High-speed encrypted traffic classification by using payload features
Digital Communications and Networks(2024)
摘要
Traffic encryption techniques facilitate cyberattackers to hide their presence and activities. Traffic classification is an important method to prevent network threats. However, due to the tremendous traffic volume and limitations of computing, most existing traffic classification techniques are inapplicable to the high-speed network environment. In this paper, we propose a High-speed Encrypted Traffic Classification (HETC) method containing two stages. First, to efficiently detect whether traffic is encrypted, HETC focuses on randomly sampled short flows and extracts aggregation entropies with chi-square test features to measure the different patterns of the byte composition and distribution between encrypted and unencrypted flows. Second, HETC introduces binary features upon the previous features and performs fine-grained traffic classification by combining these payload features with a Random Forest model. The experimental results show that HETC can achieve a 94% F-measure in detecting encrypted flows and a 85%–93% F-measure in classifying fine-grained flows for a 1-KB flow-length dataset, outperforming the state-of-the-art comparison methods. Meanwhile, HETC does not need to wait for the end of the flow and can extract mass computing features. The average time for HETC to process each flow is only 2 or 16 ms, which is lower than the flow duration in most cases, making it a good candidate for high-speed traffic classification.
更多查看译文
关键词
Traffic classification,Flow analysis,Information entropy,Machine learning,Randomness test
AI 理解论文
溯源树
样例
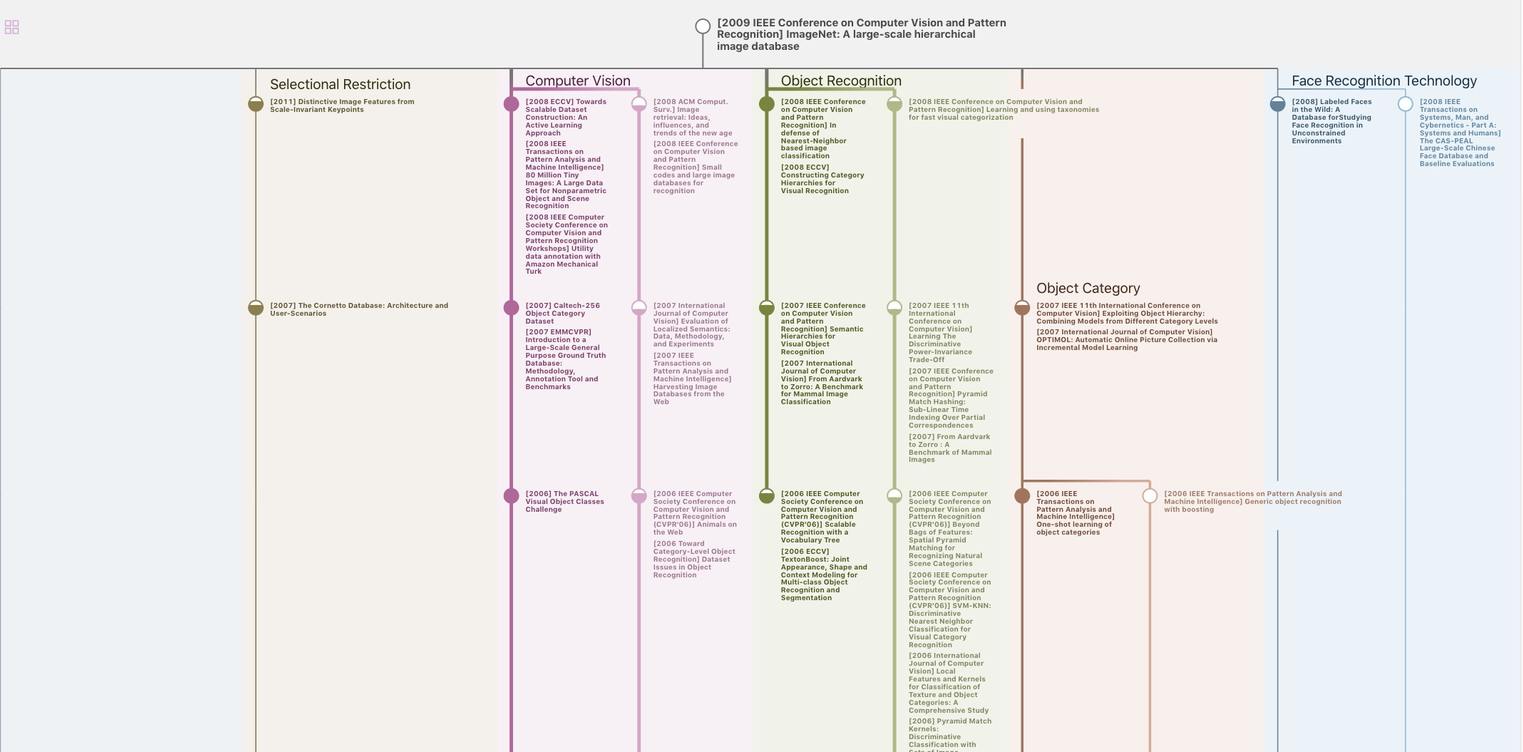
生成溯源树,研究论文发展脉络
Chat Paper
正在生成论文摘要