An arithmetic and geometric mean-based multi-objective moth-flame optimization algorithm
Cluster Computing(2024)
摘要
Expanding the capacity of optimization algorithms for simultaneous optimization of multiple competing objectives is a crucial aspect of research. This study presents MnMOMFO, a novel non-dominated sorting (NDS) and crowding distance (CD)-based multi-objective variant of the moth-flame optimization (MFO) algorithm for multi-objective optimization problems. The algorithm incorporates arithmetic and geometric mean concepts to address MFO’s limitations and to improve its performance. Subsequently, we extend this enhanced MFO into a multi-objective variant, leveraging NDS and CD strategies to achieve a well-distributed Pareto optimal front. The effectiveness of the proposed MnMOMFO algorithm is rigorously evaluated across three distinct phases. In the initial phase, we scrutinize its performance on four ZDT multi-objective optimization problems, employing four performance metrics—general distance, inverted general distance, spacing, and spread metric. Comparative analyses with select competitive multi-objective optimization algorithms comprehensively understand MnMOMFO’s efficacy. Secondly, 24 complex multi-objective IEEE CEC 2020 test suits are considered on two performance metrics. Namely, Pareto sets proximity and the inverted generational distance in decision space. In the third phase, five real-world engineering problems are considered to measure the problem-solving ability of the MnMOMFO algorithm. The results from the experiments indicated that the MnMOMFO was the best candidate algorithm, achieving more than 95
更多查看译文
关键词
Moth-flame optimization algorithm,Multi-objective algorithm,Non-dominated sorting,Crowding distance,IEEE CEC 2020 test functions
AI 理解论文
溯源树
样例
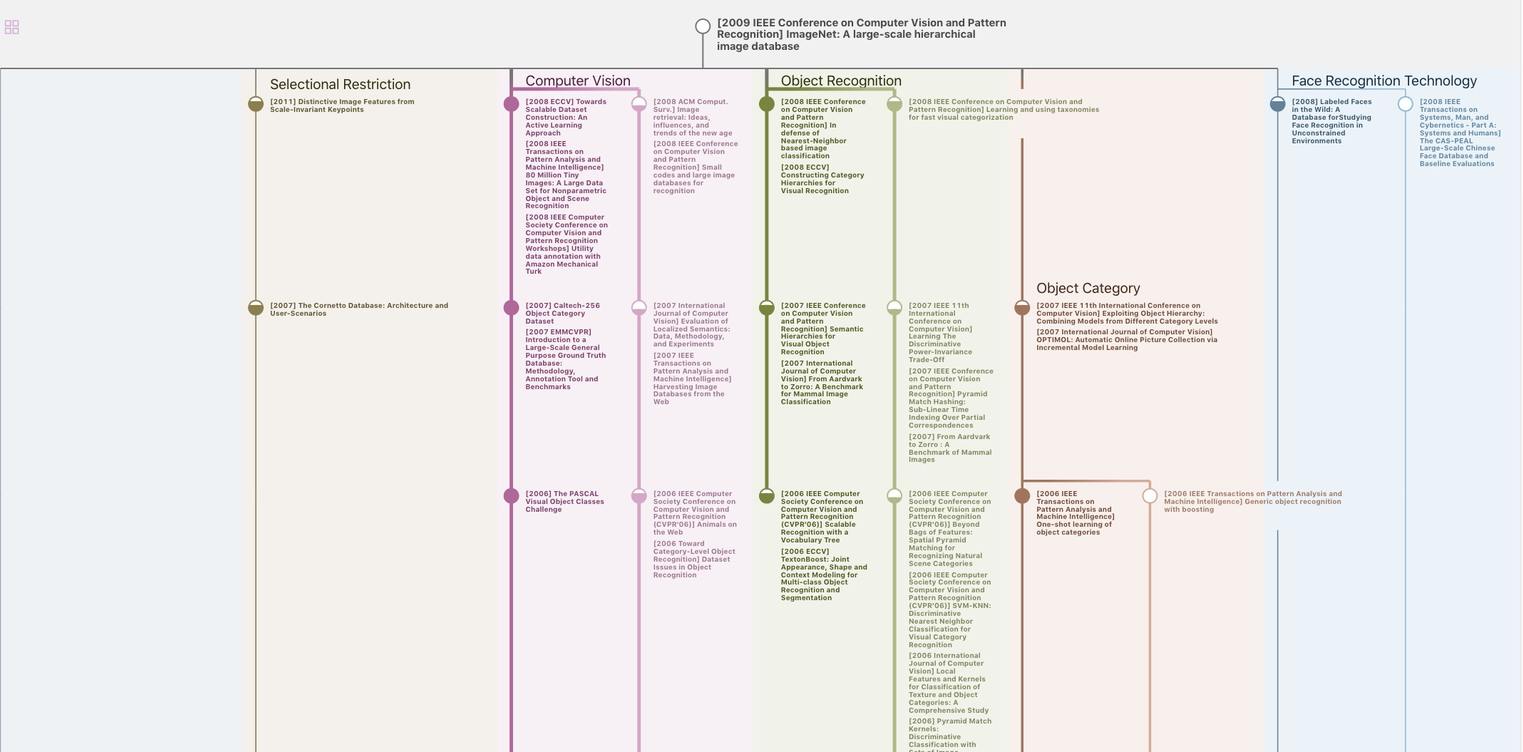
生成溯源树,研究论文发展脉络
Chat Paper
正在生成论文摘要