HPNet: High precision point cloud registration using feature pyramid and hybrid position encoding
COMPUTERS & GRAPHICS-UK(2024)
摘要
Point cloud registration precision degrades when the scene contains symmetric structures or repeated patches. Also, the common operation of continuous downsampling in existing registration methods causes the loss of detailed information and leads to further registration precision degradation. In this paper, we propose a high precision point cloud registration network (HPNet) to learn discriminative features by considering both multi -scale information and position information. We first propose a multi -scale feature extraction module similar to the feature pyramid that allows the extracted features to contain multi -scale information. Then, an information interaction is performed on the features to learn global contextual information by using Transformer with a hybrid position encoding, which takes into account both absolute and relative positions of points. Finally, the features obtained from the information interaction module are directly used to predict the point correspondences. Comprehensive experiments on 3DMatch, 3DLoMatch, ModelNet, and ModelLoNet datasets demonstrate the state-of-the-art performance of the proposed method and the implementation of HPNet in SLAM shows its effectiveness in real application.
更多查看译文
关键词
Point cloud registration,Hybrid position encoding,Deep learning
AI 理解论文
溯源树
样例
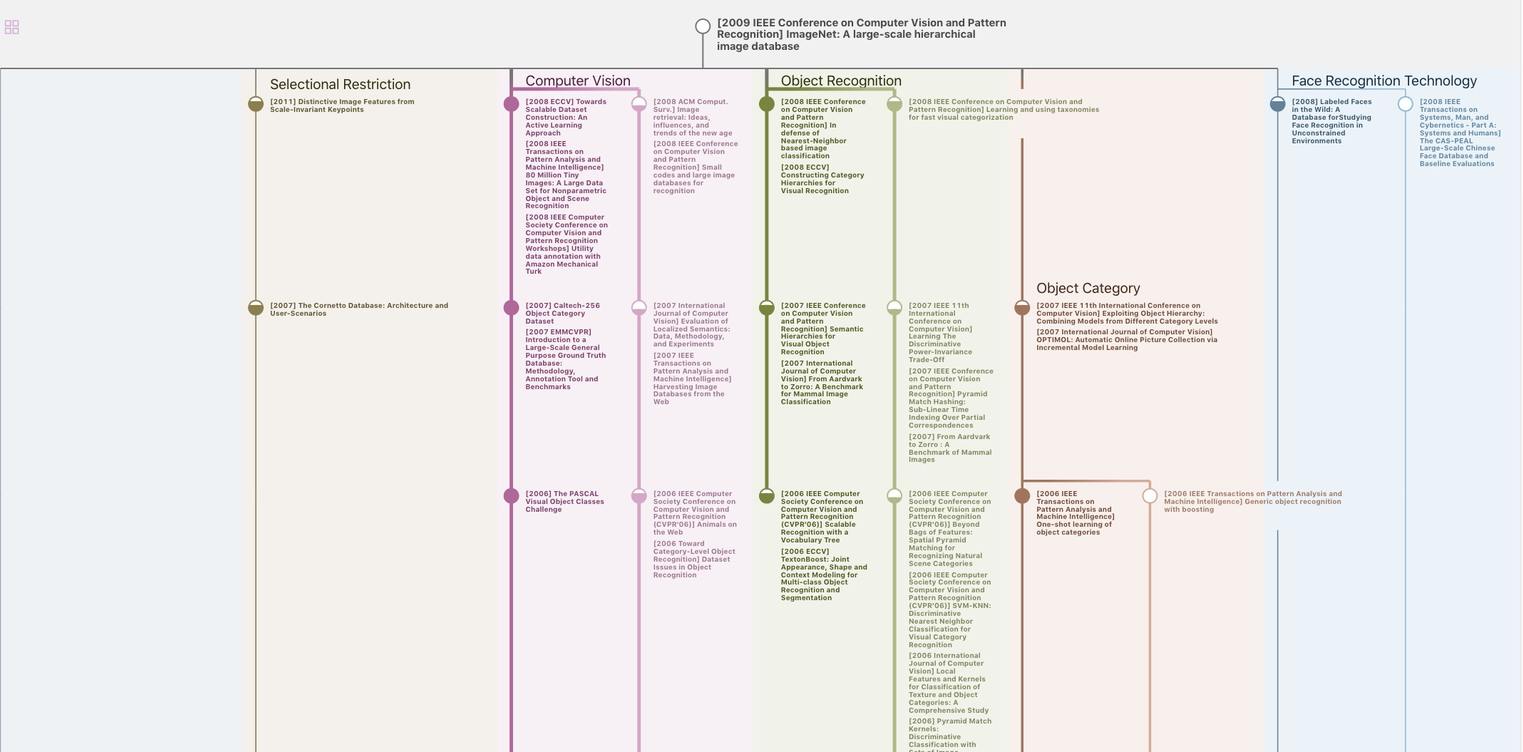
生成溯源树,研究论文发展脉络
Chat Paper
正在生成论文摘要