TUT: Template-Augmented U-Net Transformer for Unsupervised Anomaly Detection
IEEE SIGNAL PROCESSING LETTERS(2024)
摘要
In anomaly detection, acquiring a sufficient number and diverse range of anomaly samples is challenging due to their scarcity and unpredictability. To address this issue, this paper focuses on exploring an unsupervised method that can fit the distribution of positive samples during training. Previous methods adopt a reconstruction paradigm to detect anomaly positions based on significant reconstruction discrepancies since rebuilding normal inputs often has minor errors. However, since the reconstruction is an unsupervised process, most vanilla generative models can also reconstruct anomaly samples with minor reconstruction errors, which has a negative effect on detecting anomaly samples. To address this problem, we propose a new U-net pipeline that integrates template-augmented Transformer to enlarge reconstruction error for anomaly samples, while still keeping the error for normal samples small. Additionally, we employ dense contrastive learning in the pre-training phase to help the encoder extract separable representations of normal and anomaly samples. Extensive experiments on four benchmark datasets demonstrate our model robustness and effectiveness.
更多查看译文
关键词
Anomaly detection,U-net,transformer,unsupervised learning
AI 理解论文
溯源树
样例
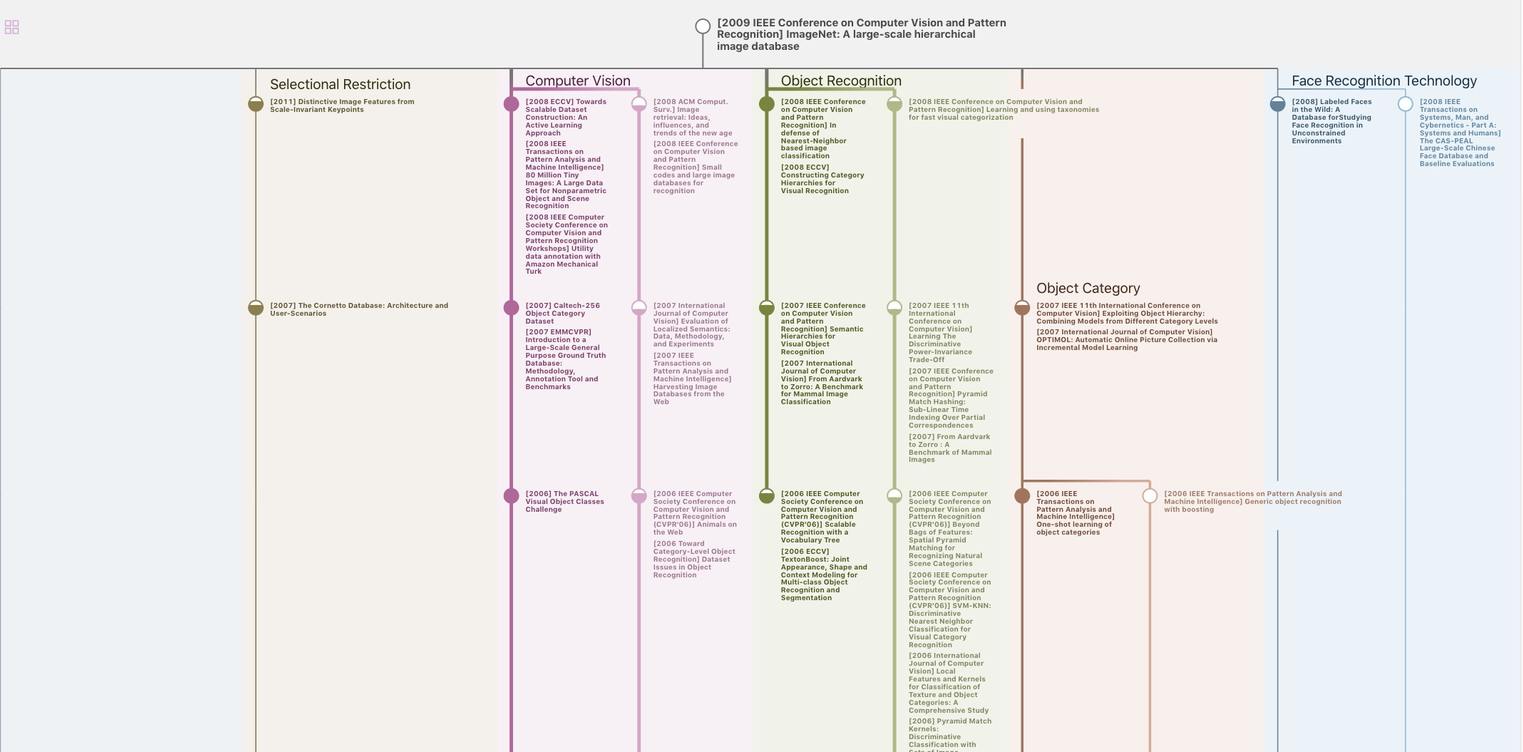
生成溯源树,研究论文发展脉络
Chat Paper
正在生成论文摘要