Optimal design of composite grid/skin structures based on deep learning and Double-Double layup strategy
Aerospace Science and Technology(2024)
摘要
This study introduces a novel two-stage optimization framework combining deep learning and genetic algorithms for the design of composite grid/skin structures. Firstly, an image dataset of 131,022 potential design configurations based on the Double-Double (DD) layup strategy is created. Subsequently, a deep-learning based Auto-encoder model is employed to extract the structural genes from this dataset. These structure genes are then used to establish a surrogate model using transfer learning, which yields accurate predictions of the buckling loads for various grid/skin structures. Finally, the Genetic Algorithm (GA) is employed to explore the optimal design configuration based on the surrogate model, with the objective of maximizing the buckling load while minimizing the weight. Results show that the optimized structure surpasses the traditional Ortho-grid and Iso-grid designs in terms of buckling load by 145% and 72%, respectively. Additionally, it improves the structure efficiency by 31% and 56%, respectively. These findings emphasize the effectiveness of the two-stage optimization method in addressing complex, multi-objective challenges, offering valuable insights and promising prospects for the optimization design of composite grid/skin structures.
更多查看译文
关键词
Optimal Design,Composite Grid/skin Structures,Deep Learning,Double-Double layup strategy, Buckling Analysis
AI 理解论文
溯源树
样例
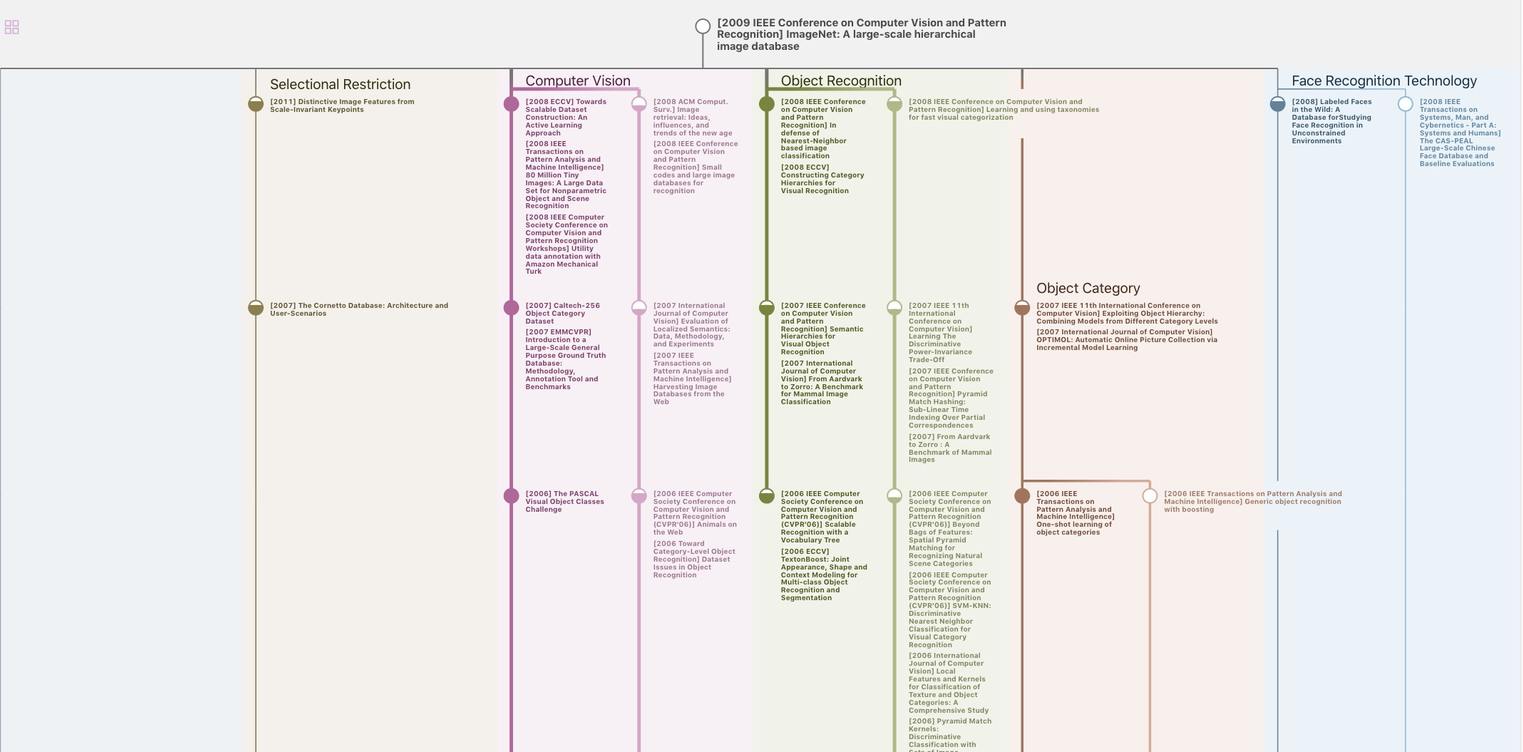
生成溯源树,研究论文发展脉络
Chat Paper
正在生成论文摘要