor entails multiple factors and exhibits limited generalizability. To mitigate this problem, a data-driven EMS modeling method for analog sensors based on limited EMS test data is proposed in this article, which extracts correlation characteristics between the sensor output offset and EMI signal parameters through Gaussian process regression (GPR). Two EMI signal parameters, namely, interfering frequency and interfering level, are utilized as training inputs, and the corresponding sensor output offset obtained from EMS tests are employed as training targets. The proposed method assumes that the acquired test data inherently contain noise, aligning with the inherent uncertainty of EMS tests. This serves to enhance its generalization capability. Two types of EMS tests, conduction susceptibility and radiation susceptibility tests, are carried out on two types of sensors to demonstrate the effectiveness and superiority of this method. Experimental results indicate that this method has the superior prediction accuracy than three other commonly used regression methods. Additionally, it has the capability to estimate the confidence of the predicted results and reduce the required testing workload through the Grubbs’ test. On this basis, the operational status of sensors can be evaluated and the sensing output can be modified appropriately.
更多查看译文
关键词
Electromagnetic susceptibility (EMS) modeling,electromagnetic interference (EMI),Gaussian process regression (GPR),machine learning
AI 理解论文
溯源树
样例
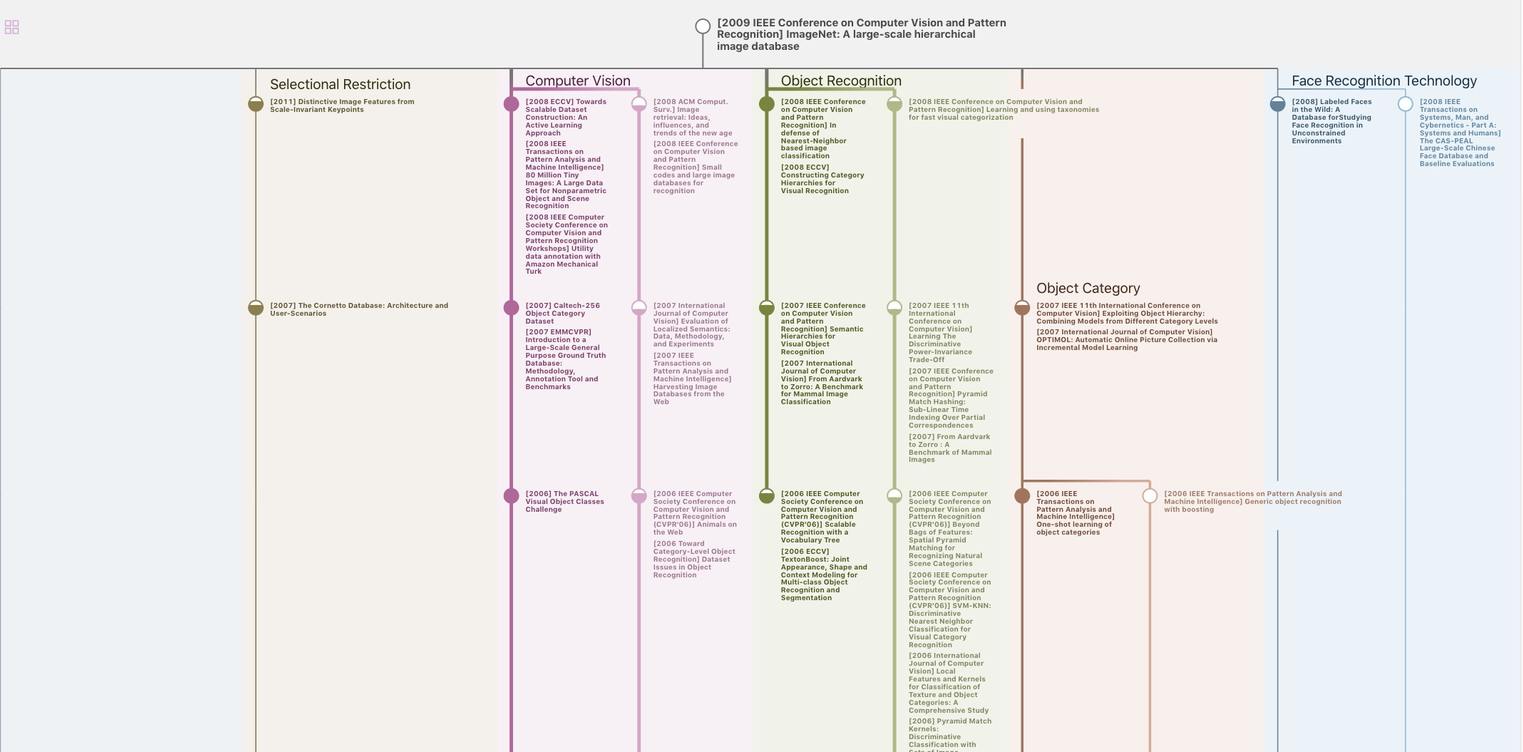
生成溯源树,研究论文发展脉络
Chat Paper
正在生成论文摘要